Gartner BI Hype Cycle. A/B Testing. EXIF. Astrophysicists. Tiny Language Models. [DSR #201]
❤️ Want to support this project? Forward this email to three friends!
🚀 Forwarded this from a friend? Sign up to the Data Science Roundup here.
This week's best data science articles
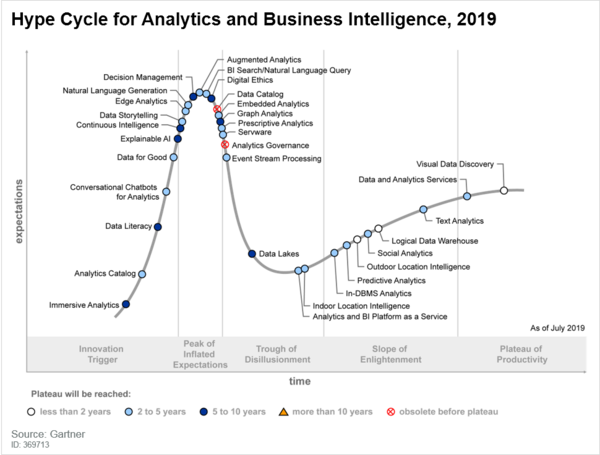
Gartner Reveals Five Major Trends Shaping the Evolution of Analytics and Business Intelligence
I think it’s a mistake to mine these Gartner hype cycle charts for too much—they’re trailing indicators, not leading ones. Forrester and Gartner are not good at predicting the future, just holding up a mirror to the industry and saying “this is what I’m hearing”.
So while I think it’s downright silly that data catalogs will be obsolete before the “plateau of productivity” (there’s an incredible amount of innovation in this space!) I do find it valuable to look through the above chart.
Squarespace | How We Talk About A/B Test Results
A/B tests can help organizations make better decisions, but we often only hear about the “success stories.” What about the A/B tests that don’t have flashy outcomes? Here’s how Squarespace communicates about A/B tests to ensure we learn from all tests.
100% agree with this. Airbnb has written good stuff on this topic before as well—the point of the article is that A/B tests aren’t just opportunities to improve performance, they’re also an important source of insights. Cataloging tests and communicating results to the broader organization is critical to realizing this value.
engineering.squarespace.com • Share
What My Data Science Degree Did Not Teach Me
Three years ago, I graduated from my M.sc in Data Science(…) When I look back, I certainly see that my Master helped immensely in giving me a start. However, there were many relevant points that my academic training did not address. (…) when I entered the field, many difficult or essential aspects of the job were unknown to me.
Worthwhile. The points the author covers won’t be brand new if you’ve followed this newsletter for a while, but she’s dead on.
towardsdatascience.com • Share
The dumb reason your fancy Computer Vision app isn’t working: Exif Orientation
I never would have known this. Straightforward, fascinating, well-written. Read this and file it away.
Tiny AI models could supercharge autocorrect and voice assistants on your phone
Researchers have successfully shrunk a giant language model to use in commercial applications.
You’re likely familiar with the impressive results of language models released in the past year as well as their massive parameter count—I’ve covered these developments here pretty extensively. This article summarizes two papers, one from Huawei and one from Google, that shrunk the behemoth models many times over and yet retained nearly-identical performance. The technique used was interesting:
Both papers use variations of a common compression technique known as knowledge distillation. It involves using the large AI model that you want to shrink (the “teacher”) to train a much smaller model (the “student”) in its image. To do so, you feed the same inputs into both and then tweak the student until its outputs match the teacher’s.
www.technologyreview.com • Share
The Style Maven Astrophysicists of Silicon Valley
This not-at-all-technical piece from Wired covers the migration of academics, particularly from astrophysics, to commercial roles in machine learning.
The thing I think is interesting about this is that I perceive a latent normative judgment here: that it’s “bad” that huge numbers of academics are migrating towards industry and away from pure research.
I don’t really agree with that. I think that there are natural pendulum swings during massive technology cycles. First the emphasis is on research and smart people flock to academia, then the emphasis is on deployment and smart people flock to industry. The tools / methodologies / culture that is being built inside of companies like Netflix and Stitch Fix today are incredibly important to the development of the ML/AI field (much is being done in the open), and these advancements will feed back into the next generation of academic research.
Time is a flat circle.
Thanks to our sponsors!
dbt: Your Entire Analytics Engineering Workflow
Analytics engineering is the data transformation work that happens between loading data into your warehouse and analyzing it. dbt allows anyone comfortable with SQL to own that workflow.
Stitch: Simple, Powerful ETL Built for Developers
Developers shouldn’t have to write ETL scripts. Consolidate your data in minutes. No API maintenance, scripting, cron jobs, or JSON wrangling required.
The internet's most useful data science articles. Curated with ❤️ by Tristan Handy.
If you don't want these updates anymore, please unsubscribe here.
If you were forwarded this newsletter and you like it, you can subscribe here.
Powered by Revue
915 Spring Garden St., Suite 500, Philadelphia, PA 19123