10 Must-Read Business Strategy Posts. Data Lineage @ Netflix. ML Infra @ Stripe. [DSR #188]
Quick note! This summer, from June through August, I’m going to slow down just a bit, publishing once every two weeks instead of once a week. I’m looking forward to enjoying my summer Saturdays and sitting at the keyboard just a bit less! I’ll be back to once a week starting in September.
Enjoy the issue :)
- Tristan
–
❤️ Want to support this project? Forward this email to three friends!
🚀 Forwarded this from a friend? Sign up to the Data Science Roundup here.
This week's best data science articles
10 Reads for Data Scientists Getting Started with Business Models
If you’re getting started with data science, you’re probably focusing your attention on mostly stats and coding. There’s nothing wrong with this, in fact, this is the right move — these are essential skills that you need to develop early on in your journey.
With this being said, the biggest knowledge gap that I’ve encountered during my data science journey doesn’t deal with either of these areas. Instead, upon starting my first full-time role as a data scientist, I realized, to my surprise, that I didn’t really understand business.
The links in the article are phenomenal. This is the single best business-related reading list I’ve ever found.
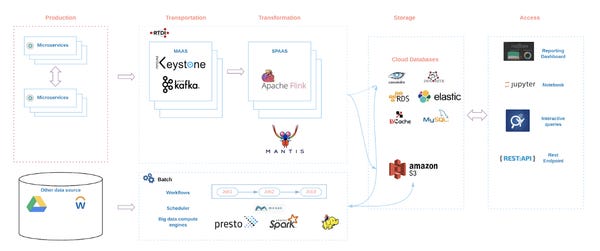
Imagine yourself in the role of a data-inspired decision maker staring at a metric on a dashboard about to make a critical business decision but pausing to ask a question — “Can I run a check myself to understand what data is behind this metric?”
Now, imagine yourself in the role of a software engineer responsible for a micro-service which publishes data consumed by few critical customer facing services (e.g. billing). You are about to make structural changes to the data and want to know who and what downstream to your service will be impacted.
The two scenarios described above are huge problems in at-scale data-driven companies, and they’re both problems of data lineage. Data lineage—the ability to understand where data comes from and goes to—is an extremely hot area at the moment, and it’s a hard problem when your infrastructure looks like Netflix’s does (the image above). Lyft just published about their internal Amundsen lineage tool and there was quite a buzz around it at last month’s DataCouncil conference. The importance of this problem is why we built dbt Docs late last year, and it’s why we’re very focused on going deeper on this area.
Anyway, this is a fascinating topic that is currently playing out in real-time, and this post outlines Netflix’s current approach.
Stripe: How We Rapidly Train Machine Learning Models with Kubernetes
This post is…intense. It’s one of the deepest “behind-the-scenes here’s how our ML infrastructure works” posts, and as such it’s quite notable. The infrastructure described in this post is impressive, and far beyond what most teams have access to.
I recently spent a bunch of time with a good friend who works in data, and we spent a lot of time talking about the pluses and minuses of different jobs in the field. It really made me recognize just how important tooling is as a part of the vetting process for a new job in data: employees at companies with advanced tooling are just far more effective than employees at companies with no or poor tooling. That means they do more valuable work, get better experience, and level up faster. They also just tend to be happier.
In your next interview process, make sure you learn about the team you’ll be working on and the tooling you’ll have access to. Weight that heavily in your decision criteria.
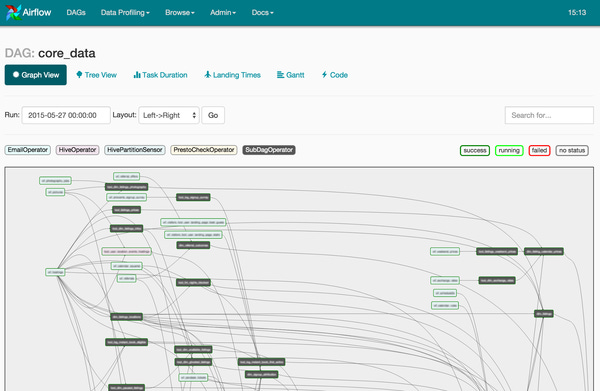
Large-Scale Data-Driven Initiatives at Airbnb
This is a short but poignant overview of Airbnb’s data efforts. It includes a nice overview of why Airbnb invests so heavily in data (very in-line with the business strategy post from above) and then provides some highlights and useful links to explore some of Airbnb’s data efforts.
In the spirit of software design patterns, here are some examples of design patterns for academic research, especially in engineering and technology-related fields.
I love this! There is plenty of advice published around how to ask good questions, but these are actually design patterns—templates to apply to your thinking—that can help guide you. The post is aimed at academic research, but there are lots of useful insights for data scientists & analysts.
Build Your Career in Data Science
This book—not yet released!—could be quite relevant if you’re just getting into the field. It’s not about technical skills, it’s actually about everything else. There’s a lot in this table of contents that is under-covered on the internet at large (for example “how to work with stakeholders”!). Could be a great investment.
Thanks to our sponsors!
Fishtown Analytics: Analytics Consulting for Startups
At Fishtown Analytics, we work with venture-funded startups to build analytics teams. Whether you’re looking to get analytics off the ground after your Series A or need support scaling, let’s chat.
www.fishtownanalytics.com • Share
Stitch: Simple, Powerful ETL Built for Developers
Developers shouldn’t have to write ETL scripts. Consolidate your data in minutes. No API maintenance, scripting, cron jobs, or JSON wrangling required.
The internet's most useful data science articles. Curated with ❤️ by Tristan Handy.
If you don't want these updates anymore, please unsubscribe here.
If you were forwarded this newsletter and you like it, you can subscribe here.
Powered by Revue
915 Spring Garden St., Suite 500, Philadelphia, PA 19123