AI Index. Parallelization of Model Training. Third Wave Data Viz. XKCD. [DSR #166]
❤️ Want to support us? Forward this email to three friends!
🚀 Forwarded this from a friend? Sign up to the Data Science Roundup here.
This week's best data science articles
Artificial Intelligence Index: 2018 Annual Report
‘Tis the season for “state of the technology” annual reports. As much as it’s a trope, these reports are actually a good way to stay up-to-date on the overall industry as a non-academic practitioner. If you’re like me, you operate in a part of the data science ecosystem and it’s a daunting task to stay up-to-date on the entirety of it. Reports like this are a useful way to do that. Spend one hour with this PDF and you’ll catch up on everything you missed over the past year. This is a particularly good report, with an academic steering committee that includes a who’s who of the field.
The most out-of-left-field stat was about downloads of robot operating system package downloads from ros.org (a site I was previously unfamiliar with):
Since 2014, total downloads and unique downloads have increased by 352% and 567%, respectively. This represents an increased interest in both robotics and the use of robot systems.
The degree of data parallelism significantly affects the speed at which AI capabilities can progress. Faster training makes more powerful models possible and accelerates research through faster iteration times.
In an earlier study, AI and Compute, we observed that the compute being used to train the largest ML models is doubling every 3.5 months, and we noted that this trend is driven by a combination of economics (willingness to spend money on compute) and the algorithmic ability to parallelize training. The latter factor (algorithmic parallelizability) is harder to predict and its limits are not well-understood, but our current results represent a step toward systematizing and quantifying it. In particular, we have evidence that more difficult tasks and more powerful models on the same task will allow for more radical data-parallelism than we have seen to date, providing a key driver for the continued fast exponential growth in training compute.
Elijah Meeks of Netflix shares his historical perspective on where we’ve come and where we’re going in data viz. From Tufte to Grammar of Graphics to the “third wave”. According to Meeks, we’re currently in the “second wave is breaking down” phase and only starting to see elements of the third wave coming into existence. He’s not prescriptive about exactly what defines the third wave, but points the way towards it with some theory and some interesting examples (the examples were fascinating).
I come at this with a very different background but have found myself also believing that we’ve hit a bit of a plateau on visualizations that are producible by non-experts. This article helped me imagine what the world could look like in five years.
towardsdatascience.com • Share
Squarespace: Can Friendly Competition Lead to Better Models?
During Squarespace’s most recent Hack Week, we experimented with a different approach to model building: an internal Kaggle competition. (…) For our internal competition, we wanted to predict subscription rates of customers who start a free trial on Squarespace. The dataset for this competition included anonymized information on customers’ marketing channels, geographic locations, product usage, previous trials, and, of course, whether or not the customers subscribed to Squarespace within 28 days of starting a trial.
This is neat. This isn’t an approach that most companies can take—most of the companies simply don’t have the internal resources that would make this worthwhile, and the author admits that it’s not a particularly efficient way of getting a task done. But if you have an important algorithm problem where the solution would be worth $$$ to the business, this strategy is a worth consideration.
Mark, if you’re reading—you didn’t share the results! How did it go? Are you using any of the models?
engineering.squarespace.com • Share
And…because it was a light week, I couldn’t resist:
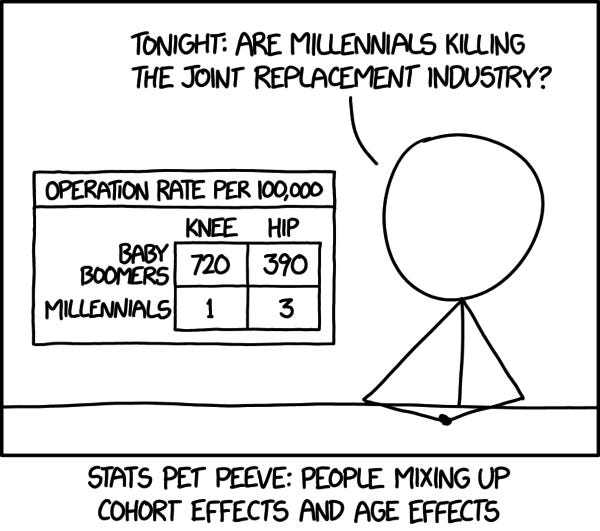
End Note
No roundup next week! I’m off spending time with family for the holidays. See you back on the 30th for the top 10 links of 2018.
- Tristan
Thanks to our sponsors!
Fishtown Analytics: Analytics Consulting for Startups
At Fishtown Analytics, we work with venture-funded startups to build analytics teams. Whether you’re looking to get analytics off the ground after your Series A or need support scaling, let’s chat.
www.fishtownanalytics.com • Share
Stitch: Simple, Powerful ETL Built for Developers
Developers shouldn’t have to write ETL scripts. Consolidate your data in minutes. No API maintenance, scripting, cron jobs, or JSON wrangling required.
The internet's most useful data science articles. Curated with ❤️ by Tristan Handy.
If you don't want these updates anymore, please unsubscribe here.
If you were forwarded this newsletter and you like it, you can subscribe here.
Powered by Revue
915 Spring Garden St., Suite 500, Philadelphia, PA 19123