Data Science Roundup #51: Not Enough Data Engineers, DeepDress, and A/B Testing at Lyft
This week I’m incredibly proud to share a just-released report I helped author called The State of Data Engineering. It’s the result of hundreds hours of research and editorial work analyzing millions of Linkedin profiles and conducting interviews with top data engineering leaders. I’d love to hear your feedback!
- Tristan
This week's best data science articles
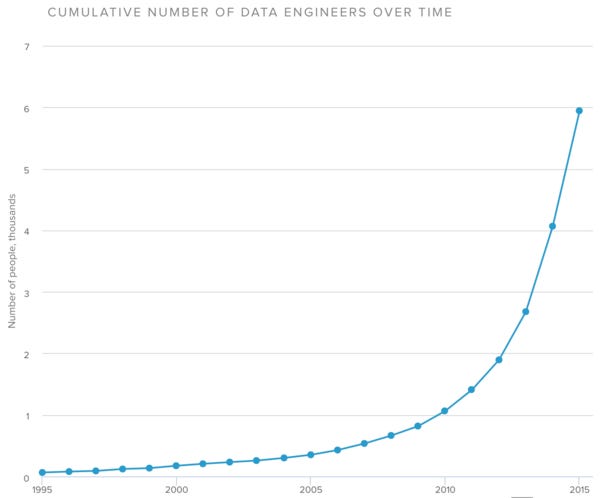
Today, there are 6,500 people on LinkedIn who call themselves data engineers. In San Francisco alone, there are 6,600 job listings for this same title. The number of data engineers has doubled in the past year, but engineering leaders still find themselves faced with a significant shortage of data engineering talent. This report shares hard data and interviews from leading experts.
2016 Data Science Salary Survey
This year’s must-read salary guide for data science practitioners. Key findings:
Python and Spark are among the tools that contribute most to salary.
Among those who code, the highest earners are the ones who code the most.
SQL, Excel, R and Python are the most commonly used tools.
Those who attend more meetings earn more.
A Technical Primer On Causality
What does “causality” mean, and how can you represent it mathematically? How can you encode causal assumptions, and what bearing do they have on data analysis? This is an ambitious post—if you’re caffeinated and ready to use your brain then I highly recommend it. Otherwise, save it for later.
Ohhhh yes. A data scientist at RentTheRunway decided to throw together, you know, a quick neural net that classified tagged images on Instagram with their most likely RTR product. The tool is called—wait for it—DeepDress and turns out to be both effective and pleasing (click through to see a very cute puppy in a dress). Code included.
Experimentation in a Ridesharing Marketplace
If you’ve done any online experimentation, you may not have had to think too hard about the mechanism by which users are partitioned into treatment and control groups. Most A/B tests use naive randomization, which is completely appropriate for many scenarios. This post explains how experimentation in networks like Lyft’s inherently causes interference between the groups, which can significantly impact the validity of the experiment. Highly recommended.
Human and Artificial Intelligence May Be Equally Impossible to Understand
Model interpretability is important, but I don’t know that we have good reason to expect to be able to reduce the interactions of millions of nodes into understandable rules. From the article: “If ‘understanding’ in this field does come, it could be of the sort found not in physics, but evolutionary biology. Rather than Principia, we might expect Origin of the Species.” Excellent article on an important topic.
Data viz of the week
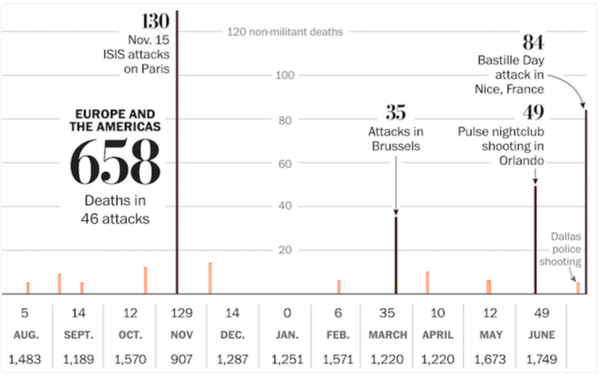
It's the small things: note the y-axis labels.
Thanks to our sponsors :D
Fishtown Analytics is a boutique analytics consultancy serving high-growth, venture-funded startups. Have analytics questions? Let’s chat.
Developers shouldn’t have to write ETL scripts. Consolidate your data in minutes. No API maintenance, scripting, cron jobs, or JSON wrangling required.
The internet's most useful data science articles. Curated with ❤️ by Tristan Handy.
If you don't want these updates anymore, please unsubscribe here.
If you were forwarded this newsletter and you like it, you can subscribe here.
Powered by Revue
915 Spring Garden St., Suite 500, Philadelphia, PA 19123