AI Principles at Google. Academic Data Sharing. 8 Python Concepts You May Have Forgotten. [DSR #139]
❤️ Want to support us? Forward this email to three friends!
🚀 Forwarded this from a friend? Sign up to the Data Science Roundup here.
The Week's Most Useful Posts
Nobody sits around before creating a new Rails project to figure out where they want to put their views; they just run rails new to get a standard project skeleton like everybody else.
The project calls itself “a project template and directory structure for Python data science projects.” It is that, but so much more. My favorite section is the “opinions” section at the end—it includes many great viewpoints as guidance on how to go about your work.
Start using this tomorrow.
8 Python Concepts You May Have Forgotten
Here’s the stuff that I’m always forgetting when working with Python, NumPy, and Pandas.
You know those things that, when you run into them, you always find yourself copying and pasting from that same Stack Overflow post? Yeah…we all do that. This post is all about pausing and solidifying a few concepts so that you’ll avoid that next Stack Overflow search.
towardsdatascience.com • Share
Attacks Against Machine Learning: An Overview
This blog post surveys the attacks techniques that target AI (Artificial Intelligence) systems and how to protect against them.
We’ve discussed ML attack vectors previously, but this is the best post I’ve seen on rounding up all known categories of attacks and explaining each. Great intro to the topic; contains many fascinating examples of attacks.
We’re announcing seven principles to guide our work in AI.
AI ethics is a hot topic, particularly at Google, where thousands of engineers have protested Google’s work in military applications. The company recently released principles that will guide its work in AI.
The principles are, on one level, certainly a work of internal and external PR given the recent dust-up. But it’s also hard to walk back from such a public statement: these principles will likely have some teeth at least for a while. The biggest potential upside of this publication would be if it exerted public pressure on other large technology companies to do the same.
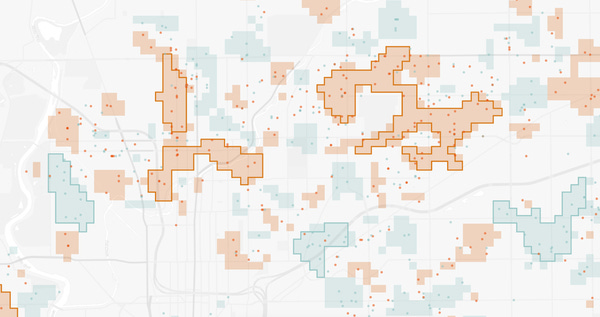
Murder with Impunity: Where Killings Go Unsolved
The Post has mapped more than 52,000 homicides in major American cities over the past decade and found that across the country, there are areas where murder is common, but arrests are rare.
This is an amazing piece of data journalism. My hometown, Baltimore, never fares well on maps like this :/
Leaving aside the content itself (which stands on its own!), the thing I find particularly interesting about the visualizations is that they are maps of rates. This is an underutilized view of the data—companies frequently plot sales by geography on a map, but almost never do the same thing for conversion rate by geography.
www.washingtonpost.com • Share
Rethinking Academic Data Sharing
Roger Peng of Simply Statistics has another angle on GDPR and personal data privacy. Researchers widely value reproducibility, and there is broadly a push towards sharing both the code and data that underly a paper to enable other researchers to reproduce the results. This reproducibility is a cornerstone of the scientific process. But:
I think research on humans is moving in the direction of making it harder to share rather than easier.
This is also true, as laws and norms establishing the privacy rights of individuals begin to take shape. How do we navigate the tradeoff between research reproducibility and personal data privacy? The post is an excellent discussion of the subject.
Data viz of the week
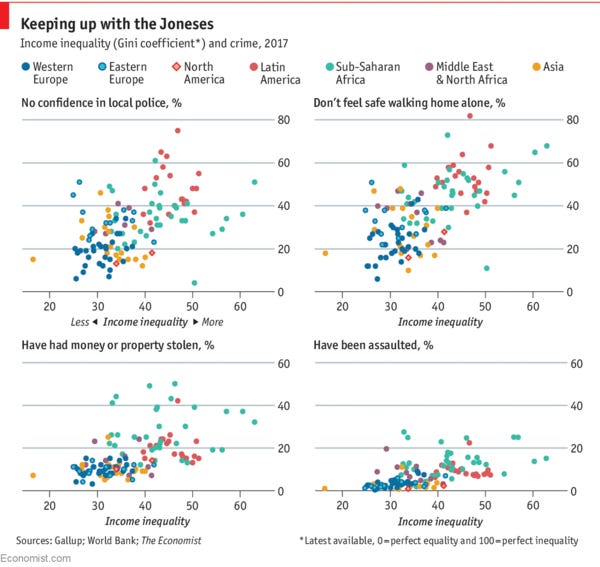
Such a clear illustration.
Thanks to our sponsors!
Fishtown Analytics: Analytics Consulting for Startups
At Fishtown Analytics, we work with venture-funded startups to build analytics teams. Whether you’re looking to get analytics off the ground after your Series A or need support scaling, let’s chat.
Stitch: Simple, Powerful ETL Built for Developers
Developers shouldn’t have to write ETL scripts. Consolidate your data in minutes. No API maintenance, scripting, cron jobs, or JSON wrangling required.
The internet's most useful data science articles. Curated with ❤️ by Tristan Handy.
If you don't want these updates anymore, please unsubscribe here.
If you were forwarded this newsletter and you like it, you can subscribe here.
Powered by Revue
915 Spring Garden St., Suite 500, Philadelphia, PA 19123