Deep Learning Popularity. Privacy @ Spotify. A/B Testing Research from Facebook. Learning DS on a Budget. [DSR #154]
❤️ Want to support us? Forward this email to three friends!
🚀 Forwarded this from a friend? Sign up to the Data Science Roundup here.
This Week's Most Useful Posts
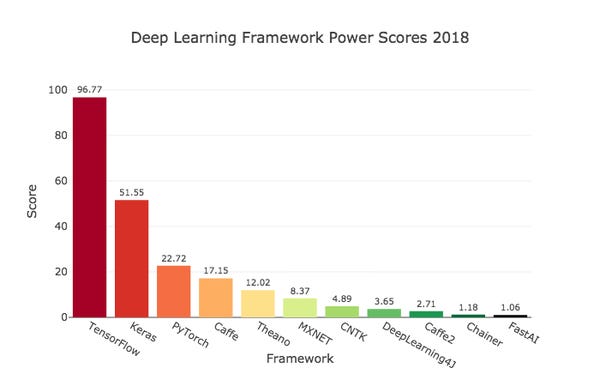
Deep Learning Framework Power Scores 2018
Author Jeff Hale has built a “power score” ranking of deep learning frameworks:
I used 11 data sources across 7 distinct categories to gauge framework usage, interest, and popularity. Then I weighted and combined the data…
The final results are in the chart above. Data sources include:
KDnuggets Usage Survey
Google Search Volume
Medium Articles
Amazon Books
ArXiv Articles
GitHub Activity
If you’re starting a new deep learning project, by all means, choose your framework based on technical requirements. But if you’re curious about adoption / popularity, this is a solid benchmark. Users exert gravity in winner-take-all technology ecosystems; I expect the top frameworks to only become more popular and more feature-rich as the space matures.
towardsdatascience.com • Share
How To Learn Data Science If You’re Broke
Over the last year, I taught myself data science. I learned from hundreds of online resources and studied 6–8 hours every day. All while working for minimum wage at a day-care.
This is one of my favorite “how I learned data science” / curriculum posts. The author presents a clear series of steps, short advice for each step, and then rounds out the post with some general advice at the end. My favorite bit:
Data Science + ___ = A Passionate Career. Fill in the blank.
Totally agree. Most people aren’t excited purely by data itself; they are excited about applications. Figuring out what applications motivate you will help you learn!
towardsdatascience.com • Share
Spotify: Scalable User Privacy
One of the standards we have set at Spotify is that personal data of our users can only be persisted when it is encrypted.
Wow. Just think about that for a second: every single piece of at-rest data associated with a user is encrypted throughout Spotify’s entire infrastructure. Thousands of microservices, thousands of datasets.
This article is a fascinating internal look at how a company that is committed to user privacy architects their data systems. While this strategy is probably not within reach for most of you today, the behind-the-scenes look at how a leading company does this is invaluable.
Nathan Yau analyzed 40,000 recipes from 20 cuisines to find the most common and the biggest outlier ingredients. It’s a surprisingly simple analysis, but I’d never seen it before and it’s brought to life with traditional Flowing Data flair. Interesting facts I learned:
The most common ingredient in each cuisine is either salt, or the regional salt-substitute (like soy sauce).
The most cuisine-specific ingredient for each cuisine is totally guessable if you are familiar with the cuisine, and trying to guess them is quite fun. For example: Russian? Beets. Italian? Ricotta.
I did not realize how much Jamaicans like allspice. Go figure.
I really enjoyed this analysis and presentation.
The Trinity Of Financial Modeling Errors
Errors in analysis and forecasting may arise from any of the following modeling issues: using an inappropriate functional form, inputting inaccurate parameters, or failing to adapt to structural changes in the market.
Excellent post. Short, correct, and somehow still contrarian even after 2008 clearly demonstrated the problems with much of “traditional” modeling done in finance. Finance textbooks still have not been rewritten.
FB Research: Efficient Tuning of Online Systems Using Bayesian Optimization
A/B tests are often used as one-shot experiments for improving a product. In our paper (…), we describe how we use an AI technique called Bayesian optimization to adaptively design rounds of A/B tests based on the results of prior tests. Compared to a grid search or manual tuning, Bayesian optimization allows us to jointly tune more parameters with fewer experiments and find better values.
The post goes into examples and shares data from usage Facebook’s just-published methodology. Important.
Thanks to our sponsors!
Fishtown Analytics: Analytics Consulting for Startups
At Fishtown Analytics, we work with venture-funded startups to build analytics teams. Whether you’re looking to get analytics off the ground after your Series A or need support scaling, let’s chat.
www.fishtownanalytics.com • Share
Stitch: Simple, Powerful ETL Built for Developers
Developers shouldn’t have to write ETL scripts. Consolidate your data in minutes. No API maintenance, scripting, cron jobs, or JSON wrangling required.
The internet's most useful data science articles. Curated with ❤️ by Tristan Handy.
If you don't want these updates anymore, please unsubscribe here.
If you were forwarded this newsletter and you like it, you can subscribe here.
Powered by Revue
915 Spring Garden St., Suite 500, Philadelphia, PA 19123