Amundsen's Launch. Scaling a Data Team. Resampling. Evolutionary Algorithms. Open Source Segment? [DSR #205]
❤️ Want to support this project? Forward this email to three friends!
🚀 Forwarded this from a friend? Sign up to the Data Science Roundup here.
This week's best data science articles
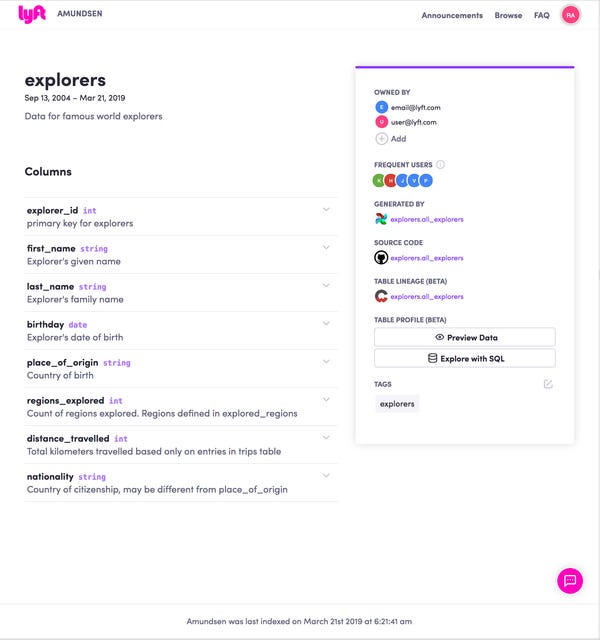
Open Sourcing Amundsen: A Data Discovery And Metadata Platform
Amundsen has been cooking at Lyft for a little while, and I’ve referenced it a bunch of times over the past 6 or so months. This post marks the team’s formal release, including an overview of the product and technical architecture.
This product is important, and I really believe the product category is going to be potentially the single most important product category in data in the coming five years. Everything is going to need to feed the catalog.
How we scaled our data team from 1 to 30 people
In the last three years at Monzo, we’ve scaled a world class data organisation from zero to 30+ people.
When I built my first data organisation five years ago in a small start-up, it took me a year of zig-zagging to get to a decent stage. When I joined Monzo back in 2016, we achieved the same result in a month because I’d learnt lots of lessons before. And it’s no surprise that implementing major changes once your team’s already huge is a lot harder than maintaining an existing high standard as you scale it! For other growing companies looking to scale their data teams, we wanted to share the lessons we’ve learnt so far, to save you some of the trouble! 😅
In this post, we’ll walk through how data works at Monzo, and share the high level principles we’ve used to scale the team.
Highly recommended.
Resampling: The New Statistics
I just came across this full textbook on resampling online. It’s excellent! Resampling is a core tool in the tool belt of any data scientist: if you’re not familiar, read Chapter 1. It’s short and provides a great intro to the topic.
Thanks to @Ben in dbt Slack for the recommendation :D
Computers Evolve a New Path Toward Human Intelligence
Evolutionary algorithms have been around for a long time, but have lost popularity in the face of advances in neural networks. This article introduces the concept of novelty search, which is a rather counterintuitive concept for those of us used to a straightforward gradient descent optimizer moving us towards a well-defined outcome. In tests, optimizing for novelty (as is done in biological evolution) actually outperforms goal-directed optimization when evaluated on performance towards that very goal. Wild.
This is a fairly high-level piece, but links to plenty of resources to drill in deeper. If the topic is interesting to you, this is a good place to dive in.
www.quantamagazine.org • Share
Rudder: An open-source alternative to Segment
Hmmm! Now that title has my attention. I think that a real open source alternative in the data routing / CDP space would be fantastic for users. Segment and others in the space have put vendor lock-in smack in the middle of their business strategies, and there just hasn’t been a great OSS alternative historically. It’s honestly one of the biggest questions I get on consulting calls: “Are there any other options to Segment?” I’d be excited to be able to answer that question with an affirmative, and Rudder is definitely speaking my language with a post titled It’s time we take back control of our data.
The project is early: the first commit in their repo was in July, and the contributors team is small. But I’m excited to watch where it’ll go.
Ever since Judea Pearl’s book came out over a year ago, there has been a renewed interested in causal reasoning in the field. This is one of the most substantive pieces of work I’ve seen actually done on the topic.
Here, we develop causal bootstrapping, a set of techniques for augmenting classical nonparametric bootstrap resampling with information about the causal relationship between variables. This makes it possible to resample observational data such that, if it is possible to identify an interventional relationship from that data, new data representing that relationship can be simulated from the original observational data. In this way, we can use modern machine learning algorithms unaltered to make statistically powerful, yet causally-robust, predictions.
Dense, and early. This is an area worth watching though.
This is an unusual piece for me to link to, and has no specific data science content in it. It’s a short press release by Adobe, the NYT, and Twitter essentially declaring that they were teaming up to solve content attribution on the internet. I can imagine a bunch of ways to attempt this, plenty of them data-science-driven, all of them fragile. It reeks of DRM to me: a mechanism of attempting to control digital content that is fundamentally uncontrollable.
It’s worth a read. Short, concise.
Thanks to our sponsors!
dbt: Your Entire Analytics Engineering Workflow
Analytics engineering is the data transformation work that happens between loading data into your warehouse and analyzing it. dbt allows anyone comfortable with SQL to own that workflow.
Stitch: Simple, Powerful ETL Built for Developers
Developers shouldn’t have to write ETL scripts. Consolidate your data in minutes. No API maintenance, scripting, cron jobs, or JSON wrangling required.
The internet's most useful data science articles. Curated with ❤️ by Tristan Handy.
If you don't want these updates anymore, please unsubscribe here.
If you were forwarded this newsletter and you like it, you can subscribe here.
Powered by Revue
915 Spring Garden St., Suite 500, Philadelphia, PA 19123