Real-time ML. MLOps Tooling. The Analytics Engineer. Operationalizing AI Ethics. [DSR #243]
❤️ Want to support this project? Forward this email to three friends!
🚀 Forwarded this from a friend? Sign up to the Data Science Roundup here.
This week's best data science articles
Machine learning is going real-time
Chip Huyen’s recent post is one of the best I’ve read in the ML space in a bit. I’ll let the author summarize it herself:
After talking to machine learning and infrastructure engineers at major Internet companies across the US, Europe, and China, I noticed two groups of companies. One group has made significant investments (hundreds of millions of dollars) into infrastructure to allow real-time machine learning and has already seen returns on their investments. Another group still wonders if there’s value in real-time ML.
There seems to be little consensus on what real-time ML means, and there hasn’t been a lot of in-depth discussion on how it’s done in the industry. In this post, I want to share what I’ve learned after talking to about a dozen companies that are doing it.
There are two levels of real-time machine learning that I’ll go over in this post. Level 1: Your ML system makes predictions in real-time (online predictions). Level 2: Your system can incorporate new data and update your model in real-time (online learning).
MLOps Tooling Landscape v2 (+84 new tools)
Ok I may have been catching up on Chip Huyen’s RSS feed ;) This post is too good to not also share.
Last June, I published the post What I learned from looking at 200 machine learning tools. The post got some attention and I got a lot of messages from people telling me about new tools. I updated the old list to now include 284 tools.
There’s also some fantastic analysis of market trends that show where we’ve been and what has momentum right now. A++
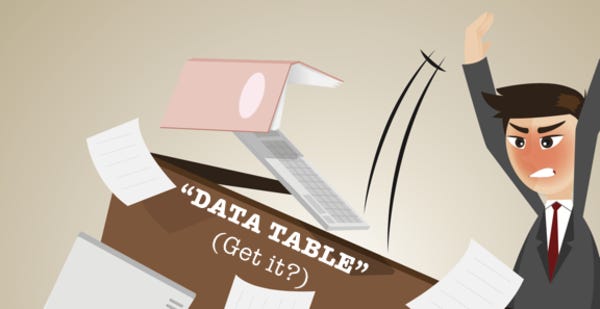
An Analytics Engineer is Really Just a Pissed Off Data Analyst
Sitting through dbt Coalesce conference this week, my biggest takeaway is that data is less trustworthy than ever and people are fired up about it. Often times, an analytics engineer is really just a pissed off analyst who has the tools and motivation to make things better for everyone else.
Hah. I wouldn’t have put it quite like that but…Seth isn’t wrong ;) I often describe myself in 2016 (when we were building v0.1 of dbt) as a frustrated data analyst. Another spitfire paragraph I love:
If your organization isn’t on board with the movement, it’s time to get on the train. It’s almost certain that people in your organization don’t feel like they can get the data they need, can’t make sense of their data, or generally don’t trust the data in their reports or dashboards. Analytics engineering sets out to solve these issues.
Short post, really conveys the essence of the movement that’s going on in the dbt community.
Do you actually clean your data or do you just throw on some Axe Body Spray in the visualization layer?
How do you, as a data entrepreneur cut through the noise and land your first 20 data customers? We’ve talked to 50+ data leaders and practitioners and here’s some of our tips.
Are you building (or considering building) a new data product? I know more than a few readers are. This post is practical and short, covering:
The Value Proposition: What are the biggest pain points for data leaders?
The Go-To-Market: How do you sell your product to data leaders?
The Evaluation: How do data leaders evaluate new products?
Retired UW computer science professor embroiled in Twitter spat over AI ethics and ‘cancel culture’
Pedro Domingos, a well-known ML/AI academic, tweeted:
It’s alarming that NeurIPS papers are being rejected based on ‘ethics reviews’. How do we guard against ideological biases in such reviews? Since when are scientific conferences in the business of policing the perceived ethics of technical papers?
And thus was begun another Twitter-storm. Read the article for more. I link to it specifically because it highlights the extent to which the field is struggling with the very practical question of how to incorporate ethical considerations into the scientific process. I actually don’t believe this is something we’ve been good at throughout the history of science, and while I’m strongly in agreement that AI ethics does matter, I don’t believe it’s such a cut-and-dry answer as to how we operationally achieve that as a scientific community.
That’s not me saying “I agree with Domimgos”–I don’t. But I also think that the broader topic is a fascinating one and doesn’t lend itself to easy answers. It does seem like the NeurIPS ethical review process has been thoughtfully designed.
The White House Launches the National Artificial Intelligence Initiative Office
So…this is a weird thing to link to, especially in the middle of the current absolute mess of a political climate in Washington DC. I want to stay well away from politics, though, and talk about industrial policy and computing.
The idea that government should be neutral on matters of industrial policy, leaving that completely up to the “free market” is ahistorical. It was the involvement of government in the technology industry starting in WWII that led to the creation of the semiconductor industry and then the internet. And we should anticipate that governments will have a major role to play in the development of AI in the coming decades. Other leading countries—most notably China and the UK—have clearly recognized this; the US has been a slower-mover here.
There has started to be some movement, though. The link above has quite a lot of fluff in it and so needs to be read with a critical eye. My read, after going a couple of links deep on it is that the US is still lagging on this topic vs. others, but we’re doing more than we were in 2017.
It’s hard to know how the future will play out, but geopolitics and technology really do interact in a very material way. The fact that ICANN is American has actually mattered over the past 2+ decades, whether one is happy about that or not. As such, following national strategies on the industry we all participate in is—while kind of boring, I admit—quite important.
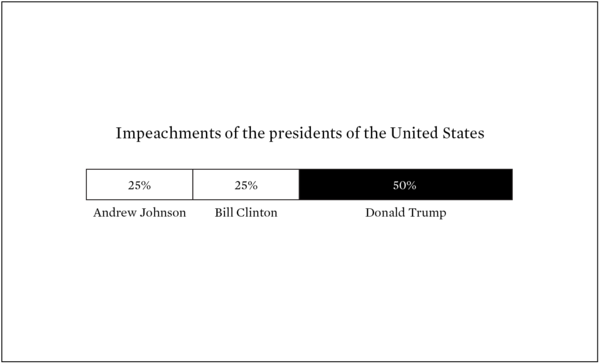
Sometimes the simplest data viz can be the most impactful 😂 From FlowingData.
Thanks to our sponsors!
dbt: Your Entire Analytics Engineering Workflow
Analytics engineering is the data transformation work that happens between loading data into your warehouse and analyzing it. dbt allows anyone comfortable with SQL to own that workflow.
The internet's most useful data science articles. Curated with ❤️ by Tristan Handy.
If you don't want these updates anymore, please unsubscribe here.
If you were forwarded this newsletter and you like it, you can subscribe here.
Powered by Revue
915 Spring Garden St., Suite 500, Philadelphia, PA 19123