Four Years of Fishtown! Data @ Shopify. ML on the Shelf. ML Tool Survey. [DSR #229]
For those of you in the US, happy Fourth of July! Definitely one for the history books. Please stay safe š·š·
- Tristan
ā¤ļø Want to support this project? Forward this email to three friends!
š Forwarded this from a friend? Sign up to the Data Science Roundup here.
This week's best data science articles
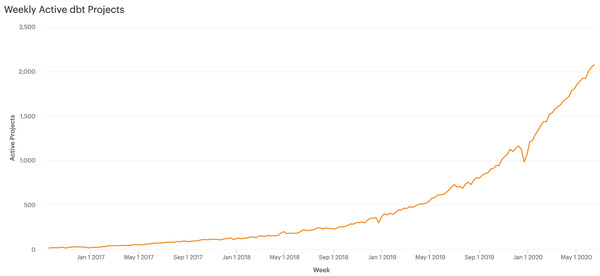
Four Years In: From Misfits to Mainstream
My armchair reflections from year 4 of growing Fishtown Analytics and dbt.
This year has been something else. Year four saw dbt grow from a tool used by forward-thinking early adopters into a burgeoning standard in the modern analytics stack. As of last week, there were more than 2,100 companies that are actively using dbt. Wow. dbt is no longer a niche product and dbt Slack is no longer a community of misfitsā¦weāve gone mainstream, folks.
Towards the end I share my thoughts on where the modern data stack has been and where itās headed. Weāve come a long way in four years, folks.
Itās a great time to be an analyst.
blog.getdbt.com ⢠Share
Shopify's Data Science & Engineering Foundations
Shopifyās Data team uses these foundational approaches to data warehousing and analysis empowering us to deliver the best results for our ecosystem.
This is a Very Good Post. Itās not groundbreakingānone of the individual ideas that it presents are fundamentally new. But it gives such a wonderful picture of how Shopifyās extremely high-functioning data team operates. Hereās a snippet from my favorite section, āDeep Product Understandingā:
At Shopify, we strive to fall in love with the problem, not the tools. Excellence doesnāt come from just looking at the data, but from understanding what it means for our merchants. (ā¦) We truly understand what enable means in the column status of some table.
This seems simple and obvious, but itās all-too-common that those working with the data actually do not have this level of familiarity of the real-world interaction that they are observing in it.
engineering.shopify.com ⢠Share
I gave the business what they asked for and they never used it
This is both amazing and hilarious. I linked to a Kenny Ning post last year about an ML project he did @ Better, and the post got plenty of attention elsewhere. Turns out though, the work got very little usage internally. This is a reflection of what went wrongāwhy didnāt users find the project valuable?
Hereās my favorite section:
We probably didnāt need a fully productionized ML solution to improve our understanding of conversion. For example, consider this much simpler solution: a) Collect a dozen candidate features and fit a model offline. You can do this using a fancy ML library, but logistic regression in Excel works fine too. b) Pick the top 3 most predictive features and sense-check with a domain expert. c) Track those 3 features as KPIs in a line chart.
I think this is often a valuable (although less flashy) approach to data science work. Generate a novel insight using ML but then present the insight using traditional descriptive statistics.
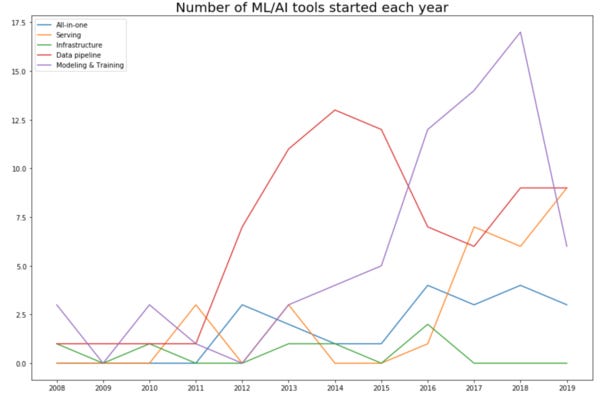
What I learned from looking at 200 machine learning tools
To better understand the landscape of available tools for machine learning production, I decided to look up every AI/ML tool I could find.
Really good postāit pulls ML/AI tooling out of the overall ML/AI āvertical applicationsā. In a given year, the author found that only 7 out of 50 ML/AI startups were focused on building tooling in the space whereas the other 43 were building applications to help businesses solve problems using ML/AI (like better email targeting, etc.)
The whole post is good, but I found the above chart to be particularly interesting. You can clearly see innovation moving up the stackāmoving from data pipeline tools to modeling & training tools to serving infrastructure. This makes complete sense, and is a very solid foundation from which to make predictions about where the industry is headed next.
huyenchip.com ⢠Share
An Opinionated Guide to ML Research
This post is fantastic. It talks about a too-infrequently-discussed topic: problem taste.
Your ability to choose the right problems to work on is even more important than your raw technical skill. This taste in problems is something youāll develop over time by watching which ideas prosper and which ones are forgotten.
and more:
Sometimes, people who are both exceptionally smart and hard-working fail to do great research. In my view, the main reason for this failure is that they work on unimportant problems. When you embark on a research project, you should ask yourself: how large is the potential upside? Will this be a 10% improvement or a 10X improvement? I often see researchers take on projects that seem sensible but could only possibly yield a small improvement to some metric.
Yes!
joschu.net ⢠Share
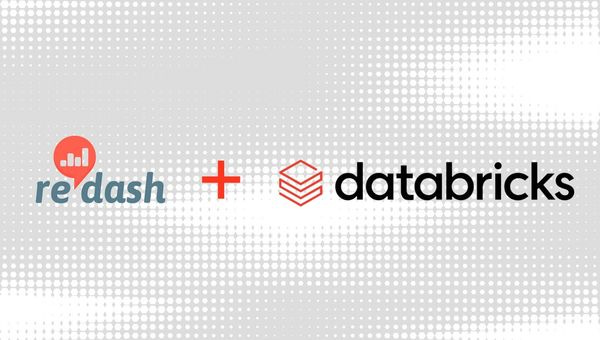
Huh! On one level, I donāt know exactly how much to read into this. Redash, while having a huge open source footprint, never went deep down the commercialization path to my knowledge. So this could be more of a story about personal decisions for the Redash team than anything else.
At the same time, Both Databricks and Snowflake are now massive companies and have ample dry powder to start broadening their solutions. Will they become more acquisitive as their valuations continue to increase into the stratosphere? IMO this almost has to happenāthe huge go-to-market teams that these companies have built exert gravity on them to solution sell and to cross-sell products into existing client relationships.
The competitive dynamics with the hyperscale cloud providers is another forcing function hereāMSFT, AMZN, and GOOG all have broad suites of tools that theyāre selling, so Snowflake and Databricks have to as well. Using this lens, I actually think Redash could stack up well against more mature products like PowerBIāit makes much more modern assumptions about the ecosystem it operates within.
blog.redash.io ⢠Share
Thanks to our sponsors!
dbt: Your Entire Analytics Engineering Workflow
Analytics engineering is the data transformation work that happens between loading data into your warehouse and analyzing it. dbt allows anyone comfortable with SQL to own that workflow.
getdbt.com ⢠Share
Stitch: Simple, Powerful ETL Built for Developers
Developers shouldnāt have to write ETL scripts. Consolidate your data in minutes. No API maintenance, scripting, cron jobs, or JSON wrangling required.
The internet's most useful data science articles. Curated with ā¤ļø by Tristan Handy.
If you don't want these updates anymore, please unsubscribe here.
If you were forwarded this newsletter and you like it, you can subscribe here.
Powered by Revue
915 Spring Garden St., Suite 500, Philadelphia, PA 19123