Druid @ Reddit. Principles for Building a Data Catalog. Third-Party Cookies. AI Power Dynamics. [DSR #247]
❤️ Want to support this project? Forward this email to three friends!
🚀 Forwarded this from a friend? Sign up to the Data Science Roundup here.
This week's best data science articles
Google’s FLoC Is a Terrible Idea | EFF
The third-party cookie is dying, and Google is trying to create its replacement.
Apple has been pushing hard on privacy-as-competitive-advantage for a few years now, and last year made blocking third-party cookies the default on all its platforms. This move has been one of the most significant in an industry-wide shift away from third-party cookies (cookies that are associated with owners other than the owner of the domain you’re on, often from ad networks).
This world is a complex one and I’ve spent very little (but not zero) time in my career directly analyzing data produced by third-party cookies. I’m generally supportive of the direction that Apple is pushing the industry towards, but also as an outsider don’t feel like I understand all of the tectonic shifts playing out here. There are literally trillions of dollars of market cap that are tied up in this trend and thinking through the game theory of who’s-going-to-do-what is probably not that productive of a way to spend your time.
BUT! It’s an important topic to stay on top of given how central cookie data is to much of the work we all do. I found this EFF article to be an opinionated take on some of the movement playing out right now and a good way to catch up.
This is really very cool.
Product analytics is hard, and it’s not super-well-solved by the modern data stack today. I’ve written about this before, but doing product analytics inside of traditional BI products isn’t intuitive and requires more technical expertise than most users have / have time for. There really is a role to play for vertical-specific analytical experiences to play in this use case, and I’m excited about companies like Indicative building on top of the modern data stack and Mixpanel making big moves in this direction as well.
PostHog goes one step further—it’s not just a product analytics tool that plays well with the modern data stack, it’s a fully-open-source approach to the problem. Own the data pipeline as well as the analytical layer on top of it. Get the convenience of their hosting, or go-it-alone with your own deployment. This eliminates the traditional lock-in business model that the industry has had and also opens up the enterprise in a big way.
PostHog is growing very quickly from a launch in 2020 with 3,000 companies already using the product. Definitely follow this.
Truly massive report on the state of AI. There’s a lot in here that—if you’re a close follower of the space—won’t be new to you. What I found particularly interesting were trends outside of the purely technical. For example:
The percentage of international students among new AI PhDs in North America continued to rise in 2019, to 64.3%—a 4.3% increase from 2018. Among foreign graduates, 81.8% stayed in the United States and 8.6% have taken jobs outside the United States.
After surpassing the US in the total number of journal publications several years ago, China now also leads in journal citations; however, the US has consistently (and significantly) more AI conference papers (which are also more heavily cited) than China over the last decade.
In 2019, 65% of graduating North American PhDs in AI went into industry—up from 44.4% in 2010, highlighting the greater role industry has begun to play in AI development.
All of these were net new information. I continue to be very interested in the politics of AI—the nation-vs-nation competition but also the industry-vs-academia-vs-government competition. Information (and information processing) is power.
Things your manager might not know
This fantastic post from Julia Evans has really made the rounds recently. Written from the perspective of a software engineer, it’s nonetheless SO relevant for data professionals.
Being good at telling your manager the right information at the right time and asking for what you need is a superpower. It makes you way more valuable to have on a team (because your manager knows they can trust you to give them the information they need), and it’s more likely that you’ll get what you want (because you’re making it easy for them to do that!).
This skill takes a lot of time to learn but it’s pretty easy to practice. You can take a few minutes to reflect before your 1:1 with your manager and think about what might be important to bring up with them.
I’ve had the experience over the past 5 years of going from running a company with two humans at it to one that now has ~80 humans. There is A LOT that I don’t know at this point, not from my abject lack of competency but simply because I can only receive and process so much information. The ability of folks around me to help me help them by making sure that I have the right information is so important.
Obviously this is a two-way street! Managers need to both create a culture in which this is safe to do and valued/acted upon, but it’s also important for folks managing upwards to empathize with the fundamental impossibility for their managers to know as much as they do about a whole variety of things. If you’ve never yourself been a manager, this post is very valuable for helping build that empathy so that you can get more out of your manager.
Pretty cool: the Reddit team replaced a pure-caching based approach to advertising performance data serving (very inflexible) to a Druid-based OLAP system that could both perform like a cache for pre-aggregations as well as handle analytical queries.
This was interesting for me as I had a conversation last week where “eliminating legacy caching systems” was flagged to me as the next big data pipeline problem to solve. It’s not a problem I’ve personally dealt with but totally understand why this is such a big pain point and why products like Druid and Materialize will be critical here.
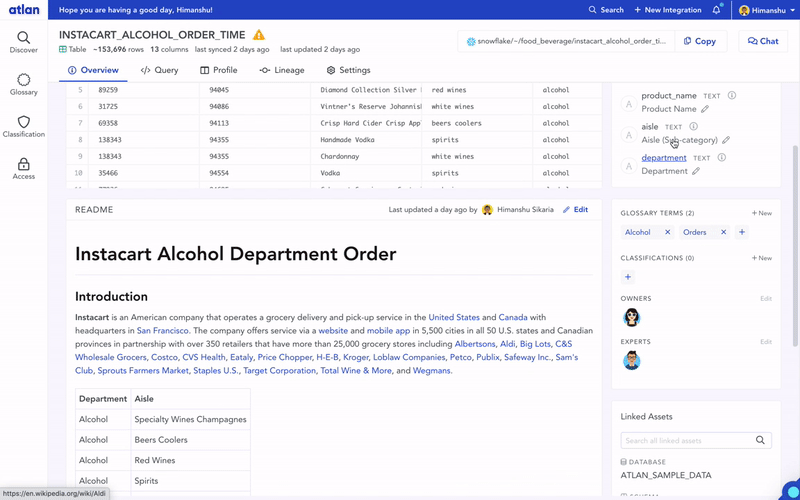
We Failed to Set Up a Data Catalog 3x. Here’s Why.
We thought it would be easy enough to figure this out, but we couldn’t have been more wrong. Here’s the story of how it took 4 attempts and 5 years to finally succeed in implementing a successful data catalog for our team.
Fantastic post. I couldn’t agree more about the characteristics that make for a successful data catalog. Seeing all of the things that didn’t work is as instructive as what did, though, because those are the approaches that I see most companies attempting first.
towardsdatascience.com • Share
Thanks to our sponsor!
dbt: Your Entire Analytics Engineering Workflow
Analytics engineering is the data transformation work that happens between loading data into your warehouse and analyzing it. dbt allows anyone comfortable with SQL to own that workflow.
The internet's most useful data science articles. Curated with ❤️ by Tristan Handy.
If you don't want these updates anymore, please unsubscribe here.
If you were forwarded this newsletter and you like it, you can subscribe here.
Powered by Revue
915 Spring Garden St., Suite 500, Philadelphia, PA 19123