Data Science Roundup #56: Doing Away with Significance, Machine Learning for the Masses, and more!
Saying goodbye to p-values; machine learning will be commoditized (what will that look like?); a single human is distorting presidential polls due to a methodological quirk; an impressive tour through Jupyter advanced features; Harry Potter spell usage; Strata recap.
Enjoy :)
- Tristan
This week's best data science articles
It’s time for science to abandon the term ‘statistically significant’
I’ve been covering the replication crisis for over a year at this point and believe it is profoundly important. Most articles on the topic point to systemic problems, but I’ve never seen someone go so far as to assert that we’re fundamentally doing statistics incorrectly. Here’s a quote: “Even quite respectable sources will tell you that the p-value is the probability that your observations occurred by chance. And that is plain wrong.”
You should read this article.
The Commoditization of Machine Learning
Technologies become more accessible over time—abstractions get built around the core innovation that make that innovation more accessible to a wider audience. This article traces that history in web and mobile, and then makes the natural transition to machine learning. How far are we down the path of abstraction in ML? What will ML need to look like in order to make it more widely accessible?
How One 19-Year-Old Illinois Man Is Distorting National Polling Averages
It seems that the current US election has created a new type of data geek, and apparently there are enough of us to get a post on polling methodology shared 40,000 times. The headline of this article is not hyperbole: there is literally a single individual who, because of the idiosyncratic grouping and weighting process of one particular poll, is shifting the results by an entire percentage point all by himself. This story is interesting in and of itself, but begs the larger question: what unintentional, quirky biases exist in our analyses and how can we ferret them out?
A Power-User's Guide to Jupyter Notebook
Jupyter is everywhere, but for all its popularity, most users (myself very much included) are only scratching the surface of its functionality. This post goes deep into magic functions, hotkeys, plotting, and many many more features of Jupyter that will take you from novice to professional.
arogozhnikov.github.io • Share
All the Harry Potter spells and When they were Used
Ok, this is just cool. While completely trivial, this visualization does a great job of exploring a dimension of the Harry Potter series. Did you know that Avada Kedavra, the killing curse, was first introduced in Book 4: Goblet of Fire? Same for Crucio, the torture curse. The later books are where things got serious. This is an excellent exploratory visualization.
Strata 2016 and the rise of New Data
Data Science Roundup subscriber Vicki Boykis wrote an excellent comparison of Strata 2016 and Strata 2013, when “90% of the talks were just about correctly configuring Hadoop clusters so they didn’t break.” This post is a great summary of the single largest data conference in the world. The industry is finally talking about ethics, trust, and privacy.
Data viz of the week
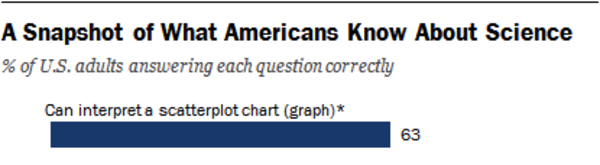
37% of Americans cannot interpret a scatterplot 😢 😢 😢
Thanks to our sponsors!
Fishtown Analytics is a boutique analytics consultancy serving high-growth, venture-funded startups. Have analytics questions? Let’s chat.
Developers shouldn’t have to write ETL scripts. Consolidate your data in minutes. No API maintenance, scripting, cron jobs, or JSON wrangling required.
The internet's most useful data science articles. Curated with ❤️ by Tristan Handy.
If you don't want these updates anymore, please unsubscribe here.
If you were forwarded this newsletter and you like it, you can subscribe here.
Powered by Revue
915 Spring Garden St., Suite 500, Philadelphia, PA 19123