Modular Analytics. Top 10 AI Trends of 2018. Data Science @ Warby Parker. Deep Learning IRL. [DSR #115]
❤️ Want to support us? Forward this email to three friends!
🚀 Forwarded this from a friend? Sign up to the Data Science Roundup here.
Why Your Analytics Stack should be Modular
Frequently when we’re working with a new client at Fishtown Analytics, it can be a bit overwhelming the number of tools that are required to do sophisticated analytics: event collection, ETL, warehousing, transformation, BI… Some companies try to resolve this complexity by buying a single monolithic product that promises to do all, or a majority of, these tasks. This is a very bad idea.
This post by Roundup reader David Wallace talks about why the only good option when assembling your data stack is to go best-of-breed. If you’re spending any time thinking about your analytics tech stack today, this is a must-read.
blog.modeanalytics.com • Share
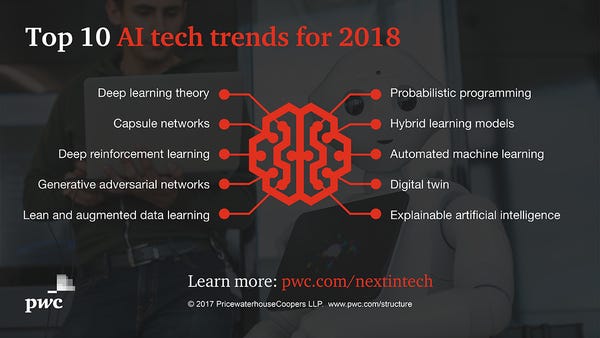
Did you miss the capsule networks flurry a month ago? Haven’t been following along with probabilistic programming? This list is a solid index of terms you should be familiar with—use it to fill in your gaps.
Regular Expressions for Data Scientists
In this tutorial, learn how to use regular expressions and the pandas library to manage large data sets.
Most data scientists use regular expressions by 1) Googling “email regex”, 2) ctrl-c, 3) ctrl-v. Do yourself a favor and spend the hour or two you need to get the fundamentals.
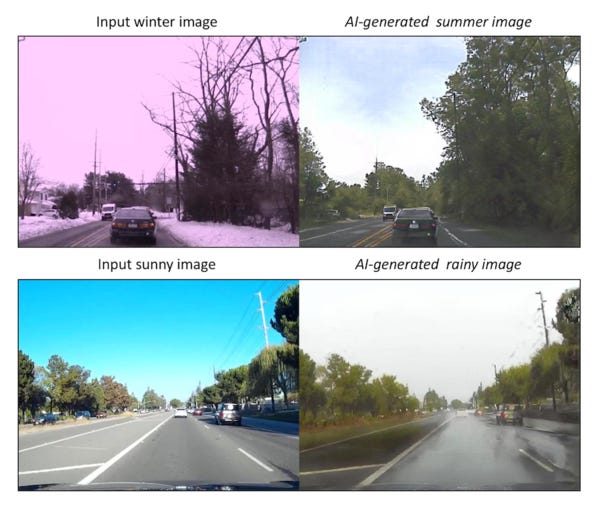
Unsupervised Image-to-Image Translation Networks
This recent research from NVidia @ NIPS 2017 blew me away. Click through and look at these pictures in more detail. I can’t tell that the images on the right were AI-generated. Can you?
Stakeholder-Driven Data Science at Warby Parker
This 30-minute talk by Max Shron, the head of data science at Warby Parker, is awesome. In it, he focuses on answering the question “How do we make sure to build things of value?” This is the hardest question practitioners are struggling with on a day-to-day basis.
Highly recommended.
blog.dominodatalab.com • Share
Using Deep Learning to Solve Real World Problems
There are altogether too many posts talking about deep learning but all-to-few talking about deployment in real-world scenarios. This post is a collection of feedback from several practitioners when Matthew Mayo of KDNuggets polled his Linkedin network. My favorite quote:
These projects require a radically different approach than traditional IT. They are essentially R&D projects, and it is difficult to both project the timelines and set milestones. And forget about trying to manage them internally using the same old processes.
Improving Smiling Detection with Race and Gender Diversity
From a Google talk @ NIPS 2017:
Recent progress in deep learning has been accompanied by a growing concern for whether models are fair for users, with equally good performance across different demographics. (…) We measure race and gender inclusion in the context of smiling detection, and introduce a method for improving smiling detection across demographic groups. (…) Our best-performing model defines a new state-of-the art for smiling detection, reaching 91% on the Faces of the World dataset.
Thanks to our sponsors!
Fishtown Analytics: Analytics Consulting for Startups
At Fishtown Analytics, we work with venture-funded startups to implement Redshift, Snowflake, Mode Analytics, and Looker. Want advanced analytics without needing to hire an entire data team? Let’s chat.
Stitch: Simple, Powerful ETL Built for Developers
Developers shouldn’t have to write ETL scripts. Consolidate your data in minutes. No API maintenance, scripting, cron jobs, or JSON wrangling required.
The internet's most useful data science articles. Curated with ❤️ by Tristan Handy.
If you don't want these updates anymore, please unsubscribe here.
If you were forwarded this newsletter and you like it, you can subscribe here.
Powered by Revue
915 Spring Garden St., Suite 500, Philadelphia, PA 19123