Data Career Ladders. Explaining Conversion Rates. Ray. Spotify's ML Infra. [DSR #213]
Quick note! If you use dbt, check out dbt Learn. It’s our two day in-person event that will make you an absolute pro at all things analytics engineering. We just launched five upcoming events from February to April, including LA, Montreal, London, NYC, and SF. Tickets are selling out surprisingly fast (I guess we’ll have to do more of these!), so if you want to join us grab yours now.
- Tristan
❤️ Want to support this project? Forward this email to three friends!
🚀 Forwarded this from a friend? Sign up to the Data Science Roundup here.
This week's best data science articles
What should you focus on this year to get yourself to the next level? How do you help your team do the same? A career ladder is one effective tool to help answer those questions.
This is such a timely post for so many teams I know, very much including our own at Fishtown Analytics. Almost all data teams are started by a single smart generalist who then hires more humans around them as more work is needed. Maybe there are titles that correspond roughly to reality. But does everyone on the team know what their promotion pathway is? Do they know what pay grades are as they continue to get promoted? Are their both senior IC roles and people manager roles opportunities for the future?
Most data teams don’t know these answers. Most data teams hire people for roles, and then try to convince those people to stay in those roles for as long as possible, and then experience a lot of turnover because team members look for their next opportunities elsewhere when internal opportunities don’t materialize. The only teams where this doesn’t seem to be the norm are larger and more established; teams like Spotify, Stitch Fix, etc.
I’m not sharing to this post because it has answers (Caitlin explicitly plans on following it up with more tactical advice in subsequent posts). I’m sharing it because 5-10 years into the evolution of the modern data team and there are a lot of resumes that don’t have any items longer than two years on them. This is suboptimal for the teams, for the humans, and for the field; we need to figure out how to create career paths.
If this is top of mind for you, I’d love to chat.
www.locallyoptimistic.com • Share
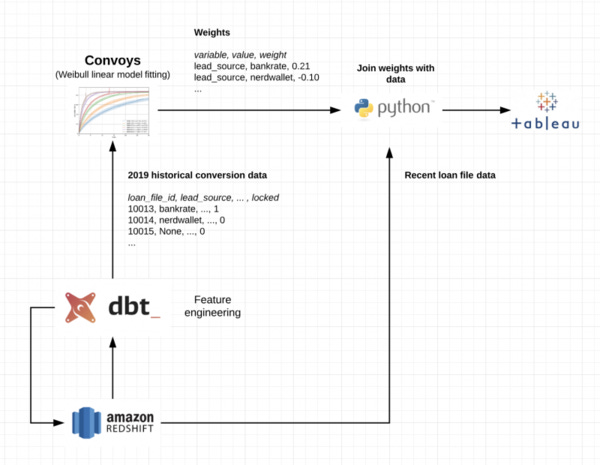
Better's Wizard: An ML tool for interpretable, causal conversion predictions
The timeless question about conversion rates is…why did they go up / down / not move as much as we thought they would? It’s easy to go to a conversion rate chart with a hypothesis and see the rough general direction you expect and attribute that change to your hypothesis. But…that’s just not how that works.
This could actually be the simplest, most effective solution I’ve seen written up for how to answer this persistent question. Useful. Great post by dbt community member Kenny Ning!
Spotify: The Winding Road to Better ML Infrastructure
Spotify has used Machine Learning for over a decade but being systematic about the development and deployment of Machine Learning is a recent introduction.
This post goes quite deep on Spotify’s ML infrastructure. If you’re into that sort of thing, it’s a great post. If not, here’s what I found interesting:
Kubeflow, which I first heard about a year ago, has apparently had a great year. The ecosystem has grown and matured, and Spotify has settled on it as a foundational layer of their ML infra.
Even companies that have cutting edge ML capabilities are still just figuring out how to scale and operate these systems and the associated teams of software engineers. We are still at the early part of the S curve.
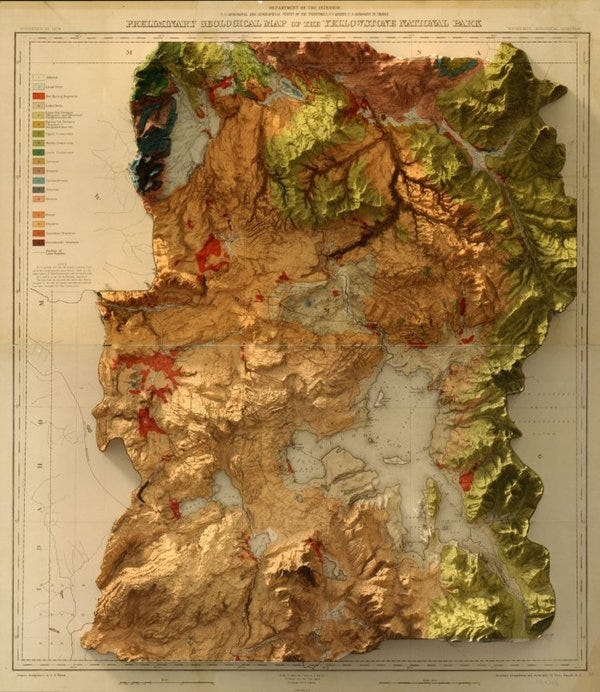
Best Data Visualization Projects of 2019
There is some really fantastic work in this list.
Worker-in-the-loop Retrospective
Author Michael Akilian was co-founder of Clara Labs (since acquired by TopFunnel), a human-in-the-loop scheduling service. This post is rather unique—it’s Michael’s perspective on the entire Human-in-the-Loop space right now. It includes recommendations on where (and where not) to build companies, considerations on how HITL systems should be built, and more.
Even though I personally have no plans to found a HITL company, I found this to be an interesting post on an increasingly-common business model.
Ray – Fast and Simple Distributed Computing
Hmmm! After Berkeley’s AMPLab (Spark’s birthplace) spun down a couple of years ago, RISELab succeeded it. This is the first project I’ve seen come out of the new lab and it seems like the community is really starting to take off. Worth paying attention to.
Thanks to our sponsors!
dbt: Your Entire Analytics Engineering Workflow
Analytics engineering is the data transformation work that happens between loading data into your warehouse and analyzing it. dbt allows anyone comfortable with SQL to own that workflow.
Stitch: Simple, Powerful ETL Built for Developers
Developers shouldn’t have to write ETL scripts. Consolidate your data in minutes. No API maintenance, scripting, cron jobs, or JSON wrangling required.
The internet's most useful data science articles. Curated with ❤️ by Tristan Handy.
If you don't want these updates anymore, please unsubscribe here.
If you were forwarded this newsletter and you like it, you can subscribe here.
Powered by Revue
915 Spring Garden St., Suite 500, Philadelphia, PA 19123