Data Organizational Complexity. The Enterprise Tech Landscape. Tracking Pixels. Skewed Data. [DSR #214]
❤️ Want to support this project? Forward this email to three friends!
🚀 Forwarded this from a friend? Sign up to the Data Science Roundup here.
This week's best data science articles
In the modern data ecosystem, organizational knowledge is no longer created by single domain experts. It's created by teams collaborating, iteratively learning, cataloging, and making that knowledge available to the whole organization. https://t.co/NdNRPQqPL3
I’ve been thinking a lot about this topic recently. The modern data stack is fantastic, and for those of us who have literally decades of experience in data, it turns us into superhumans. But it actually can create a significant barrier to entry to getting many questions answered for many people. From my just-written post, Analytics Engineering for Everyone, on the topic:
In a prior generation of data, a single person could acquire all of the relevant skills to become an expert: both a domain area expert as well as an expert in the relevant data technology. Data wasn’t that big or diverse, and typically people acquired the necessary data skills (Excel, simple SQL, SAS) as their problems required. The data was small in size and scope, but the process worked fine. Just grab a CSV and get to work.
This broke down with the advent of the modern data stack. Today, the possibilities for analysis have grown dramatically, but the breadth and depth of skills required has also grown. This means that it’s no longer enough for a single domain expert to sit down at a computer and geek out for a while to get an answer. Getting answers to questions in the modern data ecosystem requires teams working together in lockstep, using a well-thought-out workflow, with tooling built to enable this collaboration.
Modern data tech, especially the hyperscale cloud data warehouse, has unlocked so much potential. And we as an industry haven’t come even close to figuring out the tooling, workflows, and skillsets required to actually digest this change. This makes me think a lot about a recent Ben Thompson post where he makes the point that car companies stopped seeing a lot of innovation after 1920, but that the car continued to drive massive shifts in society throughout the entire 20th century.
It’s completely possible that the race to build a lot of the core technology in the modern data stack is already over! But we are at the very beginning of seeing the changes that will drive in our organizations. That topic—how organizations will adapt in response to modern data tech—is on my brain these days.
Stephen O'Grady, of RedMonk, publishes some of the best thinking on the enterprise software space today. This post isn’t specifically focused on data, but the data ecosystem participates in the megatrends of the larger enterprise software space. As such, I find that keeping up with trends in application development and deployment, cloud providers, etc. is quite useful as I build my mental model.
This post poses five questions, the answers to which will determine what 2020 will look like in enterprise tech.
How does Facebook know that you went to Old Navy?
It’s increasingly common for data analysts and scientists to work with raw event data directly in their data warehouses, which is great—until recently these folks only had access to aggregated data which left them hamstrung. But I’ve found that very few of these analysts and scientists actually understand how cookies, javascript, and the HTTP request/response cycle operate. And without understanding what exactly is happening here this data can all feel rather mysterious and arbitrary.
If this sounds like you, this post will help fix that.
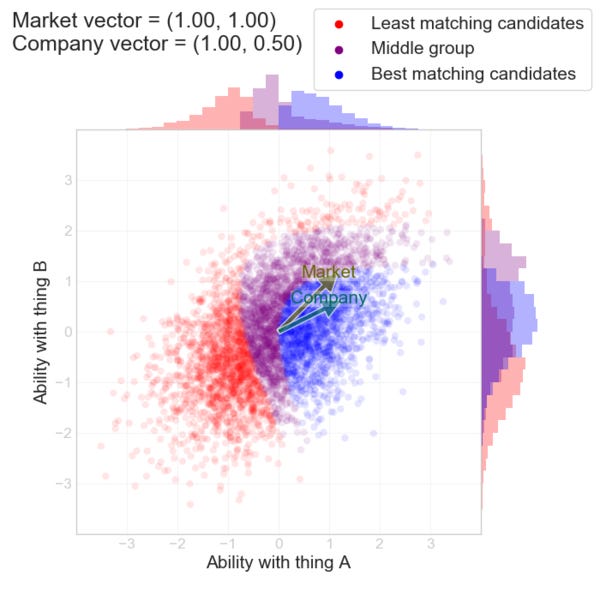
How to hire smarter than the market: a toy model
Another great post from Erik Bernhardsson:
What my model implies, is that there’s an “arbitrage opportunity” here. In fact, it’s a bit of a silver lining to the fact that the market is biased. Are companies systematically putting a premium on something? Then bet against them! Go after the underdogs.
This post introduced me to Berkson’s Paradox, a concept so simple that if you hadn’t seen it described before you might not have realized that it was so commonly-occurring (or that it needed a name at all!). But thinking clearly about this dynamic in recruiting, home-buying, or in so many other contexts has massive benefits in shaping your strategy.
Another Vicki Boykis special, this post isn’t specifically about AI or Google, it’s actually about health care. But it demystified something for me that I see all the time and yet have become pretty much inured to: constant announcements from big tech about how they had recently created some model that demonstrated some diagnostic metric that was better than human doctors. Seriously, these announcements and the coverage of them happens so often that the casual reader (me?) would be led to believe that all radiologists were already out of jobs. But…I have a relative who is a radiologist and I can confirm that this is very much not the case.
So…what is going on? This post takes a little while to get there, but it’s a great read. I honestly can’t even attempt to summarize the conclusions that Vicki comes to but her take feels dead-on to me.
How to Demystify Skewed Data and Deliver Analysis
This is just a little beginner-y, but I think it’s a surprisingly concise, effective writeup of the topic. What’s more, I’m linking to it because so many junior data analysts and scientists just…skip this step. Going straight to aggregation without meaningfully digging into the underlying data is a bad idea.
What’s your personal track on this? Often, in environments that move quickly, there is an incentive to skip this type of work and instead just give an answer. Push back against this gravity!
Thanks to our sponsors!
dbt: Your Entire Analytics Engineering Workflow
Analytics engineering is the data transformation work that happens between loading data into your warehouse and analyzing it. dbt allows anyone comfortable with SQL to own that workflow.
Stitch: Simple, Powerful ETL Built for Developers
Developers shouldn’t have to write ETL scripts. Consolidate your data in minutes. No API maintenance, scripting, cron jobs, or JSON wrangling required.
The internet's most useful data science articles. Curated with ❤️ by Tristan Handy.
If you don't want these updates anymore, please unsubscribe here.
If you were forwarded this newsletter and you like it, you can subscribe here.
Powered by Revue
915 Spring Garden St., Suite 500, Philadelphia, PA 19123