12 Ways to F*** up your A/B Test. Open Data. Visualizing Time and Space. [DSR #105]
A week ago I was unfamiliar with the term isochrone—maybe you were too? My gift to you in this week’s data viz :)
Enjoy!
- Tristan
❤️ Want to support us? Forward this email to three friends!
🚀 Forwarded this from a friend? Sign up to the Data Science Roundup here.
Required Reading
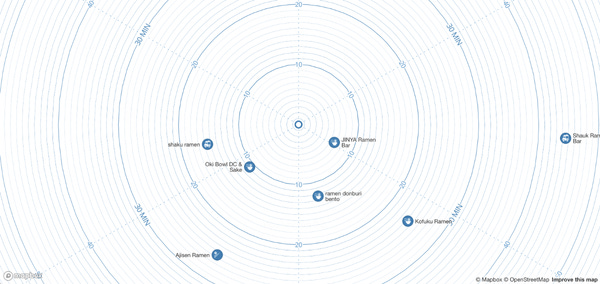
A New Kind of Map: It’s About Time
Mapbox has built a new kind of map.
Recently, we’ve been thinking of a visualization that cuts directly to the way in which people make decisions about where to go: what would a map look like if we swept the physical world away completely, in favor of the time needed to move around it?
In this time map, we preserve the direction of each point, relative to the user. But the visual distance from that center point is determined by the time it takes to get there, whether driving, biking, or on foot.
This map requires quite a lot of magic to create: geolocation, destination lookup, and mapping via all available modes of transport. Once the data is pulled together, you get a singularly useful way to answer the question “Where should we go to lunch?”
There is a gif version of the entire feature towards the bottom of the article that I highly recommend checking out.
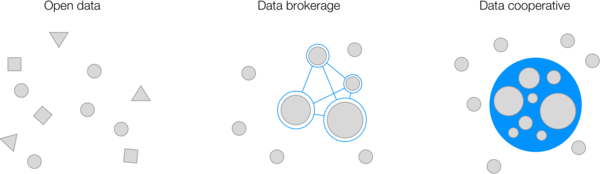
Data Liquidity in the Age of Inference
This article tackles one of the most important issues in data today. Most large datasets currently in existence are owned by large platforms who hoard that data as a core competitive advantage:
If a company has invested in building valuable data sets that help differentiate its product or service, its motivation is to preserve that moat by protecting that data against competition.
Google, Facebook, Amazon. Tesla with AutoPilot. Etc.
The author introduces three different structures in which data can be shared broadly: open data, data brokerages, and data cooperatives. Each provides a different take on the problem of getting more data into more hands.
Before the internet, almost all software code was closed source, where today open source software powers everything around us. Thinking as the Data-ists of Yuval Harari’s Homo Deus—information wants to be free—will we begin to witness a rise of collaborative data repositories?
This is an important topic, and one that doesn’t get enough attention. This post goes deeper than anything I’ve seen.
This Week's Top Posts
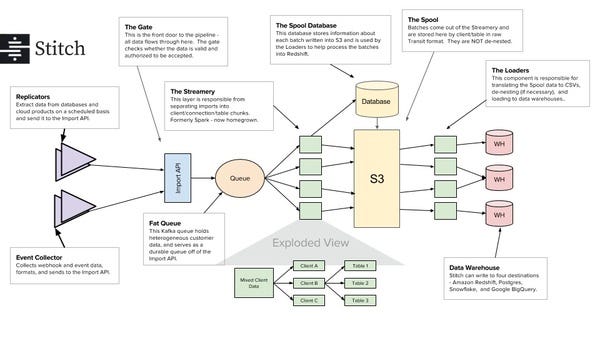
How Stitch Consolidates A Billion Records Per Day
The tech behind Stitch. This is how your data gets to your warehouse. Quite detailed, worth the read both as a reference architecture and if you’re a current/prospective customer.
Twelve Common Metric Interpretation Pitfalls in Online Controlled Experiments
This paper by Microsoft Research is the single best resource on online experimentation I’ve read in the past year. It goes through a dozen common ways that the authors have seen A/B test metrics get misinterpreted. Each one comes with a concrete example.
Long. Worthwhile.
Lynn Langit on big data, NoSQL, and Google versus AWS
ETL is still the big, bad problem in the world of data. I would like to see more ETL tools that include machine learning and statistics. “It looks like this data needs X.” “This schema is A and this schema is B. It looks like you need transformations of ABC.”
Solid interview on the cloud ecosystem. Lots of topics. Very agree.
AI is the goal for many enterprises. But, an organization needs machine learning, in order to do AI. And, machine learning is not possible without analytics. And analytics is not possible without simple, elegant data infrastructure. Simply put, there is no AI without IA (information architecture).
Quick read, excellent perspective.
New York City: Data Science’s Best Bet for Growth and Opportunity
One entrepreneur’s perspective on why NYC is the perfect environment for data science. Useful if you’re transitioning to the field and considering a move.
blog.insightdatascience.com • Share
Why I don’t like Jupyter Notebooks
While I’m a fan of Jupyter, this author makes some totally valid points. His recommendations for notebooks:
Make them deterministic + reproducible.
Store the notebook as human readable plain text.
Abandon the idea of using HTTP/TCP/IP to access them.
owainkenwayucl.github.io • Share
Data viz of the week
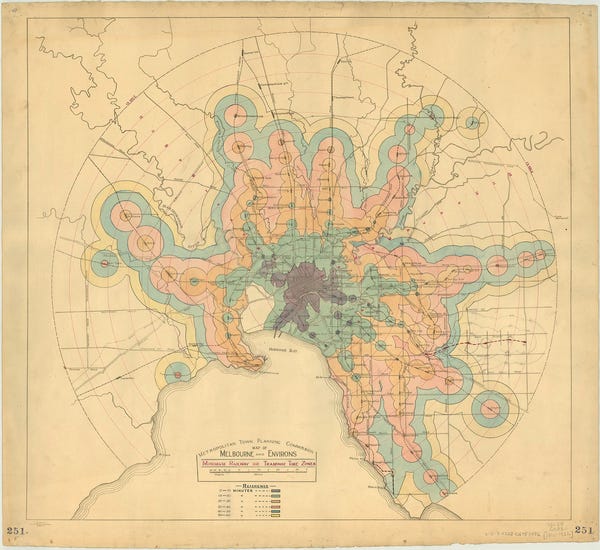
An isochrone from the 1920's illustrating travel times by train.
Thanks to our sponsors!
Fishtown Analytics: Analytics Consulting for Startups
At Fishtown Analytics, we work with venture-funded startups to implement Redshift, Snowflake, Mode Analytics, and Looker. Want advanced analytics without needing to hire an entire data team? Let’s chat.
Stitch: Simple, Powerful ETL Built for Developers
Developers shouldn’t have to write ETL scripts. Consolidate your data in minutes. No API maintenance, scripting, cron jobs, or JSON wrangling required.
The internet's most useful data science articles. Curated with ❤️ by Tristan Handy.
If you don't want these updates anymore, please unsubscribe here.
If you were forwarded this newsletter and you like it, you can subscribe here.
Powered by Revue
915 Spring Garden St., Suite 500, Philadelphia, PA 19123