Data Science Roundup #88: Airbnb's Data University, Sample Bias, AlphaGo & SparkR
Solid week this week. I’d be especially interested in your thoughts on the first article about spreading data competency through organizations. What are you doing to address this at your company?
Also: as we head into the summer, the news cycle often gets a bit less active. I’d love to take the time to share writing from the Data Science Roundup community—send me your posts!
- Tristan
❤️ Want to support us? Forward this email to three friends!
🚀 Forwarded this from a friend? Sign up to the Data Science Roundup here.
Two Posts You Can't Miss
How Airbnb Democratizes Data Science
I’ve become fascinated by the challenge of bridging the gap between data professionals and the rest of an organization. I’ve spoken to several large organizations recently who struggle with this: in each case, they have data but it simply doesn’t get used. This is somewhat mind-boggling to me, but it turns out that in multi-thousand-person organizations, it’s totally possible to lack the skills necessary to formulate relevant questions and then answer them with data.
Airbnb has a massive data organization (100+ people on their data science team!) but they still struggle with bridging this gap. This post is on their attempt to democratize data throughout their organization, and it’s awesome:
Another one of our fundamental beliefs is that every employee should be empowered to make data informed decisions. This applies to all parts of Airbnb’s organization — from deciding whether to launch a new product feature to analyzing how to provide the best possible employee experience. Our Data Science team firmly believes that part of our goal is to empower the company to understand and work with data. In order to inform every decision with data, it wouldn’t be possible to have a data scientist in every room — we needed to scale our skillset.
Must read.
The Subtle Sources of Sampling Bias Hiding in Your Data
Plummeting data acquisition costs have been a big part of the surge in business analytics. We have much richer samples of data to use for insight. But more data doesn’t inherently remove sampling bias; in fact, it may make it worse.
This is a really excellent article. Very readable, and makes several points that any modern data practitioner should always keep in mind. Here’s another great quote:
Historically, variation within a sample has been used to infer sampling error. With increased data volumes, statistical significance is trivial to find but distracts from the larger point of sampling error; the sample may be internally consistent but not reflect the desired population. Data volume then gives false comfort.
Sound familiar?
This Week's Top Posts
Data scientists give many, many talks. Sharing work internally with other data scientists, internally with project stakeholders, externally at meetups, or externally at conferences. So many talks.
In my experience, many talks by data scientists are…not great. It is hard to talk about your work! And data science as a subject matter is hard to communicate about, period. This is the single best article I’ve ever read on how to prepare for a talk. Read it. Steal ideas from it. Make your next talk awesome.
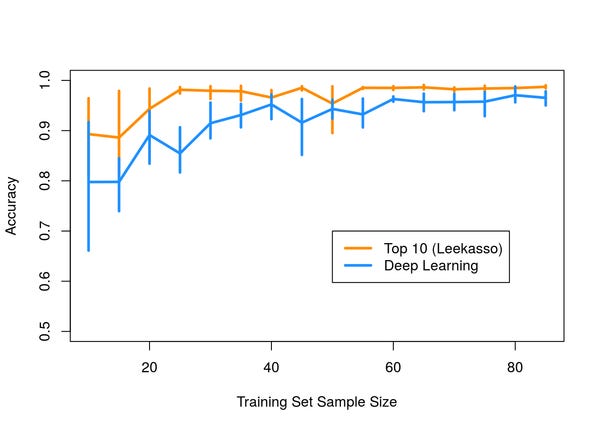
Don't use deep learning; your data isn't that big
Practitioners are using deep learning in wildly inappropriate use cases today. This author is here to tell you to cut the shit and use a simpler model.
Refreshing.
Andrej Karpathy of OpenAI writes:
I had a chance to talk to several people about the recent AlphaGo matches with Ke Jie and others. In particular, most of the coverage was a mix of popular science + PR so the most common questions I’ve seen were along the lines of “to what extent is AlphaGo a breakthrough?”, “How do researchers in AI see its victories?” and “what implications do the wins have?”. I thought I might as well serialize some of my thoughts into a post.
Andrej finds that AlphaGo says more about Google than it says about the wider industry: AlphaGo is fundamentally a narrow AI that doesn’t translate well into real world applications, but the technology and corresponding expertise bode well for future deep learning initiatives within Google.
Applying deep learning to real-world problems
Excellent post that covers three lessons on how to go from nothing to a working model in a real-world setting. The first section of the post focuses on pre-training, an under-appreciated topic.
Highly recommended, getting a lot of traction.
FairML: Auditing Black-Box Predictive Models
How do we know if a machine learning model is fair? And what does fairness in machine learning mean?
The author walks through, in detail, how to evaluate a black box model using perturbation and correction for multicollinearity. If you’ve ever needed to evaluate a model, this is an excellent read: great diagrams, includes code.
blog.fastforwardlabs.com • Share
Machine Learning using Spark and R
Until recently, R has gotten short shrift in the Spark ecosystem, but since Spark 2.1, that’s all changed. If you haven’t used R with your Spark cluster yet, this is an excellent getting started guide.
Data Viz of the week
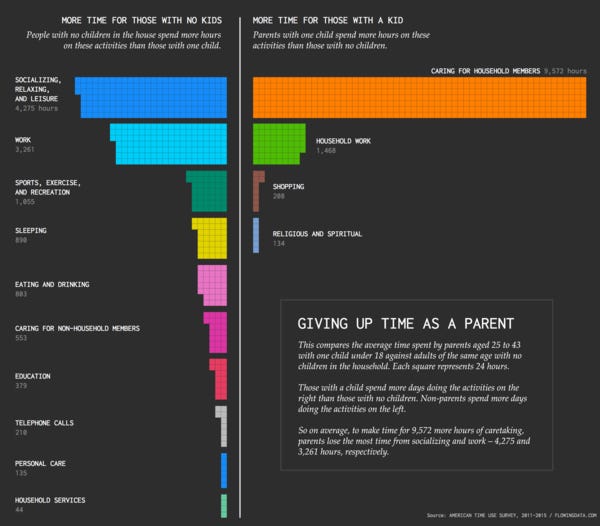
Immediate impact, clear story.
Thanks to our sponsors!
Fishtown Analytics: Analytics Consulting for Startups
At Fishtown Analytics, we work with venture-funded startups to implement Redshift, Snowflake, Mode Analytics, and Looker. Want advanced analytics without needing to hire an entire data team? Let’s chat.
Stitch: Simple, Powerful ETL Built for Developers
Developers shouldn’t have to write ETL scripts. Consolidate your data in minutes. No API maintenance, scripting, cron jobs, or JSON wrangling required.
The internet's most useful data science articles. Curated with ❤️ by Tristan Handy.
If you don't want these updates anymore, please unsubscribe here.
If you were forwarded this newsletter and you like it, you can subscribe here.
Powered by Revue
915 Spring Garden St., Suite 500, Philadelphia, PA 19123