Growing the GitLab Data Team. Sketching. Long-Range Memory. Decision-making. [DSR #218]
❤️ Want to support this project? Forward this email to three friends!
🚀 Forwarded this from a friend? Sign up to the Data Science Roundup here.
This week's best data science articles
Lessons learned managing the GitLab Data team
What follows are a few lessons I learned (and relearned!) in my 1 year stint as the manager of the Data team. (…) While I was Manager, GitLab grew in size by ~300%. Having only worked previously at established companies and at a very small startup, I was not prepared for this level of growth and the strain it would put on our resources.
Wow—what a fantastic post. There are not a lot of people who have gone on the ride that Taylor Murphy has gone on, scaling a data org as their company went through hypergrowth. Taylor’s takeaways are spot on, and demonstrate not just what a great job he did in his 1-year stint on the role, but how the larger organizational culture at Gitlab made it possible. It’s not often that “blitzscaling” happens while keeping quality and culture, and it’s hard.
Kudos Taylor, and congrats on switching back to the IC track :D
DeepMind: A new model and dataset for long-range memory
Throughout our lives, we build up memories that are retained over a diverse array of timescales, from minutes to months to years to decades. When reading a book, we can recall characters who were introduced many chapters ago, or in an earlier book in a series, and reason about their motivations and likely actions in the current context. We can even put the book down during a busy week, and pick up from where we left off without forgetting the plotline.
We do not achieve such feats by storing every detail of sensory input we receive about the world throughout our lifetimes. Our brains select, filter, and integrate input stimuli based on factors of relevance, surprise, perceived danger, and repetition. In other words, we compress lifelong experience to a set of salient memories which help us understand the past, and better anticipate the future. A major goal of AI researchers is discovering ways of implementing such abilities in computational systems and benchmarks which require complex reasoning over long time-spans.
Memory is an extremely deep area of research, perhaps one of the deepest in all of AI. DeepMind’s announcement here of their Compressive Transformer architecture is an interesting step along that path. Good overview of the topic and a digestible review of their research.
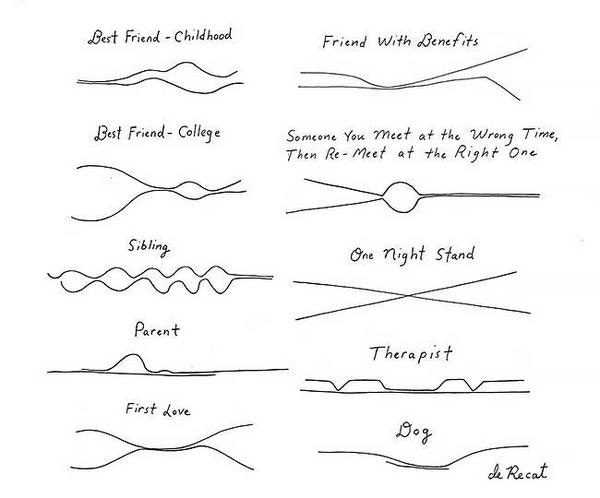
A minimalist drawing that represents closeness over time.
This is an unusual thing for me to post, but it sparked a thought process that I wanted to share. One of the forms of data visualization that used to be easy but now is hard is just…sketching. When most of our work was done on paper, sketching was the easiest possible way to illustrate a point using the techniques of data visualization; it was easier to sketch some approximation of a dataset than to faithfully represent the real thing. At this point, that has flipped: casual representations meant to make a point but not exactly represent reality are now significantly harder to make than mapping 1,000 points faithfully in an XY plane.
That’s a problem. If you ever read Stratechery, you know just how much value Ben’s sketches add to his prose: images are just more information-dense than writing. I use the Paper app on my iPad all the time for this type of work (very much inspired by Ben) but I don’t see many others doing this. If you feel like the image above is an effective means of communication, then you probably should bring this visual form back into your repertoire.
A technique for making difficult decisions—formed in my time as a Google and Facebook exec, and widely deployed at Square.
Another unusual post: this isn’t specifically about data, it’s about decision-making. Often, though, data scientists and analysts are asked not just to bring data to the table, but to be experts on the decision-making process. It is quite possible to make bad decisions that are based on solid data if you don’t have a good process.
This is the process used at Square to this day to organize their decision-making for their most critical decisions.
An Opinionated Guide to ML Research
In this essay, I provide some advice to up-and-coming researchers in machine learning (ML), based on my experience doing research and advising others. The advice covers how to choose problems and organize your time.
Thoughtful advice from an experienced practitioner.
Multi-Channel Marketing Attribution using Segment, Google BigQuery, dbt and Looker
I’ve had this conversation so many times:
What you really want is attribution and conversion reporting thats independent of the networks themselves, uses a multi-touch attribution model that considers all the touch-points in the conversion journey and is under your control, not the advertisers’.
Even moderately effective marketing attribution remains extremely hard for most organizations to achieve. This writeup is by Mark Rittman, and it closely mirrors how we’ve been doing solving this problem for years. I’m so happy that someone took the time to write out a great walkthrough.
Thanks to our sponsors!
dbt: Your Entire Analytics Engineering Workflow
Analytics engineering is the data transformation work that happens between loading data into your warehouse and analyzing it. dbt allows anyone comfortable with SQL to own that workflow.
Stitch: Simple, Powerful ETL Built for Developers
Developers shouldn’t have to write ETL scripts. Consolidate your data in minutes. No API maintenance, scripting, cron jobs, or JSON wrangling required.
The internet's most useful data science articles. Curated with ❤️ by Tristan Handy.
If you don't want these updates anymore, please unsubscribe here.
If you were forwarded this newsletter and you like it, you can subscribe here.
Powered by Revue
915 Spring Garden St., Suite 500, Philadelphia, PA 19123