Google Duplex. Data Privacy in Analytics. Team Names @ Lyft. Scientific Debt [DSR #135]
❤️ Want to support us? Forward this email to three friends!
🚀 Forwarded this from a friend? Sign up to the Data Science Roundup here.
The Week's Most Useful Posts
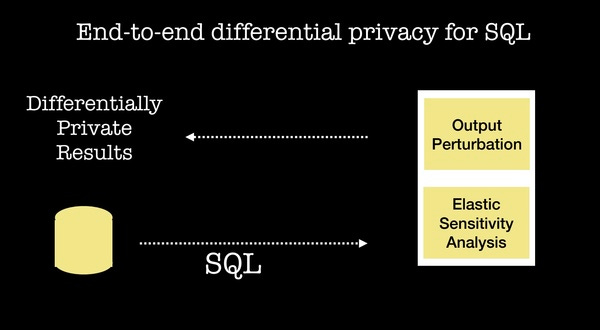
How to build analytic products in an age when data privacy has become critical
The industry has historically wanted to spend as little time thinking about data security and privacy as possible. The more time one spends thinking about security and privacy, the less time is spent actually pursuing insights!
This mentality is changing, though, for two reasons:
Data is more integrated than ever, which significantly increases risk.
Regulation (GDPR, specifically) is creating a set of accepted practices where there previously were none.
Often, posts on security and privacy are boring and high-level, which is why I don’t often link to them. This one, however, is excellent. It introduces a ton of concepts, all with links to explore in greater depth. It talks about work being done at universities and in industry. Highly recommended.
Biggest takeaway:
At its core, privacy by design calls for the inclusion of data protection from the onset of the designing of systems, rather than as an addition.
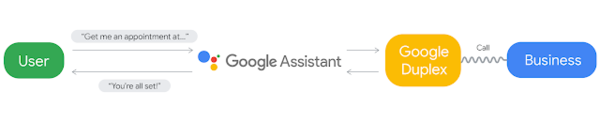
Google Duplex: An AI System for Accomplishing Real-World Tasks via Phone
It’s big tech co conference time, and the announcement that’s generating the most buzz is Google’s Duplex. If you haven’t heard of it, take 4:12 and watch Sundar give the demo (below).
The linked post goes into depth on the product and tech. It’s well worth a read. I’m quite impressed by how natural the interactions feel; I don’t think I could tell I was talking to a robot, which presents a real moral question: is it ok for machines to impersonate humans? Must such machines declare that they are machines? There’s a great summary of the conversations currently occurring on this topic here.
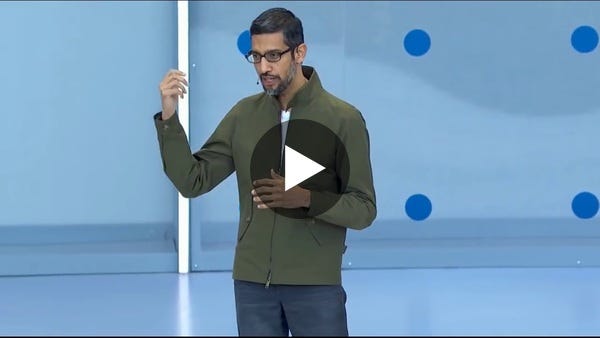
Google Duplex Demo from Google IO 2018 - YouTube
You’re probably familiar with technical debt in software engineering. David Robinson (one of my favorite data science writers), extends this concept to scientific debt:
…I realized that data scientists have a rough equivalent to this concept: “scientific debt.” Scientific debt is when a team takes shortcuts in data analysis, experimental practices, and monitoring that could have long-term negative consequences.
This post goes deep into what scientific debt is, how to recognize it, and the impacts on your organization.
At Lyft, we’re rebranding our Data Analyst function as Data Scientist, and our Data Scientist function as Research Scientist.
As the industry evolves, there is still plenty of debate of what exactly it means to be a data scientist. At the end of the day, what actually matters is consensus: names mean what we all agree that they mean. In this post, Lyft describes why they’re changing their titles, and it’s all around perceptions and the hiring process:
We expect this change to result in higher-precision (thus more efficient) hiring funnels for both groups.
This seems reasonable to me. Even if two jobs are identical in responsibilities, the difference in titles has come to signify something important (salary bands!).
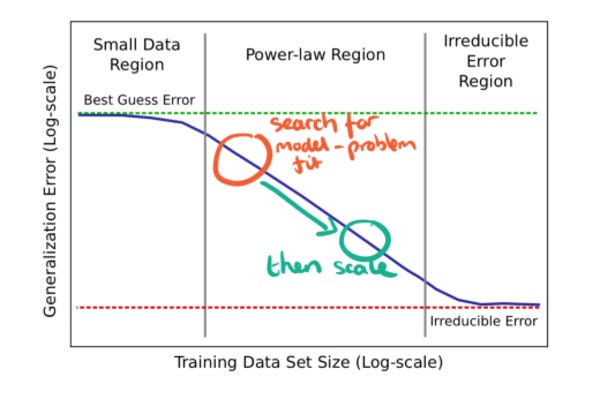
Deep Learning Scaling is Predictable, Empirically
Deep learning performance scales in three specific ways:
We can search for improved model architectures.
We can scale computation.
We can create larger training data sets
This (very accessible!) summary of a recent paper takes a look at the empirical results of how different deep learning has scaled in different domains. It shows that there are consistent scaling properties across different problem domains, leading to the conclusion that we can make predictions about how future scaling will occur.
Who Is Going To Make Money In AI?
We are in the midst of a gold rush in AI. But who will reap the economic benefits? The mass of startups who are all gold panning? The corporates who have massive gold mining operations? The technology giants who are supplying the picks and shovels? And which nations have the richest seams of gold?
This post got a bunch of attention this past week. Good high-level outlook and overall interesting read.
towardsdatascience.com • Share
Thanks to our sponsors!
Fishtown Analytics: Analytics Consulting for Startups
At Fishtown Analytics, we work with venture-funded startups to implement Redshift, Snowflake, Mode Analytics, and Looker. Want advanced analytics without needing to hire an entire data team? Let’s chat.
Stitch: Simple, Powerful ETL Built for Developers
Developers shouldn’t have to write ETL scripts. Consolidate your data in minutes. No API maintenance, scripting, cron jobs, or JSON wrangling required.
The internet's most useful data science articles. Curated with ❤️ by Tristan Handy.
If you don't want these updates anymore, please unsubscribe here.
If you were forwarded this newsletter and you like it, you can subscribe here.
Powered by Revue
915 Spring Garden St., Suite 500, Philadelphia, PA 19123