Agile Analytics. GDPR. Netflix Interview Questions. 10 Reasons Enterprises Suck at Data. [DSR #137]
❤️ Want to support us? Forward this email to three friends!
🚀 Forwarded this from a friend? Sign up to the Data Science Roundup here.
The Week's Most Useful Posts
How will the GDPR Impact Machine Learning?
Answers to the three most commonly asked questions about maintaining GDPR-compliant machine learning programs.
Coming off a week of privacy policy notices, this is probably the single most important post you need to read. It’s an excellent, and very digestible, summary of what you need to know if you’re currently doing machine learning on top of any personal data.
Agile Analytics, Part 1: The Good Stuff
Really great post that reflects the realities on the ground. The author runs the analytics team @ Harry’s.
Agile software engineering practices have become the standard work management tool for modern software development teams. Are these techniques applicable to analytics, or is the nature of research prohibitively distinct from the nature of engineering? In this post I am going to explore some of the pros of using a scrum-like work management process in analytics.
We use scrum to deliver all of our work at Fishtown Analytics and absolutely wouldn’t do it any other way. If you’re not using scrum on your team, this post is a must-read.
www.locallyoptimistic.com • Share
Netflix Data Science Interview Questions
Vimarsh Karbhari has a small publication, Acing AI, that shares information on the data science interviewing processes of major tech companies. His most recent article focuses on Netflix and covers the required background reading you need to do prior to the interview, as well as 18 interview topic areas that you should be ready to address.
Whether or not you are looking for a new role today, this list is a great barometer of the major knowledge areas that one of the best data science organizations in the world cares about. Could you walk into this interview today?
If you found this article useful, check out similar articles for Microsoft, Linkedin, and more at Acing AI.
Top 20 R Libraries for Data Science in 2018
If you’re an R user, this summary is worth a look. There were a couple of surprises for me—dplyr has really grown a ton in the past year, as has plot.ly—so it might be useful to update your priors on the ecosystem.
Ten Red Flags Signaling Your Analytics Program Will Fail
I often throw shade at bigco efforts in data, but have come to very much respect just how hard their task is. Doing analytics at a F500 company is not (primarily) a technology problem, it’s a coordination problem. When you have 25 (or even 250) people at your company, this coordination / communication problems is tractable. At 25,000, it is exponentially harder.
This article is most useful when read as a piece of anthropology. It could just as easily be titled Top 10 Reasons Why Large Enterprises Suck at Data. As you read it, just absorb just how ineffective many of these efforts are:
…a large organization spent hundreds of millions of dollars and more than two years on a company-wide data-cleansing and data-lake-development initiative. The objective was to have one data meta-model—essentially one source of truth and a common place for data management. The effort was a waste.
That hurts my soul. It’s clearly possible to have a large company that excels at data, but it seems like that competency often needs to be baked in from the beginning, deep into the culture.
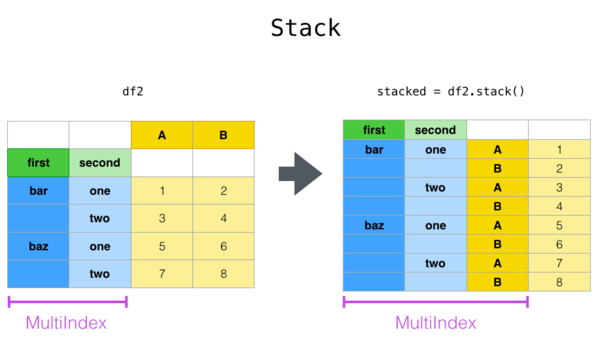
Visualizing Pandas' Pivoting and Reshaping Functions
Learning pandas after learning SQL, many of the data-reshaping functions may seem a bit foreign. This extremely brief, wholly visual explanation is extremely useful / memorable.
My team is currently considering the differences in the roles & titles “data analyst” vs “data scientist” – which vary greatly by industry & org – and trying to define these internally to establish responsibilities & expectations.
What do y’all personally see as the differences?
Following up on the recent Lyft “data analyst vs data scientist” post, this thread on Twitter generated a lot of interesting replies recently. Mikhail is an analyst at the Wikimedia Foundation. Click through for the whole conversation with a lot of smart people.
Road Map for Choosing Between Statistical Modeling and Machine Learning
Here are some rough guidelines that attempt to help researchers choose between the two approaches for a prediction problem.
This is the clearest, most concise post I’ve seen on this important and frequently-screwed-up decision.
Thanks to our sponsors!
Fishtown Analytics: Analytics Consulting for Startups
At Fishtown Analytics, we work with venture-funded startups to implement Redshift, Snowflake, Mode Analytics, and Looker. Want advanced analytics without needing to hire an entire data team? Let’s chat.
Stitch: Simple, Powerful ETL Built for Developers
Developers shouldn’t have to write ETL scripts. Consolidate your data in minutes. No API maintenance, scripting, cron jobs, or JSON wrangling required.
The internet's most useful data science articles. Curated with ❤️ by Tristan Handy.
If you don't want these updates anymore, please unsubscribe here.
If you were forwarded this newsletter and you like it, you can subscribe here.
Powered by Revue
915 Spring Garden St., Suite 500, Philadelphia, PA 19123