Scaling Knowledge. 5 Tips for Better DS Writing. Infrastructure @ Stitch Fix. New Data on DS Jobs. [DSR #152]
Special thanks to Domino Data Lab for sponsoring this week’s Roundup. I’ve actually jumped on the “Sponsored Content” bandwagon—you’ll see a post from Domino below with a “Sponsored” callout in the title. We’ll only accept sponsorships like this from organizations we think highly of and—of course—will always prioritize your reading experience.
Enjoy this week’s issue :)
- Tristan
❤️ Want to support us? Forward this email to three friends!
🚀 Forwarded this from a friend? Sign up to the Data Science Roundup here.
This Week's Most Useful Posts
Data scientists, analysts, and engineers are ultimately employed by companies for the single purpose of producing and disseminating knowledge. And yet we spend all of our time talking about the producing part, with very little time dedicated to the disseminating part. As a result, we as an industry all-too-frequently produce amazing analysis that we utterly fail to disseminate throughout our organizations. This is a big problem.
Ever been frustrated that the analysis you’re producing isn’t being used? This post is for you. Here’s my favorite quote:
In order to disseminate factual knowledge, it is insufficient to simply disseminate data. Factual knowledge must include the data themselves as well as the knowledge about how those data were produced.
blog.fishtownanalytics.com • Share
5 Bits of Practical Advice for Data Science Writing
I’ve mentioned recently how important I consider writing for my own personal growth, and this author’s advice resonates with me. It’s hard to start writing in this space—it definitely feels overwhelming at first.
Here are the five topics. Read the post for more info on each.
Aim for 90%
Consistency helps
Don’t worry about your credentials
The best tool is the one that gets the job done
Read widely and deeply
towardsdatascience.com • Share
Themes and Conferences per Pacoid! (Sponsored)
In this brand new monthly series, Paco Nathan summarizes highlights from recent industry conferences, new open source projects, interesting research, great examples, amazing people, etc.—all pointed at how to level up your organization’s data science practices.
Read episode 1 today, and subscribe to the Domino Data Science Blog to automatically get emailed next month’s episode!
blog.dominodatalab.com • Share
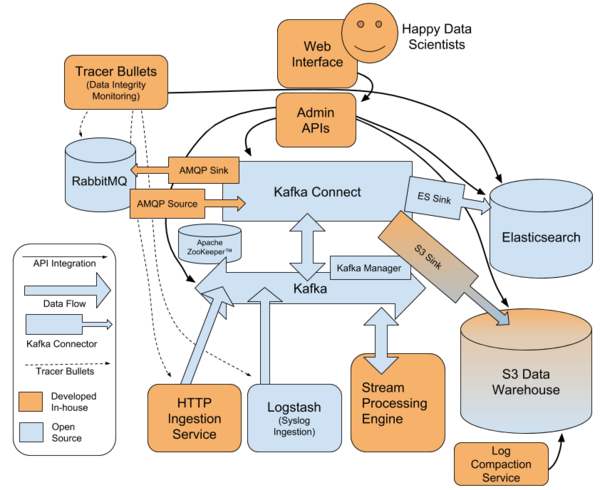
Stitch Fix: Putting the Power of Kafka into the Hands of Data Scientists
It’s been a while since I read an amazing “here’s how we built our killer data infrastructure” post, but this one more than scratched the itch. It details a year-long project involving design, technology selection, and implementation.
The original goals:
Fully self-service for data scientists (remember, this is the company that believes engineers shouldn’t write ETL)
Enable real-time analysis
High-fidelity change capture
Even if you’re not imagining going through a project like this tomorrow, this post is the distillation of three people’s thinking over the course of an entire year, and contains tons of wisdom. My absolute favorite part was at the end where the author discusses their strong preference for investing in open source:
Never have I worked on a project this challenging that involved writing so little code. We spent about two-thirds of the project timeline on research, design, debate and prototyping. Over months we whittled away at every component until it was as small and maintainable as possible. We tried to salvage as much as we could from our legacy infrastructure. More than once we submitted patches upstream to Kafka Connect to avoid extra complexity on our end.
An amazing model for much of modern software development.
multithreaded.stitchfix.com • Share
New Linkedin Report: Demand for Data Scientists is Off the Charts
This post is a good summary of results from a recent Linkedin research report (just CMD-F on “data science” to find the relevant section). Here’s the biggest result:
LinkedIn calculates that, in August, employers were seeking 151,717 more data scientists than exist in the U.S.
New York and San Francisco, unsurprisingly, top the charts of cities where data science talent supply lags demand.
Google Dataset Search: Making it Easier to Discover Datasets
In today’s world, scientists in many disciplines and a growing number of journalists live and breathe data. There are many thousands of data repositories on the web, providing access to millions of datasets; and local and national governments around the world publish their data as well. To enable easy access to this data, we launched Dataset Search, so that scientists, data journalists, data geeks, or anyone else can find the data required for their work and their stories, or simply to satisfy their intellectual curiosity.
I spent a bit of time with it and was impressed. Another tool for your tool belt.
Thanks to our sponsors!
Stitch: Simple, Powerful ETL Built for Developers
Developers shouldn’t have to write ETL scripts. Consolidate your data in minutes. No API maintenance, scripting, cron jobs, or JSON wrangling required.
The internet's most useful data science articles. Curated with ❤️ by Tristan Handy.
If you don't want these updates anymore, please unsubscribe here.
If you were forwarded this newsletter and you like it, you can subscribe here.
Powered by Revue
915 Spring Garden St., Suite 500, Philadelphia, PA 19123