Prioritizing Data Work. Focus on: Data Products! (Databricks, Firebolt, Airbyte, Continual...) [DSR #253]
Quick note before diving in: The Coalesce Call For Proposals deadline is June 25! Coalesce 2020 was absolutely fantastic and I am so excited for this year’s event. The energy, positivity, and new ideas were the professional highlight of my year. (And–I’m hopeful we’ll pull together some level of in-person element for this year!)
So: if you attended last year’s premier analytics engineering conference and/or if you’re looking forward to attending this year’s, please take a minute to:
ask yourself what lightbulbs have gone off in your head over the past year that you just have to share with the community, and
take a minute to think about whose voice you would desperately love to hear from and give them a nomination. Nudges like this are so helpful in encouraging folks to present who might otherwise not step forwards on their own.
CFP is here, and thanks for your support!
- Tristan
—-
❤️ Want to support this project? Forward this email to three friends!
🚀 Forwarded this from a friend? Sign up to the Data Science Roundup here.
I might title this: how to prioritize work in a modern data team. It is a super super interesting topic, and the post goes much deeper than the prior art in this space. This process—"What does the data team work on?“—is so central to the operations of the team and yet is often such a black box.
I won’t try to summarize it further here, the post itself isn’t so long. I do want to highlight the passage below, though, as I think it’s so incredibly critical:
…don’t accept new work without requirements. If a stakeholder is not able or not willing to answer the hard questions about why they want something done, then the work is either not clear enough or not important enough for a data team to work on.
One of the things I feel strongly about re: org dynamics of the modern data team is that it needs to have real organizational power—it needs to be able to say "no” and mean it. If your data team doesn’t truly have the power to say no to stakeholders, it will get sent on all kinds of wild goose chases, be unproductive, experience employee churn, etc. This is one of the reasons why data should report directly to the CEO.
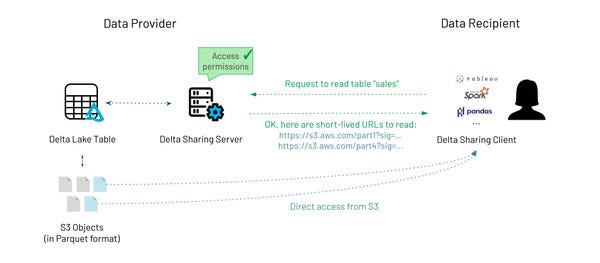
Databricks | Introducing Delta Sharing: an Open Protocol for Secure Data Sharing
Ok this is cool. Instead of Snowflake’s you-can-share-data-with-anyone-as-long-as-they’re-on-Snowflake, this is an open protocol, an open source reference implementation server (host your own or have Databricks manage it for you), all living on top of open source file formats (Delta / Parquet) and other open protocols (REST/HTTP). If you want to create a widespread network effect of data sharing (and all sharing behaviors are fundamentally network-driven) this is the way you have to do it.
From my read, though, there are still things to figure out here. I’m not sure that the most common use case for data sharing is “grab a bunch of Parquet files” which is what this makes easy. That’s not really a knock on the protocol, just a guess that there is likely more to be built on this in the months and years ahead. Which is cool.
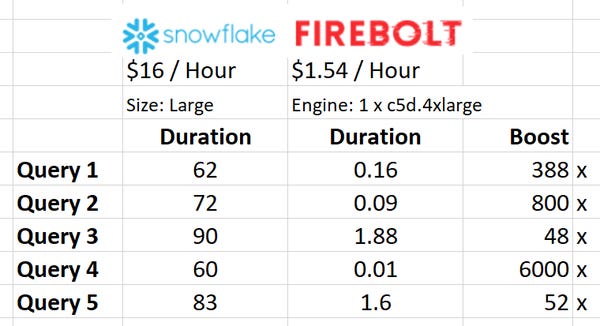
Is FireBolt the Future of Data Platforms?
I just looked back at the archives and realized that I had never covered Firebolt before—what a big miss! I’ve been a fan since meeting the founders a while back. I’m absolutely sold on its merits as a technology, and the company has started to put a truly superstar team together.
There’s a lot to say about why the product is an exciting competitor in the data warehouse ecosystem, but this post does it better than I could. I’m slightly skeptical of the representativeness of the benchmarks above but I absolutely believe that the cost / performance advantage could be very real.
If you are an active Firebolt user I’d love to chat with you live about it!
Airbyte announces $26M Series A for open-source data connector platform
If Firebolt is a welcome newcomer in the cloud data warehouse space, Airbyte is playing the same role in data ingestion. Stitch, the early champion of open source data ingestion with its Singer framework, is something less of a player than it once was after being acquired by Talend a few years ago. It’s the pairing of Meltano and Airbyte who are looking to take up that mantle and compete with much larger players building proprietary products. Competition + openness == nothing but good for the ecosystem.
Over the past ~year there has been a lot of ink spilled in the dbt Community Slack (#tools-data-loaders) about the maturity of these newer platforms. Worth catching up there and joining in the conversation if you’re considering them.
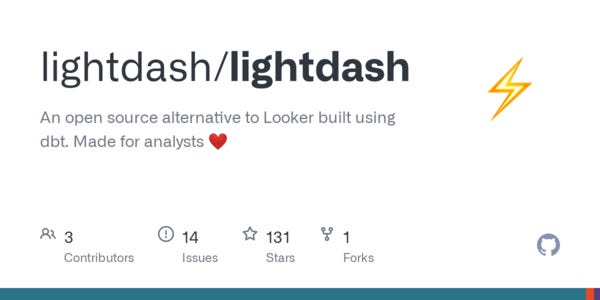
Lightdash: An open source alternative to Looker built using dbt.
👀👀👀
Lightdash removes the gap between your data transformation layer and your data visualization layer. It enables data analysts and engineers to control all of their business intelligence (data transformations/business logic as well as data visualization) in a single place.
Lightdash integrates with your dbt project and gives a framework for defining metrics and specifying joins between models all within your existing dbt YAML files. The data output from your dbt project is then available for exploring and sharing in Lightdash.
Love this type of open experimentation! The product is pretty nascent but the public demo experience is quite solid (no signup required). Need to learn more about this, I haven’t met the folks involved. Reach out if you’re connected!
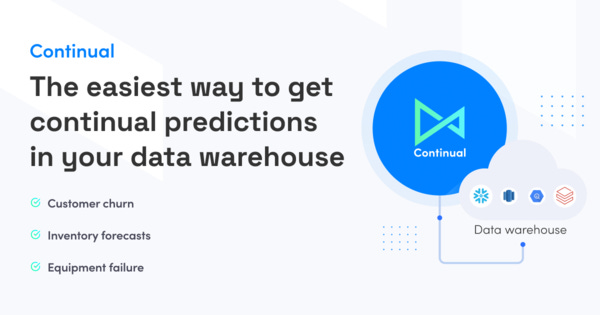
Introducing Continual – the missing AI layer for the modern data stack
Unlike traditional machine learning engineering platforms, Continual is built to empower data and analytics professionals not simply machine learning engineers. If you like SQL and dbt, you’ll love Continual. Unlike most no-code AI tools, Continual is built for production, not exploratory workloads. It has a declarative workflow like SQL that radically simplifies operational AI/ML and delivers continually improving models and predictions.
I’m very bullish on the approach this team is taking and so excited for the launch! I want to start using this internally like…tomorrow.
Data analytics firm Cloudera taken private for $4.7B USD
The article itself isn’t super-interesting, it’s the headline that’s relevant. Couple of thoughts:
Cloudera and Hortonworks merged back in 2018. The line from the press release then: this transaction “will create the world’s leading next generation data platform provider.” These were the two biggest pure-play companies in the Hadoop ecosystem combined into a single entity. The merger valued the combined entity at $5.2B USD.
The company has decreased in value by $.5B over the past three years.
Cloudera’s revenue is in the same ballpark as both Snowflake and Databricks, but it’s totally flat. Both SF and DB are growing revenue at >100% / year.
This is an incredibly clear nail in the Hadoop coffin.
Thanks to our sponsor!
dbt: Your Entire Analytics Engineering Workflow
Analytics engineering is the data transformation work that happens between loading data into your warehouse and analyzing it. dbt allows anyone comfortable with SQL to own that workflow.
The internet's most useful data science articles. Curated with ❤️ by Tristan Handy.
If you don't want these updates anymore, please unsubscribe here.
If you were forwarded this newsletter and you like it, you can subscribe here.
Powered by Revue
915 Spring Garden St., Suite 500, Philadelphia, PA 19123