Data Science Roundup #91: Paradoxes, empathy, and lower entry-level salaries(!?)
This is an atypical issue, where I (mostly) take a break from focusing on implementation questions. Instead:
How can you use stories to dissuade people from faulty statistical thinking?
How can we apply empathy and design thinking to algorithm development?
How can you explain the diverse roles of your data team members to “normals” throughout your org?
Feedback welcome!
- Tristan
❤️ Want to support us? Forward this email to three friends!
🚀 Forwarded this from a friend? Sign up to the Data Science Roundup here.
Two Posts You Can't Miss
Paradoxes of Probability and Other Statistical Strangeness
Nowadays, researchers can access a wealth of software packages that can readily analyse data and output the results of complex statistical tests. While these are powerful resources, they also open the door to people without a full statistical understanding to misunderstand some of the subtleties within a dataset and to draw wildly incorrect conclusions.
I love this post so much—it pokes at a topic that I think is incredibly important in all of our professional (and personal!) lives today: the inability of most people to reason statistically.
As a data scientist, it isn’t your job to find the right answer: it is your job to convince other people of the right answer. Knowing that something is true is completely without value if that knowledge doesn’t affect change in the world, and that almost always requires consensus.
I think of these paradoxes—Simpson’s paradox, Berkson’s paradox, Will Rogers paradox—like fables: they’re short anecdotes that teach statistical reasoning. Like most fables, repetition is the key. Know these by heart and reference them when explaining why a particular line of reasoning is faulty.
Without human purpose, a computer is just a rock that we tricked into thinking.
Evaluating the impact of ML models is a hot topic today, but this is the first writing I’ve seen that incorporates human outcomes into the process of algorithm design. This post by Data Science Roundup subscriber Chris Butler does just that: it reframes the construction of ML systems as “empathy maps”, and asks what the algorithm needs to do, sense, say, think, and feel.
It seems like we’re about as good at designing algorithms today as software developers in the 70’s were at building mainframe systems. While technology has certainly improved in the ensuing years, so too has the way we have thought about constructing such systems.
While I don’t know whether this particular approach is specifically the answer, I anticipate much more design thinking applied to algorithms.
This Week's Top Posts
Wow. The EFF has a team tracking AI progress, and they put together this truly behemoth collection of top results. If you scroll to the end, you can see a table of every problem that they’ve catalogued, including a “solved” or “not solved” indicator. I’ve never seen a more comprehensive listing of results.
Fascinating to me: it turns out that folks at Deepmind are attempting to reconstruct the entire ruleset of Magic: The Gathering purely from the content of the cards (in the same way that a human would). Result so far? Not solved. (Not even close!)
Data Science Entry Level Salaries are Down
This Burtch Works survey has been making the rounds over the past week, and it shows some interesting stuff. Broadly, the hype and the high salaries in data science have caused an influx of new junior hires, causing a slight decrease in entry level salaries.
While there are plenty of people who complain about data science programs “printing” data scientists without key skills and experience, to me that feels like a good thing—that’s why we refer to those positions as entry level. Time to get out in the real world and learn!
BigQuery vs Redshift vs Athena
This is the first comparison I’ve seen between BigQuery and Athena since Athena was released last year. Overall, it seems like BigQuery’s performance is generally better while Athena is generally cheaper.
Big caveat here: this analysis intentionally ignores partitioning, which is possible in each platform (albeit differently). So, these results are instructive on relative performance but aren’t representative of the way an optimized implementation would perform in the real world.
What's the difference between a data analyst, scientist and engineer?
Ok, this is probably not new information for you, but there are probably plenty of folks in your organization who could use help understanding the different roles on a modern data team. This is a great resource if you find yourself in that conversation.
While most of the machine learning talent works in big tech companies, massive and timely problems are lurking in every major industry outside tech.
The author’s thesis: if you want to build a company in AI, find a non-tech vertical and build an end-to-end solution. Completely and totally agree.
Automatic Tools for Improving R Packages
Run R CMD check, you fools!
Yes!
Data Viz of the Week
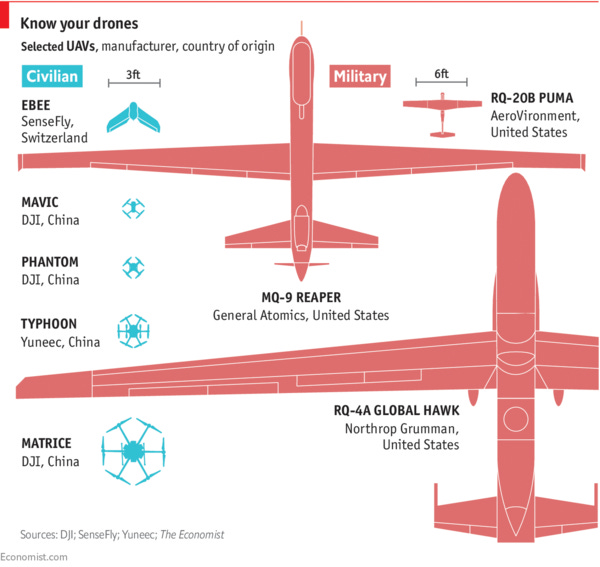
So interesting. Makes me immediately want to know more.
Thanks to our sponsors!
Fishtown Analytics: Analytics Consulting for Startups
At Fishtown Analytics, we work with venture-funded startups to implement Redshift, Snowflake, Mode Analytics, and Looker. Want advanced analytics without needing to hire an entire data team? Let’s chat.
Stitch: Simple, Powerful ETL Built for Developers
Developers shouldn’t have to write ETL scripts. Consolidate your data in minutes. No API maintenance, scripting, cron jobs, or JSON wrangling required.
The internet's most useful data science articles. Curated with ❤️ by Tristan Handy.
If you don't want these updates anymore, please unsubscribe here.
If you were forwarded this newsletter and you like it, you can subscribe here.
Powered by Revue
915 Spring Garden St., Suite 500, Philadelphia, PA 19123