Data Quality @ Intuit. Discovery @ Lyft. Integrating Models into Real-World Systems. Death By Experiment. [DSR #183]
❤️ Want to support this project? Forward this email to three friends!
🚀 Forwarded this from a friend? Sign up to the Data Science Roundup here.
This week's best data science articles
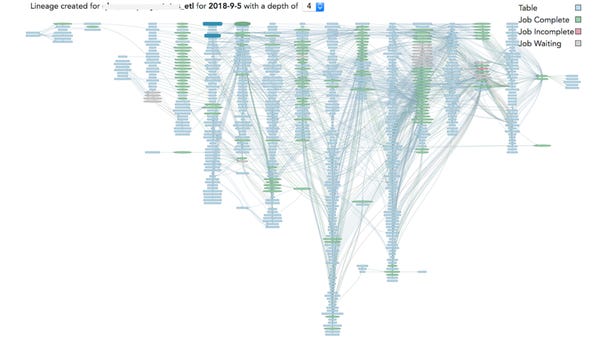
Intuit: Taming Data Quality with Circuit Breakers
I love this post—this is my favorite thing I’ve read for a little while. It talks about the Quickbooks data engineering team’s tools and process for monitoring their data pipelines, from ingestion to transformation to serving. Data quality is often the single biggest time suck for a data team, and too few teams have automated tools to monitor it.
I think their concept of a circuit breaker is interesting, but I’m not sold on it. Instead, the thing that is so fascinating is all the work they’ve done prior to that point. Their thousands of jobs all collect both operational profiling and data profiling metrics, and yours probably should as well.
We’re still early in the modern data engineering game. Most data engineers are focused on making pipelines work at all, and haven’t yet had the luxury of building robust tooling for monitoring. The industry as a whole will get there, but it will take time. This is a topic that I plan on following closely.
quickbooks-engineering.intuit.com • Share
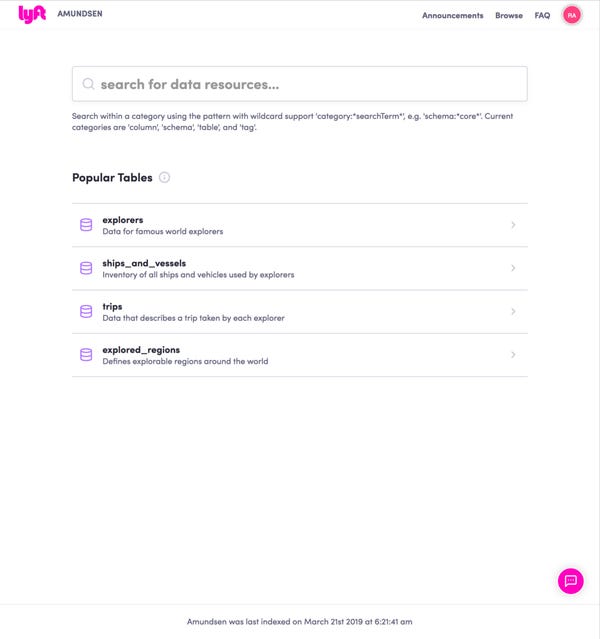
Amundsen — Lyft’s Data Discovery & Metadata Engine
Another topic I think is incredibly important: data discovery and curation! If your organization is of sufficient size, how do users:
learn about new datasets that they have never worked with before?
understand the provenance of the data that their reports are built on?
know who to go to for more information?
…and more. Operating a data infrastructure at a company of 80 people is a completely different challenge than operating a data infrastructure for a company of 5,000 people, with the core difference being that you can’t just shoulder-tap someone to get an answer. Lyft realized that 25% of their data teams’ time was spent just trying to find the relevant data. That number was even higher when I worked at GE.
This post goes through Lyft’s tool, Amundsen, that indexes their internal datasets and exposes that information to users. Amazing read, and very informative if you’re a dbt user—this is exactly the experience we’re on the path to facilitating for all dbt users with dbt Docs.
From the article: “IMHO the following topics are completely undervalued and deserve way more attention from the machine learning community:”
Problem Formulation: Translate a problem into a prediction or pattern recognition problem.
Data-Generating Process: Understand the data, its limitations and suitability for solving the problem.
Model Interpretation: Analyze the model beyond cross-validated performance estimates.
Application Context: Reflect how the model will interact with the world.
Model Deployment: Integrate the model into a product or process.
💯
Misadventures in experiments for growth
Large-scale live experimentation is a big part of online product development. In fact, this blog has published posts on this very topic. With the right experiment methodology, a product can make continuous improvements, as Google and others have done. But what works for established products may not work for a product that is still trying to find its audience. Many of the assumptions on which the “standard” experiment methodology is premised are not valid. This means a small and growing product has to use experimentation differently and very carefully. Indeed, failure to do so may cause experiments to mislead rather than guide. This blog post is about experimentation in this regime.
So good. I’ve been at the fledgeling company described in this post. Don’t over-rely on experimentation before you have an established product!
www.unofficialgoogledatascience.com • Share
Open Questions about Generative Adversarial Networks
What we’d like to find out about GANs that we don’t know yet.
The author is from the Google Brain team. The post itself is the most extensive overview I’ve read on state-of-the-art GAN research. Technical, but a truly unique resource if this is a topic you care about.
Actively curated list of data tools. PRs welcome!
Well this is certainly a much-needed resource. As the data ecosystem is expanding so rapidly, it’s incredibly hard to keep track of the variety of tools available. I just spent the past 30 minutes exploring tools I had never heard of before. Have a favorite that’s not currently on the list? Submit a PR!
Thanks to our sponsors!
Fishtown Analytics: Analytics Consulting for Startups
At Fishtown Analytics, we work with venture-funded startups to build analytics teams. Whether you’re looking to get analytics off the ground after your Series A or need support scaling, let’s chat.
www.fishtownanalytics.com • Share
Stitch: Simple, Powerful ETL Built for Developers
Developers shouldn’t have to write ETL scripts. Consolidate your data in minutes. No API maintenance, scripting, cron jobs, or JSON wrangling required.
The internet's most useful data science articles. Curated with ❤️ by Tristan Handy.
If you don't want these updates anymore, please unsubscribe here.
If you were forwarded this newsletter and you like it, you can subscribe here.
Powered by Revue
915 Spring Garden St., Suite 500, Philadelphia, PA 19123