ML's Jet Age. 100 Billion Events / Day. Qualitative Research. Evaluating your Models. [DSR #133]
❤️ Want to support us? Forward this email to three friends!
🚀 Forwarded this from a friend? Sign up to the Data Science Roundup here.
The Week's Most Useful Posts
Toward the Jet Age of Machine Learning
Great post. Please do read the whole thing, but you can get a lot just from these three quote pulls:
Machine learning today resembles the dawn of aviation. In 1903, dramatic flights by the Wright brothers ushered in the Pioneer Age of aviation, and within a decade, there was widespread belief that powered flight would revolutionize transportation and society more generally. Machine learning (ML) today is also rapidly advancing.
However, this excitement should also be met with caution. For all the enthusiasm that the Wright brothers generated, nearly half a century would pass before widespread commercial aviation finally became a reality.
…we needed to invent aeronautical engineering before we could transform the aviation industry.
The three main challenges for ML engineering? Efficiency, Automation, and Safety.
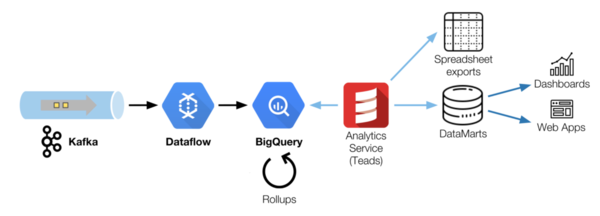
Give Meaning to 100 Billion Events Per Day
Data scientists all seem to agree that a large majority of the work involved in doing data science well is gathering, cleaning, and making raw data available. Yet posts that describe how real companies are solving these problems in production are still quite rare. This one is a gem.
In this article, we describe how we orchestrate Kafka, Dataflow and BigQuery together to ingest and transform a large stream of events.
The post discusses the massive effort that the team at Teads went through to solve their large-scale pipeline problem to get event data into BigQuery efficiently. There were quite a few hiccups along the way, but in the end the Google Cloud stack served them well.
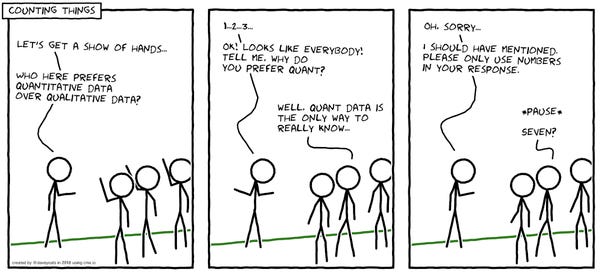
How Qualitative Methods Support Better Data Science
Learn how qualitative methods can help data scientists stay in touch with end users and build better models.
This is a truly under-discussed topic. Web designers had to learn this lesson over a decade or so, and data scientists are in the very early stages of learning it as well: qualitative research is both critical and hard. Just recommending that data scientists “talk to users” isn’t enough.
This is a great post in that it points out the importance of qualitative research methods, but my favorite part of it is the list of resources at the end. Really foundational stuff.
Lessons from My First Two Years of AI Research
A friend of mine who is about to start a career in artificial intelligence research recently asked what I wish I had known when I started two years ago. Below are some lessons I have learned so far. They range from general life lessons to relatively specific tricks of the AI trade. I hope others find them useful.
This is advice I haven’t seen written up anywhere else. Very good, very practical advice focused specifically on AI researchers.
5 Reasons “Logistic Regression” should be the first thing you learn when becoming a Data Scientist
I love that this author provided his own TL;DR:
Learn Logistic Regression first to become familiar with the pipeline and not being overwhelmed with fancy algorithms.
Completely agree with his point: the pipeline is foundational. Understand that first, then go deeper on algorithms.
towardsdatascience.com • Share
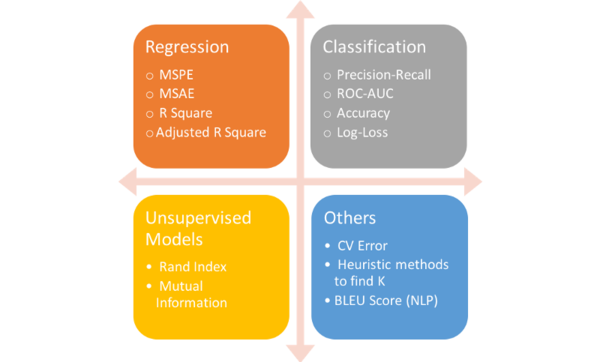
Choosing the Right Metric for Evaluating Machine Learning Models
Even if data prep and feature engineering are the most time consuming parts of most data science projects, model evaluation is the easiest to f#$! up. This is a great post on the metrics you should be using to evaluate your model performance. This is an under-appreciated topic, and one where failing to understand the fundamentals can lead to significant (and costly) missteps.
7 R Data Science Influencers to Follow
Good list! These are names you should be (or become!) familiar with.
blog.modeanalytics.com • Share
Thanks to our sponsors!
Fishtown Analytics: Analytics Consulting for Startups
At Fishtown Analytics, we work with venture-funded startups to implement Redshift, Snowflake, Mode Analytics, and Looker. Want advanced analytics without needing to hire an entire data team? Let’s chat.
Stitch: Simple, Powerful ETL Built for Developers
Developers shouldn’t have to write ETL scripts. Consolidate your data in minutes. No API maintenance, scripting, cron jobs, or JSON wrangling required.
The internet's most useful data science articles. Curated with ❤️ by Tristan Handy.
If you don't want these updates anymore, please unsubscribe here.
If you were forwarded this newsletter and you like it, you can subscribe here.
Powered by Revue
915 Spring Garden St., Suite 500, Philadelphia, PA 19123