
Five Books. Gender Shades. Deploying Cloud Infrastructure. Top AI Grad Programs. [DSR #128]
My co-founders Drew and Connor will be attending DataEngConf San Francisco next month! As a part of the event festivities, we’re hosting a happy hour with the folks from Mode Analytics and Stitch! We’d love to meet up with you if you’re in town for the event—sign up here.
Enjoy the issue :)
- Tristan
❤️ Want to support us? Forward this email to three friends!
🚀 Forwarded this from a friend? Sign up to the Data Science Roundup here.
The Week's Most Useful Posts
Top 5 Business Books Every Data Scientist Should Read
This list is really damn good: I’ve read every book on it and agree that each have been foundational in building my own mental models. Each book is actually fairly short; you could easily read all five in the next month.
towardsdatascience.com • Share
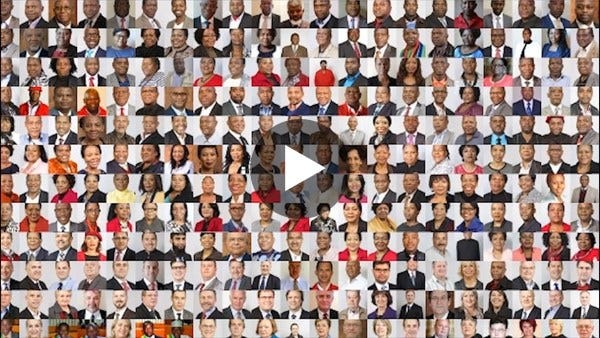
You likely know that image classifiers tend to do a worse job at detecting faces in people of color. But do you know how much worse?
The video above features researcher Joy Buolamwini, head of the Gender Shades project at MIT Media Lab, going over her team’s results. The team systematically studied the accuracy of image classifiers from IBM, Microsoft, and Face++ on different genders and skin tones, and the results were not good.
This matters.
The Machine Learning Reproducibility Crisis
I was recently chatting to a friend whose startup’s machine learning models were so disorganized it was causing serious problems as his team tried to build on each other’s work and share it with clients. Even the original author sometimes couldn’t train the same model and get similar results! He was hoping that I had a solution I could recommend, but I had to admit that I struggle with the same problems in my own work. It’s hard to explain to people who haven’t worked with machine learning, but we’re still back in the dark ages when it comes to tracking changes and rebuilding models from scratch. It’s so bad it sometimes feels like stepping back in time to when we coded without source control.
100% agree. We may have made a lot of progress in the past couple of years deploying ML systems, but the industry is nowhere near a point of process maturity. We still suck at this.
What does it mean to be a Senior Data Scientist?
Peadar Coyle is a Senior Data Scientist. Given that many organizations still struggle with what it means to be a data scientist, what exactly does the “Senior” prefix mean?
The post is a bit of a stream-of-consciousness, but I really think Peadar’s perspective is excellent. Here’s my favorite paragraph:
As a Senior Data Scientist working in a the regulated world of Financial Services – I’ve grown to appreciate that it’s my job to have a working knowledge of GDPR, it’s something we regularly bring up when we discuss the viability of projects, and it’s a ‘risk factor’. It would be immature to just ignore this, and frankly unethical and unprofessional.
“Senior” indicates not just being really good at stats and math and programming—to Peadar it’s more about people skills and ethics and impact.
peadarcoyle.wordpress.com • Share
From Local Machine to Dask Cluster with Terraform
Learn how you can take local code that does grid search with the Scikit-Learn package to a cluster of AWS (EC2) nodes with Terraform.
Data scientists and the data science blogosphere have made a lot of hay out of Docker. And with good reason: Docker is definitely an important tool in the data science tool belt. But I’m always surprised that Terraform doesn’t make more of an appearance in these conversations.
Terraform has become a mainstay of the devops community, and is a part of the infrastructure-as-code trend. With it, you can describe the configuration of a set of cloud resources, run “apply” and your infrastructure (complete with associated containers) is spun up.
We use Terraform internally very heavily; I expect its usage within the data science community to grow. This is an awesome walkthrough of how one data science team used it to solve a real problem.
US News & World Report: Top 20 AI Graduate Programs
US News & World Report just released a brand new ranking category for AI grad programs. This is the first time I’ve seen a list of top AI programs, and I figured it might be useful if any of you are evaluating your options today.
Take it with a grain of salt, obviously: the academic ranking process is certainly imperfect.
Cambridge Analytica Scandal: The Biggest Revelations So Far
The recent Cambridge Analytica news is a case study on what happens when you fail to effectively act as steward of your users’ privacy.
You’re likely faced with decisions every day that could impact the privacy of thousands or millions of users. Are you emailing data files or passwords? Do you have IP whitelists and SSH tunnels to limit access to your infrastructure? What are your data retention policies? Do you restrict access to only need-to-know PII internally?
These decisions are not just for corporate leaders—they’re for every single person with access to PII today.
Jobs!
Data Engineer @ 8fit
Junior Data Analyst @ Simba Sleep
Machine Learning Engineer @ Thrive Commerce
Want to see your job here? Join dbt Slack and post to #jobs!
Thanks to our sponsors!
Fishtown Analytics: Analytics Consulting for Startups
At Fishtown Analytics, we work with venture-funded startups to implement Redshift, Snowflake, Mode Analytics, and Looker. Want advanced analytics without needing to hire an entire data team? Let’s chat.
Stitch: Simple, Powerful ETL Built for Developers
Developers shouldn’t have to write ETL scripts. Consolidate your data in minutes. No API maintenance, scripting, cron jobs, or JSON wrangling required.
The internet's most useful data science articles. Curated with ❤️ by Tristan Handy.
If you don't want these updates anymore, please unsubscribe here.
If you were forwarded this newsletter and you like it, you can subscribe here.
Powered by Revue
915 Spring Garden St., Suite 500, Philadelphia, PA 19123