20 Questions. AI and Compute. Reporting is a Gateway Drug. [DSR #136]
❤️ Want to support us? Forward this email to three friends!
🚀 Forwarded this from a friend? Sign up to the Data Science Roundup here.
The Week's Most Useful Posts
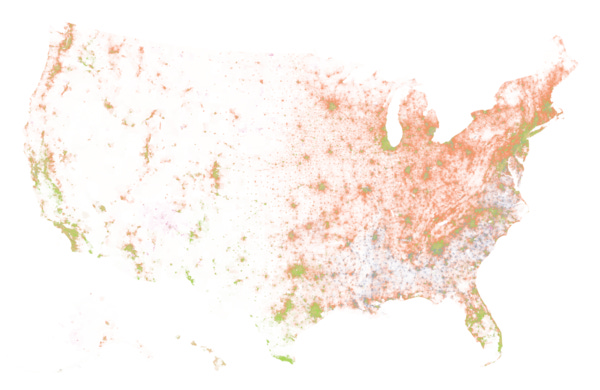
If We All Left to “Go Back Where We Came From”
Everyone comes from somewhere.
This Nathan Yau special is absolutely stunning, but oh-so-simple. You’ve likely seen maps that show racial breakdown of the US. One commonality of every such chart I’ve ever seen has been its low resolution. As we all know, aggregation can hide interesting details in the raw data.
Click through to the source article and look at the detail on the maps. Each dot represents a single person. What do you start to see from the data when it’s no longer aggregated? How do you react to this image differently than this one or this one?
The choices we make when analyzing, presenting, and visualizing data matter quite a lot.
20 Questions to Ask Prior to Starting Data Analysis
This is a topic that doesn’t get a lot of attention: what’s the process for conducting analysis?
Most analytical endeavors, in my experience, fail because analysts didn’t answer all of these questions before getting started. People miss obvious shit. They fail to recognize up front that there is a core data element that is missing. They don’t set data latency expectations with stakeholders. Et cetera.
Analytics is hard. And professionals facing hard problems solve them with checklists. This is as good of a checklist for analytics as I’ve ever seen.
towardsdatascience.com • Share
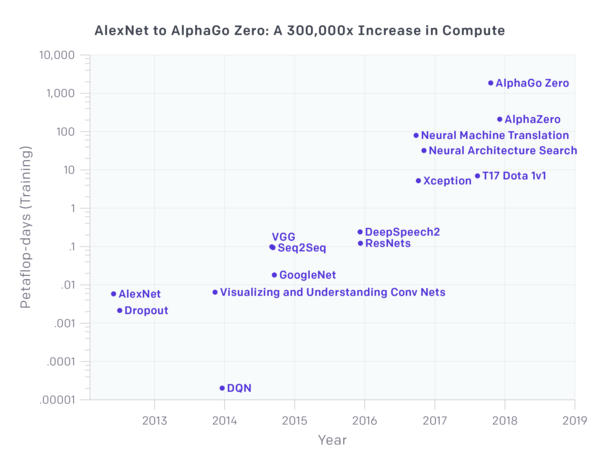
New research from OpenAI is fascinating:
We’re releasing an analysis showing that since 2012, the amount of compute used in the largest AI training runs has been increasing exponentially with a 3.5 month-doubling time (by comparison, Moore’s Law had an 18-month doubling period). Since 2012, this metric has grown by more than 300,000x (an 18-month doubling period would yield only a 12x increase). Improvements in compute have been a key component of AI progress, so as long as this trend continues, it’s worth preparing for the implications of systems far outside today’s capabilities.
The article goes into detail on why there is room left in this trajectory.
So Your Data Science Project Isn’t Working
This is a great post not because it contains some new truth about what goes wrong on data science projects, but rather because it focuses on the emotional experience of failure for the project team. That failure can be quite frustrating and stressful. Here’s the author’s conclusion:
Feeling upset about these things is natural! Over time you will grow as a data scientist. You will get better at understanding the potential risks, but you can never avoid them fully. Take care of yourself and remember that you’re doing the best with what you got.
Not every data science project is fated to succeed—it’s a high-variance field! If you’re on a project that doesn’t work, keep moving forwards.
This post is wonderful advice for anyone starting a new analytics team at an organization. Start by delivering existing reports better.
If executed well, reporting can be the gateway drug, resulting in an organization that is completely addicted to its Analytics team. Here is some advice on how to use reporting as a means to create strong stakeholder relationships in your organization.
Creating strong stakeholder relationships is critical for the success of a data team.
www.locallyoptimistic.com • Share
To Build Truly Intelligent Machines, Teach Them Cause and Effect
Judea Pearl, a pioneering figure in artificial intelligence, argues that AI has been stuck in a decades-long rut. His prescription for progress? Teach machines to understand the question why.
There’s a lot in this post. Judea Pearl is worth taking seriously given his bona fides, but this entire train of thought—using causal reasoning rather than statistical relationships to understand the world—is far outside the norm in the field today. Here’s a choice quote from the interview that illustrates just how out of left field it is:
As much as I look into what’s being done with deep learning, I see they’re all stuck there on the level of associations. Curve fitting. That sounds like sacrilege, to say that all the impressive achievements of deep learning amount to just fitting a curve to data. From the point of view of the mathematical hierarchy, no matter how skillfully you manipulate the data and what you read into the data when you manipulate it, it’s still a curve-fitting exercise, albeit complex and nontrivial.
I kind of love that.
www.quantamagazine.org • Share
Thanks to our sponsors!
Fishtown Analytics: Analytics Consulting for Startups
At Fishtown Analytics, we work with venture-funded startups to implement Redshift, Snowflake, Mode Analytics, and Looker. Want advanced analytics without needing to hire an entire data team? Let’s chat.
Stitch: Simple, Powerful ETL Built for Developers
Developers shouldn’t have to write ETL scripts. Consolidate your data in minutes. No API maintenance, scripting, cron jobs, or JSON wrangling required.
The internet's most useful data science articles. Curated with ❤️ by Tristan Handy.
If you don't want these updates anymore, please unsubscribe here.
If you were forwarded this newsletter and you like it, you can subscribe here.
Powered by Revue
915 Spring Garden St., Suite 500, Philadelphia, PA 19123