Data Ops. The Importance of "Gut". Curiosity. Model Exploration at Uber. [DSR #170]
❤️ Want to support this project? Forward this email to three friends!
🚀 Forwarded this from a friend? Sign up to the Data Science Roundup here.
This week's best data science articles
This excerpt is solid gold:
in almost any decision-making situation involving data, there is some non-zero percentage of the process that involves “gut”. The reason is because not all information about a process can be incorporated into a data analysis, and it’s important for data analysts to realize that.
This is something that is rarely discussed! I have run into a fair number of people who have very limited capacity to make good strategic decisions on gut. These people instead attempt to answer all such questions via some quantitative process. This is a mistake.
For strategic decisions, modern data analytics can only provide inputs to an ultimate human decision maker who must then incorporate other information sources. We colloquially call the human part of the process “gut”, but what’s actually happening is that:
The human brain has a more sophisticated neural net than we yet know how to build.
We offload data processing to computers when we know how to effectively do so for a given problem domain.
We re-incorporate all externally processed data into our mental model and make a final decision.
In this view, “gut” is a bit like ensembling. You shouldn’t see it as a problem unless you’re actively working on building AGI.
Overplanned Analytics Initiatives Are Doomed to Fail
Benn Stancil, Co-Founder of Mode Analytics, talks to a lot of companies who are kicking off analytics initiatives.
From all the conversations we’ve had, one signal has emerged as the clearest indicator of likely success: The analytics team is agile, and they constantly deliver incremental progress.
In this post, he talks about why companies should be structuring their analytics efforts to be agile. I couldn’t agree with this post more, and this reasoning heavily informs both our product and our consulting strategies at Fishtown Analytics.
blog.modeanalytics.com • Share
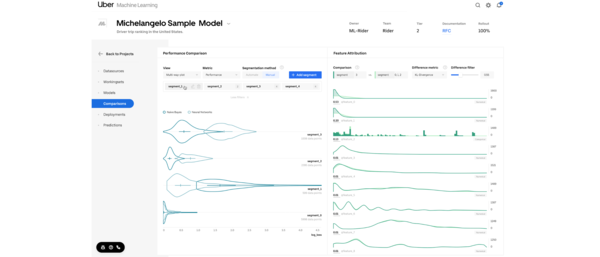
Uber's "Manifold": A Model-Agnostic Visual Debugging Tool for Machine Learning
Uber built Manifold, a model-agnostic visualization tool for ML performance diagnosis and model debugging, to optimize our model iteration process.
Optimizing ML models is hard. It requires a data scientist to hold quite a lot in their brain at once—product designers would say it has high “cognitive load”. High cognitive load tasks are not uncommon for technical fields that are in their nacency (as ML is), but as these fields mature it becomes important to reduce the cognitive load in order to broaden the potential user base.
This is starting to increasingly be a focus in ML. Google’s heavy focus on AutoML is one approach, and providing better tooling to model builders to do their own tuning (what Uber is doing with Manifold) is another.
I’m personally very interested in the “make ML accessible” trend and think we’re still in the mainframe phase—big, centralized, inaccessible (except to the high priests).
Stitch Fix | Let Curiosity Drive: Fostering Innovation in Data Science
The author is the Chief Algorithms Officer for Stitch Fix. The post is about how curiosity, not a structured process, is responsible for the highest-value data science outcomes.
The real value of data science lies not in making existing processes incrementally more efficient but rather in the creation of new algorithmic capabilities that enable step-function changes in value. However, such capabilities are rarely asked for in a top-down fashion. Instead, they are discovered and revealed through curiosity-driven tinkering by data scientists.
Highly recommended. The ideas are not totally out totally foreign, but they’re presented in a way that will help you see them in a new light.
multithreaded.stitchfix.com • Share
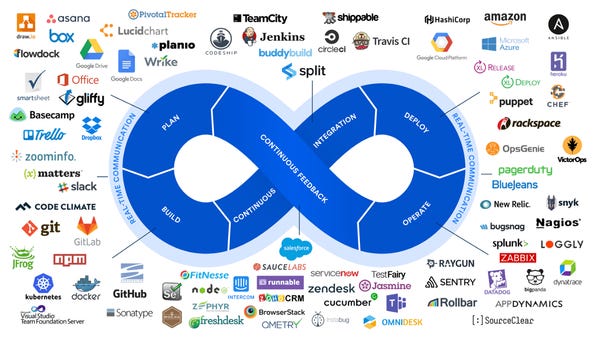
DataOps as Part of a New Enterprise Stack
Great post on the emerging “DataOps” space. The more data flows around, the more we need tooling to both get it where it needs to go and monitor the pipelines that it flows through. Many of the tools in the DataOps stack are the same as the ones in the DevOps stack, but the users and use cases are very different.
I frequently take a very startup-centric view of this ecosystem because of the world I operate in, but the author (from enterprise-focused vendor Tamr) has a view that is much more focused on the enterprise. The post was originally released over the summer but I didn’t come across it until now and found it to be a valuable addition to my personal mental model.
Thanks to our sponsors!
Fishtown Analytics: Analytics Consulting for Startups
At Fishtown Analytics, we work with venture-funded startups to build analytics teams. Whether you’re looking to get analytics off the ground after your Series A or need support scaling, let’s chat.
www.fishtownanalytics.com • Share
Stitch: Simple, Powerful ETL Built for Developers
Developers shouldn’t have to write ETL scripts. Consolidate your data in minutes. No API maintenance, scripting, cron jobs, or JSON wrangling required.
The internet's most useful data science articles. Curated with ❤️ by Tristan Handy.
If you don't want these updates anymore, please unsubscribe here.
If you were forwarded this newsletter and you like it, you can subscribe here.
Powered by Revue
915 Spring Garden St., Suite 500, Philadelphia, PA 19123