How is the state of analytics engineering?
The 2024 State of Analytics Engineering is in. Let's get into it.
dbt Labs recently published the 2024 State of Analytics Engineering. If you haven’t seen it, we interviewed 400+ data practitioners and leaders about their work, their investments, their challenges…there was also a question about pie charts, because we couldn’t help it.
Also, if you really haven’t seen the report, check it out.
For a report like this, it’s always best to get out of the way and to let folks speak for themselves. We kept the analysis light. But there are a few initial observations that have bubbled up since publishing, because the 2024 State of Analytics Engineering went on a bit of a tour.
First there was a meetup in NYC hosted at Materialize’s office featuring Adam Stone from Brooklyn Data Co., and then a webinar featuring Ian Macomber from Ramp. We’ve started to hear how folks are responding to the report's findings and what resonates with them. It’s the whole point of a project like this—capturing the experiences of data teams.
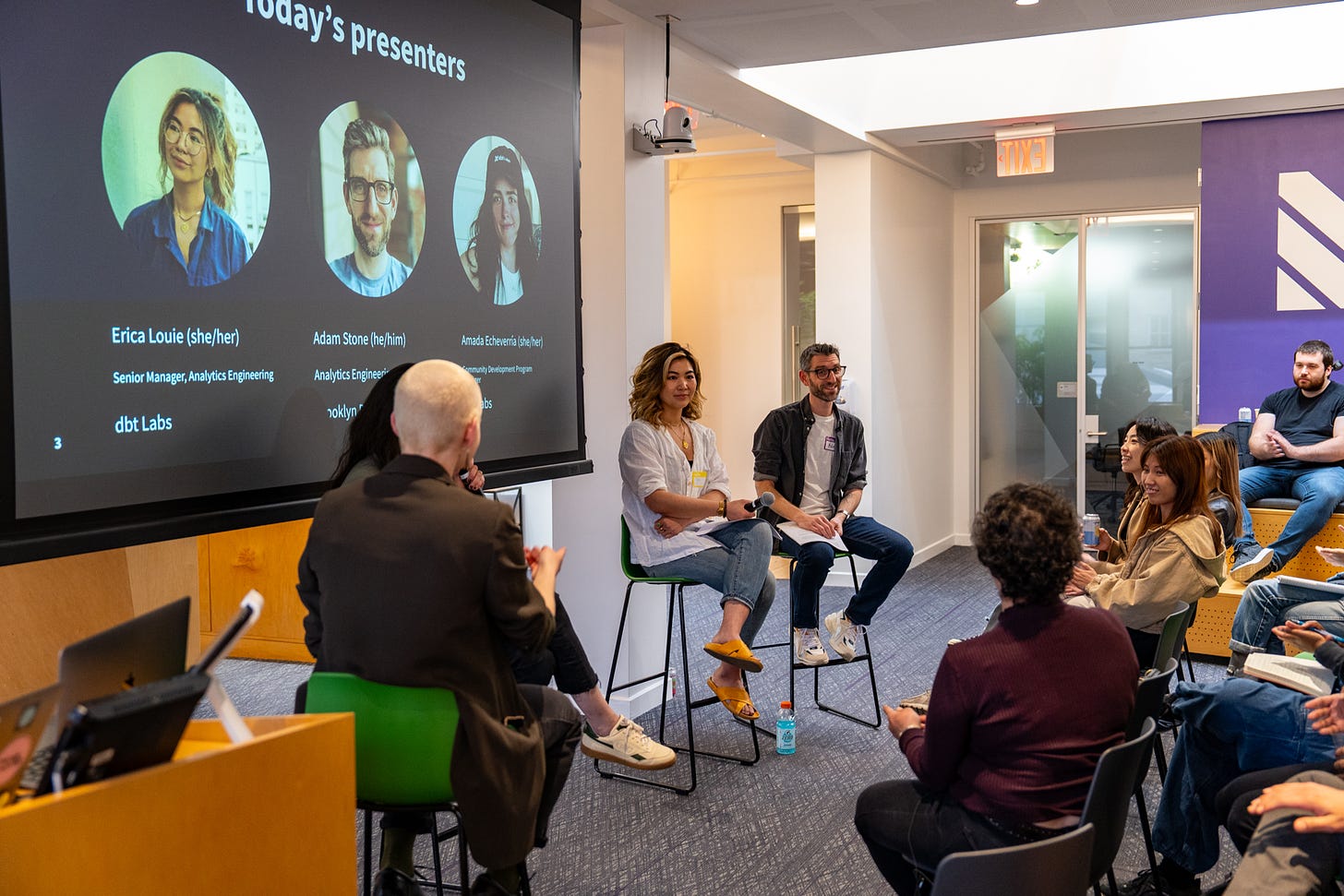
The challenges of data teams today aren't the same as yesterday (if yesterday was 10 years ago)
The chart that has generated the most conversation so far is on what data teams find most challenging while preparing data for analysis.
Poor data quality wins(?) the day. It’s not entirely surprising that data practitioners need to be concerned about poor data quality. We did note, however, that it has escalated—57% of respondents identified poor data quality as a chief concern, which is up from 41% last year.
But look at the right side of the above chart. Of all the challenges in the daily life of data teams, building data transformations and constraints on compute resources is near the bottom of concerns for data teams. A few years ago, those were the biggest problems in data. Today? Not so much. It’s pretty wild how much the landscape can change in a few years.
When Tristan published the dbt viewpoint in 2016, it raised a set of philosophies about how analytics work should be done. That analytics work is collaborative. That you should write your analytics projects using version control. You should test. You should make it modular. You should use the insights from software engineering and bring software engineering best practices into your analytics code.
This was an unusual viewpoint at the time! This was not the way that data teams operated. Data teams were doing a lot of manual work to pull one-off reports. Today? Those original dbt viewpoints now are just the way data gets done.
Or as Adam from Brooklyn Data Co. put it at the dbt Meetup: “In this industry, there are some problems that never really get solved, and others that do. And it's nice to live through a period where you actually are able to solve a big problem.”
But that doesn’t mean there’s not work to do. The modern data stack—a term I’m never allowed to use again—brought mature data workflows; and mature data workflows require mature reliability and organizational structure.
Mahdi Karabiben, a staff engineer at Zendesk, said it well on LinkedIn:
Today, the biggest two challenges that data teams face (poor data quality and ambiguous ownership) are mostly related to people and processes rather than technical complexity or the data platform itself. This is a big shift from the struggles of the pre-Modern-Data-Stack world.
As someone who started working on data platforms during the Hadoop era, it's inspiring to see the industry move past spending endless engineering resources on maintaining complex data infrastructure that had a terrible return on investment.
In a way, this is a direct acknowledgment of the Modern Data Stack’s role in solving one of the Hadoop era’s biggest problems: today’s data platform (mostly) just works. Gone are the days of wasting expensive engineering time on building and maintaining the platform.
The Modern Data Stack may be dead, but for all its flaws, it solved the data platform’s technical hurdles. Now it’s time to solve the business ones.
What remains is what comes next for that output from data teams. As Ian from Ramp says in the State of Analytics Engineering webinar (it’s really worth watching), “A lot of the challenges now for us largely revolve around this truism, which is if you do something that is valuable and appears in a BI tool every 24 hours, [your organization] then asks for three things, which are: 1) I want to put this somewhere else, 2) I want to operationalize, and 3) I want it faster.”
And when data teams are asked to integrate with more tools and systems, these data teams increasingly feel they do not have 100% ownership over their surface areas. This starts to point to why ambiguous data ownership weighs so heavily on data teams.
Because again, data teams more or less have a handle on data transformation, but as the report notes, maintaining datasets is still how data teams are spending most of their time. As teams bring data close to the metal of organizational processes, how are data teams making sure they are enabling others to correctly own their data?
On that note of data teams enabling others:
Sara Levy of Euno shared the following on LinkedIn inspired by the above chart:
We want our business users to think of the data team as a partner and enabler, helping them access the data they need, not as an obstacle or gatekeeper to be worked around.
In other words, free analysts from the “philosophy” of data and focus more on performing the actual analyses.
The thing is…
There are only so many analytics engineers and hours in a day to keep data models up to date and consistent. Striking a balance means giving autonomy to analysts while ensuring a level of control and visibility for the central data teams.
And then AI very quickly, because it’s 2024
If you listened to the Analytics Engineering Podcast, you know Tristan’s last question for each guest has always been, “What do you hope to be true in the data industry in 10 years?” Thanks to the pace of change we’re seeing, Tristan recently switched it to, “What do you hope to be true in the data industry in 5 years?” Based on the above chart, he may need to revise that again to, “What do you hope to be true in the data industry next year?”
In the webinar, Ian lays out an example of where Ramp has seen the most value from generative AI: Taking unstructured images and PDFs, being opinionated about what the structure looks like, converting it to semi-structured data, then uploading it into a world where they can run SQL on top of it.
There’s another possible use case for AI in analytics engineering—actually building your projects, writing your code, testing your code, etc.
In reality, individual contributors might be spending a lot less time thinking about AI than their leaders are; data practitioners are trying to get their existing work done.
But in the webinar,
notes there’s one particular aspect of generative AI that is of interest to him, which is taking data work that you've already done, and using AI to help spread it across your organization. As Jason says:One of my favorite quotes ever about dbt was Tristan told me back in the very early days: ‘dbt helps you solve hard problems once.’ And I think that there are some ways that AI can take the hard problems that we have solved once and make them more usable and distributed.
Here’s to solving more and more hard problems once.
Additionally, Jason and his DX team have had a lot of fun using dbt Semantic Layer to answer natural language questions on top of a complex enterprise data source, inspired by Juan Sequeda and his colleagues at data.world’s benchmark for natural language questions against databases.
But getting there still requires understanding the data really well. In the webinar, Jason shares how his team resolved issues in the project by adding appropriate descriptions to the data so that the AI system could understand how to apply filters and apply the logic needed for the project.
As Jason notes, the role of the analytics engineer in a world where we’re using AI systems is getting a deep understanding of the data.
Because at the end of the day—analytics engineering is about bridging the gap between data and human systems. It’s about working to increase our understanding of our organizations and the world around us. We’ve been lucky enough to see progress on some of the challenges we’re tackling and we’ve got a whole lot more problems to work on together.
And where to next? Let us know what you would like to see included in next year’s report.
This newsletter is sponsored by dbt Labs. Discover why more than 30,000 companies use dbt to accelerate their data development.
Great post and thanks for sharing this crucial and vital issues and solutions including using Ai to help fix it. People and Process in enterprise both internal and external including customers can influence data fidelity and services. Data connects entire ecosystem and since we have built next generation technology on Ai which relies on data mau need to change with human like judgment reasoning and collaborative approaches including evolutionary solutions similar to life forms on earth.