Experimental Guardrails. Capital Markets for Data Products. DALL-E. Dashboard UX. RBAC(!?) [DSR #244]
While not at all related to data, I just have to share: it’s not every day that you get featured in an article titled Anti Tech Bro Startups. Really proud of what our entire team has been able to achieve so far in creating a diverse, inclusive team and culture. There’s more work to do.
- Tristan
❤️ Want to support this project? Forward this email to three friends!
🚀 Forwarded this from a friend? Sign up to the Data Science Roundup here.
This week's best data science articles
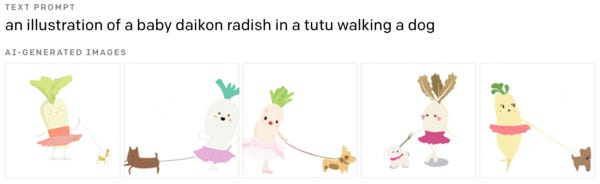
OpenAI: DALL·E: Creating Images from Text
DALL·E is a 12-billion parameter version of GPT-3 trained to generate images from text descriptions, using a dataset of text–image pairs. We’ve found that it has a diverse set of capabilities, including creating anthropomorphized versions of animals and objects, combining unrelated concepts in plausible ways, rendering text, and applying transformations to existing images.
A picture is worth a thousand words. You should absolutely click through to the article to see a ton of fascinating examples of what DALL-E has been able to achieve.
OpenAI is really on a roll.
Databricks is raising over $2 billion in a new funding round, people familiar with the deal said, a massive haul that will give the cloud-based data analytics company a $29 billion post-money valuation. (…) Wall Street heavily anticipates a Databricks IPO sometime this year.
Just another observation to feed into your neural net—the data equities market right now is very hot (also see: Starburst).
Here’s why you should care. I’ve talked before about Carlotta Perez’ framework on how technological change happens: first you get a bubble, then a crash, then a “golden age.” Essentially, the capital rushing in during the bubble gets deployed in building net new technology and infrastructure. Whether or not the financial asset itself is overvalued is kind of besides the point—the dollars still go into funding lots of new R&D.
In this particular case I have absolutely zero opinion on what the “right” valuation is for companies in data today (that question feels boring), but I do get to see very up-close-and-personal where the capital is going. For example, Databricks is investing a tremendous amount in SQL Analytics (and Delta Lake, the open source technology it’s built upon). Delta Lake is awesome.
IMO, this is wind in the sales of every data practitioner today. Capital markets have decided that they want all of us to have better tools.
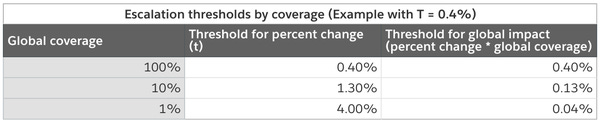
Designing Experimentation Guardrails
Introducing the Experiment Guardrails framework we implemented at Airbnb, which helps us prevent negative impact on key metrics while experimenting at scale.
Super-interesting. I’m always fascinated to hear about the experimentation systems implemented inside of big digital native tech companies because they are so far ahead of what everyone else has access to. This is the first time I’ve read about an automated monitoring system that looks at “guardrail” metrics to potentially end experiments early.
This is just like terminating a clinical trial early if you detect significant side effects of the treatment under study. Such a clearly good idea, but really hard to achieve in practice.
Scaling An ML Team (0–10 People)
You have to think about how to create the right team structure to make ML projects successful. Here’s some lessons drawn from our own experiences of building and scaling ML teams.
The recommendations in this post are not shocking, but I’ve never actually seen a post that presents quite as well-structured, grounded-in-practical-reality advice on building an ML team from scratch. It’s super super different than the roadmap for building an internal analytics team from scratch! What I found particularly interesting is just how cross-functional the ideal 10-person team is.
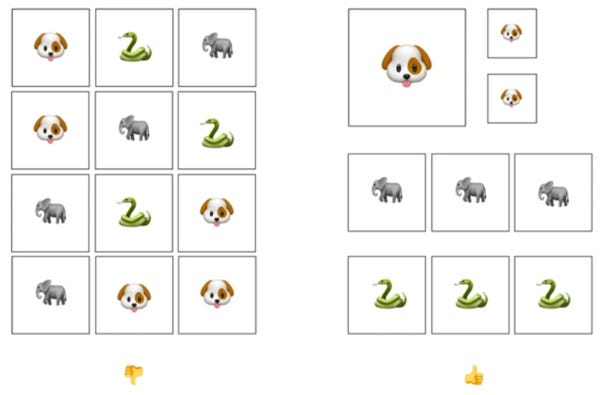
How to Make Dashboards Using a Product Thinking Approach
A step-by-step guide to how you can create dashboards that are user-centered and impact-driven.
If you’re a long-time reader I realize this may be a soapbox that you’re bored of. But I still see so many bad dashboards! This isn’t the fault of a single actor in the ecosystem—the data analyst, dashboard vendors, the dashboard consumer, the structure of data teams and their relationship to the business—it’s all of them working in concert. Each of those four factors pushes dashboards towards being “chart dumps.”
Imagine you needed to write up a summary report on something in text, and your strategy was: open a blank document and just keep typing until you couldn’t think of anything else to say. No editing, no structure, just a pure brain dump. Then hit “publish.” That’s what we still do with dashboards.
Whenever I’ve tried to work with practitioners to improve the UX of their dashboards I’m met with downright consternation that the totality of the experience (rather than just the individual charts themselves) should even be a matter for consideration.
Anyway. Sorry. I’ll get down now. But still, this is a great post if you want to make better dashboard experiences :)
Role-Based Access Control for Data Teams
Code-based RBAC will increase security, lighten cognitive load, and set the foundation for simpler data discovery.
Let me make a prediction: as soon as you read that headline you said to yourself “that sounds boring.” Yeah. I get it. We’re practitioners, we get excited about the insights-generation process and things directly adjacent. But just hear me out.
Access control is a big freaking problem right now. There are so many data systems, and every single one of them has its own abstractions for defining who can see what. As a result, companies of any size are extremely conservative with permissioning, because they have a really hard time asserting that the wrong people don’t have access to sensitive (and often legally-protected) data in this heterogeneous, chaotic environment.
This article points out that software engineering organizations already know how to solve this problem in a declarative, idempotent, automatically-testable way. Which has led to (shocker) more self-sufficient and empowered software engineers.
What would it take to see the data systems we all use every day live up to these same expectations? I actually don’t think it would be so hard. And the upside is huge—in fact, I’m pretty convinced that this is truly on the critical path to true data democratization within medium and large organizations.
A trend I'm excited for this year: DataOps & the Analytical Engineer
~10 years ago DevOps was born. The role of system admins and developers merged. Infrastructure became self-serve
Today the role of data engineers and business analysts are merging. Data is becoming self-serve
Thanks to our sponsors!
dbt: Your Entire Analytics Engineering Workflow
Analytics engineering is the data transformation work that happens between loading data into your warehouse and analyzing it. dbt allows anyone comfortable with SQL to own that workflow.
The internet's most useful data science articles. Curated with ❤️ by Tristan Handy.
If you don't want these updates anymore, please unsubscribe here.
If you were forwarded this newsletter and you like it, you can subscribe here.
Powered by Revue
915 Spring Garden St., Suite 500, Philadelphia, PA 19123