Getting a PhD. Measuring Intelligence. Taking Notes. Event Delivery @ Spotify. [DSR #206]
❤️ Want to support this project? Forward this email to three friends!
🚀 Forwarded this from a friend? Sign up to the Data Science Roundup here.
This week's best data science articles
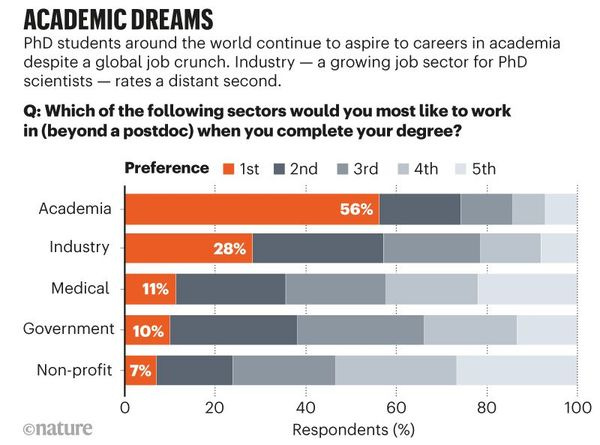
Nature’s survey of more than 6,000 graduate students reveals the turbulent nature of doctoral research.
This has nothing explicitly to do with data, but I believe it explains some of the forces in the job market that are a little bit unusual in our field. All other primary functions of an operating business are staffed with people who have batchelor’s or master’s degrees, but it is not at all uncommon to find a data science team stocked with PhDs. I believe these survey results (and specifically the answer to the above question) point towards the cause: there is a mismatch between career ambitions and job availability within academia. Many PhDs have advanced quantitative skills, and find that data science is a natural (and lucrative) transition from a weak academic job market.
This begs the question: if one’s career is ultimately the point of the degree (which is by no means always true), then wouldn’t it make more sense to get a different degree? PhDs are extremely personally costly.
There is certainly a much larger conversation to be had here, and my point is not to discourage applications to PhD programs. Rather, I think it’s important to have a realistic understanding of the job market and make well-informed life decisions. To be super-clear: you don’t need a PhD to become a great data scientist.
This brand new paper from Keras creator François Chollet tackles some pretty fundamental stuff:
We articulate a new formal definition of intelligence based on Algorithmic Information Theory, describing intelligence as skill-acquisition efficiency and highlighting the concepts of scope, generalization difficulty, priors, and experience. Using this definition, we propose a set of guidelines for what a general AI benchmark should look like.
Finally, we present a benchmark closely following these guidelines, the Abstraction and Reasoning Corpus (ARC), built upon an explicit set of priors designed to be as close as possible to innate human priors. We argue that ARC can be used to measure a human-like form of general fluid intelligence and that it enables fair general intelligence comparisons between AI systems and humans.
AI generalizability is a topic that needs meaningful definitions and measurements if we are to make real progress on it, and this is a big swing.
Can somebody volunteer to take notes?
The meeting is about to begin and the moderator asks “Can somebody take notes?”. And then nobody says a word. Should you volunteer?
Tanya Reilly (of Being Glue fame) wrote a great post on why notes matter, who should take them, and when you should volunteer. I agree with 100% of everything she says and want to be better myself about taking notes. I used to do this a lot in the earlier stages of my career and it made a surprisingly large impact; I should recover the practice.
Important, short read.
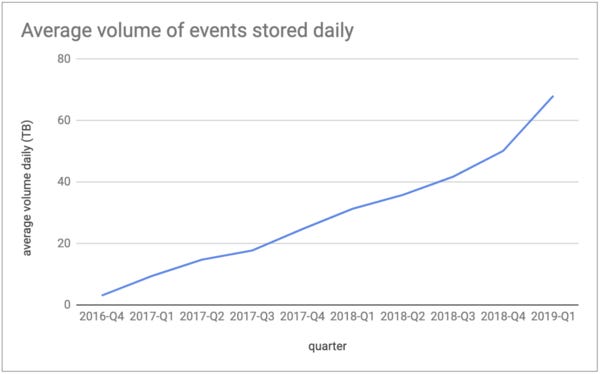
Spotify’s Event Delivery – Life in the Cloud
A blog post describing how Spotify’s event delivery has evolved after being on the Google Cloud Platform for two and a half years.
Heh. Wow. 65TB / day is a lot. Roughly 24PB / year at that pace, and the rate is continuing to grow.
I was very interested in Spotify’s decision to heavily use GCP services—not just compute engine instances and GCS buckets, but services like PubSub, Bigquery, and many more—to build their pipeline on back in 2016. This decision was notably different from the decisions made at other consumer unicorns like Airbnb / Pinterest / Uber: those companies all use open source tools like Kafka and Presto as the foundation for their pipelines.
That’s why this post is interesting: it’s notably different than similar posts written by these other similar companies. Very useful perspective.
How to Find Consulting Clients
Written from the perspective of a data scientist just starting to think about taking the plunge into the consulting world. As someone who has gone through this process, this is actually the best advice I’ve ever read on the topic. It’s quite straightforward, but invaluable if you haven’t done it before.
My two cents: if you’ve ever considered going it alone as a consultant, I think it’s a great choice. It’s certainly not for everyone, but the rewards are really significant and the downside is very limited. And there is plenty of data work to be done right now—if you have strong skills and are willing to put in the work, you’ll very likely be able to make a go of it.
Thanks to our sponsors!
dbt: Your Entire Analytics Engineering Workflow
Analytics engineering is the data transformation work that happens between loading data into your warehouse and analyzing it. dbt allows anyone comfortable with SQL to own that workflow.
Stitch: Simple, Powerful ETL Built for Developers
Developers shouldn’t have to write ETL scripts. Consolidate your data in minutes. No API maintenance, scripting, cron jobs, or JSON wrangling required.
The internet's most useful data science articles. Curated with ❤️ by Tristan Handy.
If you don't want these updates anymore, please unsubscribe here.
If you were forwarded this newsletter and you like it, you can subscribe here.
Powered by Revue
915 Spring Garden St., Suite 500, Philadelphia, PA 19123