Salaries in Data Science. Hiring an Analytics Engineer. The Future of Data Eng. FDA for Algorithms? [DSR #195]
❤️ Want to support this project? Forward this email to three friends!
🚀 Forwarded this from a friend? Sign up to the Data Science Roundup here.
This week's best data science articles
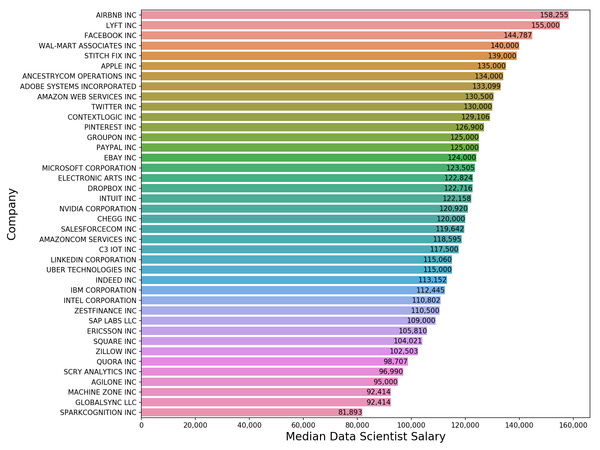
How Much Do Data Scientists Make?
There’s some great data in this post! You don’t actually have to read it, just look through the figures. The one I found most interesting was the one in the thumbnail above (which you may or may not be able to read)—I hadn’t previously seen any source on the relative salaries of top data science employers. Of course, this is purely based on H1-B applications, but it’s not unreasonable to believe that this is a rough proxy for the relative salary bands at these companies.
As much as the enterprise is obsessed with data science and AI, it’s really large tech companies that are willing to pay the top salaries.
towardsdatascience.com • Share
How to hire an analytics engineer
The analytics engineer is the newest member of the modern data team–owning everything from data transformation to testing and documentation. Here’s how to hire one.
Written in collaboration with over a dozen members of the dbt community, this post goes deep into the types of people being hired for this role, interviewing tactics, and sourcing candidates. This is a hard role to hire for and critical for the success of your entire data team.
The Future of Data Engineering
There are four areas, in particular, where I expect to see shifts over the next few years: timeliness, connectivity, centralization, and automation (…)
Fantastic post from an author deeply embedded in the current ecosystem. There really is a long way for the field still to go: it is very much in its nascency.
Must-read.
AI Algorithms Need FDA-Style Drug Trials
Algorithms cause permanent side effects on society. They need clinical tests.
There is a lot of discussion around AI ethics, but there are few actual recommendations on what to do (other than “raise public awareness” and “have the conversation”). I don’t know whether I’m particularly in support of this suggestion, but I do think it is not crazy and it is actually a real suggestion. The three authors are super-qualified on the topic and the article is well-thought-out. Worth a ponder.
Data as a Product vs. Data as a Service
There are two broad mandates that data teams tend to get formed with: 1) provide data to the company, 2) provide insights to the company. These might sound similar — and they’re certainly both important — but they necessitate completely different skillsets. In fact, I’m going to argue that the conflation of these two objectives is exactly what kills good data talent and confounds the hiring process.
This post is an excellent discussion around an important point: should data teams be engaged in helping business units answer specific questions or do they exist to make data available and facilitate self-service?
There is no consensus on this topic right now. My sense is that modern data teams lean towards enabling self-service (“data as product”) because that approach tends to scale better as an organization grows. This approach does, however, rely on business units having strong analytical skillsets of their own.
This article advocates that maturing data teams should skew towards data-as-a-service. I’m not sure that I agree with this perspective but more importantly I think the answer is largely contextual to the particular organization. What’s definitely true is that you need to be intentional about designing the interaction paradigm between your data team and the rest of your org and align your hiring decisions with it.
Thought-provoking.
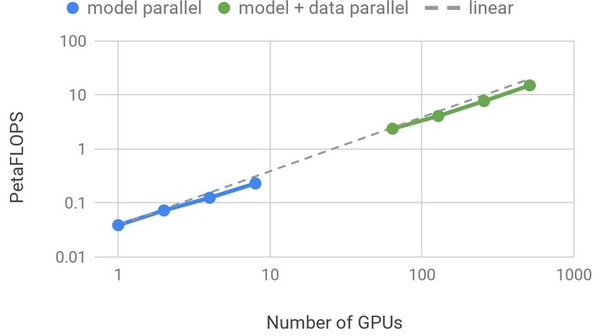
NVIDIA Clocks World’s Fastest BERT Training Time and Largest Transformer Based Model
This is an impressive feat:
The NVIDIA DGX SuperPOD with 92 DGX-2H nodes set a new record by training BERT-Large in just 53 minutes. This record was set using 1,472 V100 SXM3-32GB 450W GPUs and 8 Mellanox Infiniband compute adapters per node, running PyTorch(…)
The post reads like the press release it is, but was interesting nonetheless. My primary takeaway was the level of scalability the team was able to achieve: 76% efficiency vs. baseline in a setup with 512 GPUs. Impressive.
The 5 Graph Algorithms that Data Scientists should know
Great introduction to how to solve common problems using graphs. Excellent explanations, simple code snippets… Very useful introduction to a topic that is too-infrequently discussed.
towardsdatascience.com • Share
Thanks to our sponsors!
dbt: Your Entire Analytics Engineering Workflow
Analytics engineering is the data transformation work that happens between loading data into your warehouse and analyzing it. dbt allows anyone comfortable with SQL to own that workflow.
Stitch: Simple, Powerful ETL Built for Developers
Developers shouldn’t have to write ETL scripts. Consolidate your data in minutes. No API maintenance, scripting, cron jobs, or JSON wrangling required.
The internet's most useful data science articles. Curated with ❤️ by Tristan Handy.
If you don't want these updates anymore, please unsubscribe here.
If you were forwarded this newsletter and you like it, you can subscribe here.
Powered by Revue
915 Spring Garden St., Suite 500, Philadelphia, PA 19123