GPT-3 in the Wild. Dashboards are Dead. Momentum on Streaming. Managing Cost @ Netflix, PII @ Square. [DSR #231]
There’s a lot going on in data right now! Usually the summer is quiet, but this is probably my favorite issue in months.
Hope you and yours are healthy in the midst of this crazy year. If you’re anything like me, this was the best news you’ve had all year. 🤞🤞🤞🤞🤞
- Tristan
❤️ Want to support this project? Forward this email to three friends!
🚀 Forwarded this from a friend? Sign up to the Data Science Roundup here.
On GPT-3
The technology twitterverse is abuzz with GPT-3 experiments. Even having high-level understanding of the model, I’ve been taken aback at some of the things that people have been able to do with it. I’m not at all surprised that it’s been able to do a very good job of generating prose, but writing syntactically correct HTML and SQL that accomplishes a user’s natural language intent? That…was unexpected.
The below two posts are the best overviews that I’ve seen, and I want to include them here because if you haven’t familiarized yourself with this topic I think it’s important that you do. This feels important. Even with the current (obviously imperfect) version, there will be real production use cases and plenty of fascinating and unexpected downstream results.
I highly recommend spending some time diving into this, and these are two good starting points.
GPT-3 is essentially a context-based generative AI. What this means is that when the AI is given some sort of context, it then tries to fill in the rest. If you give it the first half of a script, for example, it will continue the script. Give it the first half of an essay, it will generate the rest of the essay.
Analysis of GPT-3 and its implication for the future
…let’s have a look at what GPT-3 is, what it is capable off and what not, and why it is important to understand it and its implications.
This week's best data science articles
Shared Arrangements: practical inter-query sharing for streaming dataflows
From the author of Materialize.io:
Current systems for data-parallel, incremental processing and view maintenance over high-rate streams isolate the execution of independent queries. This creates unwanted redundancy and overhead in the presence of concurrent incrementally maintained queries: each query must independently maintain the same indexed state over the same input streams, and new queries must build this state from scratch before they can begin to emit their first results.
This paper introduces shared arrangements: indexed views of maintained state that allow concurrent queries to reuse the same in memory state without compromising data-parallel performance and scaling. We implement shared arrangements in a modern stream processor and show order-of-magnitude improvements in query response time and resource consumption for incremental, interactive queries against high-throughput streams, while also significantly improving performance in other domains including business analytics, graph processing, and program analysis.
This is so cool. We’re actively seeing real research and progress on stream processing systems that present like databases! I am starting to be bullish on this being a very big deal within the next 5 years.
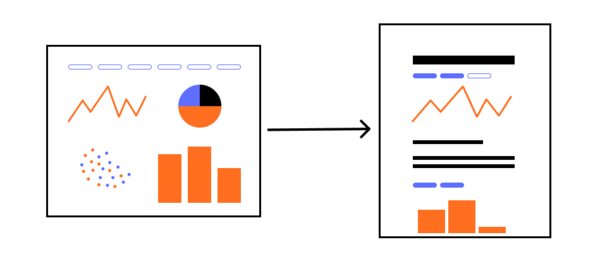
There is a lot a lot to like about this post. The thing I like best is that someone is actually putting real effort into alternatives to the dashboard-based BI status quo. I’m not saying that dashboards aren’t good and valuable, but I have personally been feeling some of the pains that the author points out in the post.
That said, I’m not sure that I 100% agree with notebooks as The Solution. Notebooks are awesome! But they’ve also been around for…a while now. Why is 2020 the year where all dashboards migrate to notebook form?
The thing I do 100% agree with in the author’s solution section is that “data is going portrait mode.” My personal belief is, though, that this portrait mode is going to look a lot more like Reddit—posts with constrained content types, specific topic areas that can be subscribed to, social features… You’ll train the feed to surface the data from your org that you most care about.
I realize that might seem like a bit of a big jump, but it’s something I’ve been noodling on a lot recently. Want to tell me I’m crazy? Hit reply, I’d love to hear it :)
towardsdatascience.com • Share
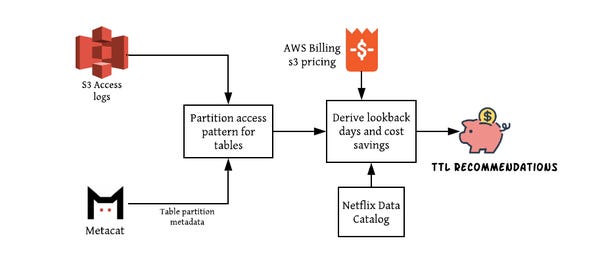
Making Netflix’s Data Infrastructure Cost-Effective
Wowow! This is really cool. Netflix has gone very deep on making sure that they have control over their data-related costs, not at all an easy thing given the heterogeneity of their data ecosystem. This is a topic that has come up a bunch of times recently and generally the feedback I’ve heard from practitioners is “we’re not doing a very good job of that.” So it’s interesting to see what “good” looks like.
What’s probably the most interesting about this post is the topic of time-to-live. Netflix’s infrastructure can automatically provide recommendations for how long to keep historical data in given collections based on partition query access patterns (see the diagram above). As we all start generating and analyzing more and more data, this type of system is going to become an absolute requirement.
Using Amundsen to Support User Privacy via Metadata Collection at Square
When I started at Square, one of our primary privacy challenges was that we needed to scale and automate insights into what data we stored, collected, and processed. We relied mostly on manual work by individual teams to understand our data. As a company with hundreds of services, each with their own database and workflow, we knew that building some type of automated tooling was key to continuing to protect our users’ privacy at scale.
The author details Square’s investments in automatically detecting PII and scoping user’s access to it appropriately. This is a really non-trivial problem, and I really don’t believe many organizations are doing it well today. It’s a big issue, too—without being able to do this well, your only two options are:
fail to protect user PII (presents huge organizational and consumer risk)
be highly restrictive in what data access you hand out (significantly limits culture of self-service)
developer.squareup.com • Share
Opinionated analysis development
This might feel a little obvious to you if you’re in the trenches doing real data work, but what’s fascinating to me is that it’s a point that even needs to be made! Hilary Parker of Stitchfix is telling academic data programs that they need to actually teach students how to construct an analysis:
Traditionally, statistical training has focused primarily on mathematical derivations and proofs of statistical tests. The process of developing the technical artifact—that is, the paper, dashboard, or other deliverable—is much less frequently taught, presumably because of an aversion to cookbookery or prescribing specific software choices. In this paper I argue that it’s critical to teach analysts how to go about developing an analysis in order to maximize the probability that their analysis is reproducible, accurate, and collaborative. A critical component of this is adopting a blameless postmortem culture. By encouraging the use of and fluency in tooling that implements these opinions, as well as a blameless way of correcting course as analysts encounter errors, we as a community can foster the growth of processes that fail the practitioners as infrequently as possible.
Teaching someone how to derive the mean of the binomial distribution but not how to conduct a reproducible analysis and check it into source control feels to me like teaching Newtonian physics without ever covering the scientific method. Process turns out to be pretty damn important.
Thanks to our sponsors!
dbt: Your Entire Analytics Engineering Workflow
Analytics engineering is the data transformation work that happens between loading data into your warehouse and analyzing it. dbt allows anyone comfortable with SQL to own that workflow.
Stitch: Simple, Powerful ETL Built for Developers
Developers shouldn’t have to write ETL scripts. Consolidate your data in minutes. No API maintenance, scripting, cron jobs, or JSON wrangling required.
The internet's most useful data science articles. Curated with ❤️ by Tristan Handy.
If you don't want these updates anymore, please unsubscribe here.
If you were forwarded this newsletter and you like it, you can subscribe here.
Powered by Revue
915 Spring Garden St., Suite 500, Philadelphia, PA 19123