Modern Data Infrastructure. "Upskilling" Analysts. Data Team Org Structures. Bolt-On AI. [DSR #237]
❤️ Want to support this project? Forward this email to three friends!
🚀 Forwarded this from a friend? Sign up to the Data Science Roundup here.
This week's best data science articles
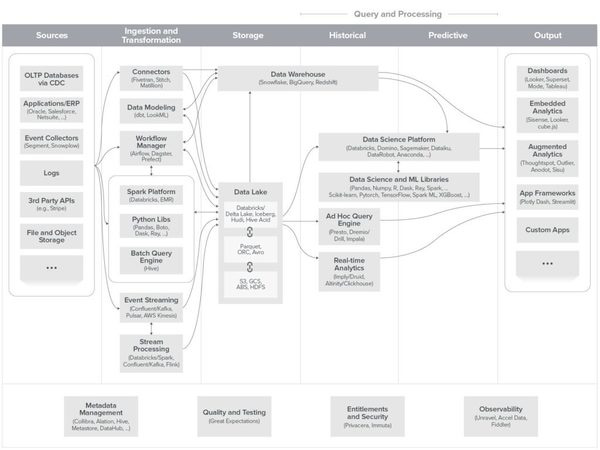
The Emerging Architectures for Modern Data Infrastructure
The graphic above is rather…involved…but it’s quite good / useful. It’s actually the superset of all modern technology architecture diagrams that exist today, the superset of all possible “how we built our data stack” posts. The folks at A16Z have been working on this post for ~6 months at this point and the hard work has paid off.
What I particularly find valuable is the separation of the total stack into separate reference architectures for BI and AI/ML, while recognizing that it’s also possible to combine those two into a single cohesive platform. Having such a clear depiction of how these pieces fit together is incredibly useful.
Companies Are Rushing to Use AI—but Few See a Payoff
I find this type of article annoying and maybe you do too. Someone did a survey of executives at big companies and found that most of them aren’t seeing much value from AI yet. Saved you a click.
So why link to it? I think there’s specifically a problem with this type of survey, and it points to something bigger. The types of companies who benefit most from AI are often technology-first companies. FAANG, Spotify, Stitch Fix, Airbnb, etc. While certainly a part of this is about talent—and those companies have a lions’ share of it—I think it’s more importantly about business model. I don’t believe you just take a fundamentally industrial-age company and just slap AI on top of it and—voila!—profit. I think the companies benefiting from it most have architected how value is provided from the beginning to the end of their operations with AI at the heart. Netflix’s entire content strategy (and thus corporate strategy) is centered around their recommendation algorithm. Etc…you get the point.
If AI is to have the huge impact that I believe it will, most of that impact will not come through “transformation” efforts at existing large companies. It will come through the creation of brand new companies built from the ground up with AI at their core. And so, asking a bunch of large existing businesses whether AI is having a positive impact has an inherent sampling bias—the impact it’s going to have on many/most of them is to threaten their market share. If you want to get a more interesting answer, ask Gong.io how AI is impacting its business.
IMHO, one of the questions that a data scientist should be asking before taking a job is: “Is AI at the heart of this company’s value to its customer or am I just being bolted on?”
Linkedin: 2020 Emerging Jobs Report
Data scientist, data engineer, and now AI specialist are all in the top 15. The AI Specialist role in particular is experiencing pretty stunning growth—74% annual growth over the past 4 years. Not revolutionary, but a quick read.
A few weeks ago, I got curious about how organizations can intentionally retrain analysts for data science roles. This post is the result of a few conversations with data leaders who have been there, done that, and my own research on the topic.
Really interesting topic. One of the most interesting things in data right now is (IMO) figuring out the right career path for a data analyst. One of dbt’s main goals is to create another career path—the analytics engineer—that can be a source of long-term satisfaction and high salaries. But I 100% agree that data science is a legit pathway for someone with analytics skills who begins to ask where their career is going.
If you run a team and want to enable 1:N analysts to go on this path, the right answer isn’t just “stick them in some Coursera classes and start having them work in Python.” Read this post first.
betterwithdata.substack.com • Share
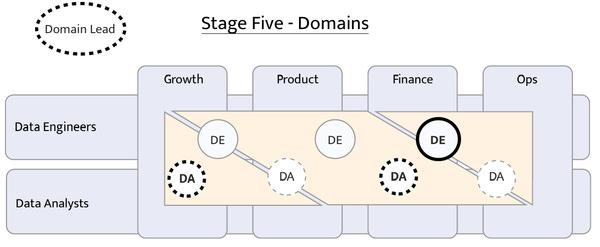
How should our company structure our data team?
Real-life learnings from five data team iterations: centralized, embedded, full-stack, pods and business domains.
Three comments:
Best post on this topic I’ve read on a very long time. Really excited to watch David’s talk at our 2020 user conference, Coalesce.
Holy crap, 5 different org structures over the course of ~4 years? That’s…intense.
The “Domains” structure (pictured above) was a brand new idea to me. It’s interesting. I’m not sure I fully grok it but I’m super-curious to learn more.
Why using Excel Caused Covid-19 Results to be Lost
OK, you almost definitely have heard about this. It’s easy to criticize the folks at PHE for building a terrible system with Excel (and the deprecated-in-the-90’s .xls file format) at its core. But if you believe this “expert”, you’re just as detached from reality as he is:
“Excel was always meant for people mucking around with a bunch of data for their small company to see what it looked like. And then when you need to do something more serious, you build something bespoke that works - there’s dozens of other things you could do. But you wouldn’t use XLS. Nobody would start with that.”
Reality check—Excel is the most-used data tool in existence. If you’re not taking this into account in your thinking, you should be. How are you helping to enable Excel users at your org?
Thanks to our sponsors!
dbt: Your Entire Analytics Engineering Workflow
Analytics engineering is the data transformation work that happens between loading data into your warehouse and analyzing it. dbt allows anyone comfortable with SQL to own that workflow.
The internet's most useful data science articles. Curated with ❤️ by Tristan Handy.
If you don't want these updates anymore, please unsubscribe here.
If you were forwarded this newsletter and you like it, you can subscribe here.
Powered by Revue
915 Spring Garden St., Suite 500, Philadelphia, PA 19123