Survival Analysis @ Better. Presto @ Pinterest. Dagster. Data Science in Organizations (a two-fer). [DSR #194]
Join me on Tuesday @ 11AM ET to talk about deploying Snowflake + dbt at scale at Chesapeake Energy. Ryan Goltz and Chesapeake are breaking new ground in the enterprise, aggressively rolling out a modern tech stack well in advance of many of their peers; I’m excited to join him to talk about it. If you’re currently considering migrating data stacks, this conversation will be well worth it.
Also: it’s been a stellar couple of weeks—there are some great posts in this week’s issue. Thanks internet.
- Tristan
❤️ Want to support this project? Forward this email to three friends!
🚀 Forwarded this from a friend? Sign up to the Data Science Roundup here.
This week's best data science articles
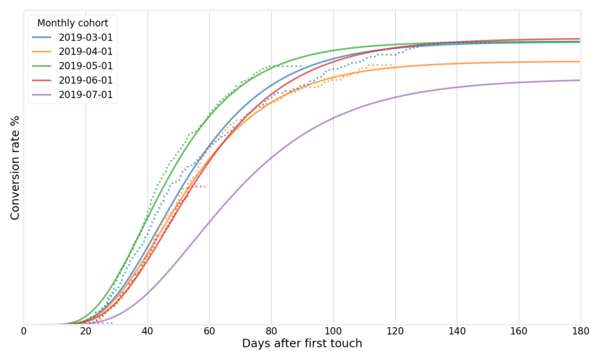
Modeling conversion rates and saving millions of dollars using Kaplan-Meier and gamma distributions
If you’ve ever wanted to do a better job of analyzing conversion rates, conversion rate changes over time, estimating lifetime value without needing to wait the entire lifetime, this post is for you. It’s been going around Data Twitter this past week and it delivers. It doesn’t just do a fantastic job of outlining problems and solutions, it actually delivers up a Python package, Convoys, that helps you do everything from the post.
This is one of the most common hard problems in modern analytics, and this is the best single post I’ve seen to take you from 0 to 60 on it. If you try Convoy I’d love to hear about your experiences.
Truly epic. Forward this to post your team.
Q. Embedded or centralized?
A. Both.
Embedded for context, relevance, communication efficiency, and to be in sync; centralized for hiring and promotion purposes, for peer review, and for sharing and maintaining best practices
Models for integrating data science teams within organizations
This post expands on the above two tweets (and is by the same author). IMO, it is the single best piece of writing on how to integrate data science into a larger organizational context.
Remember: if your data science team isn’t effectively integrated into the larger organization, it will have limited or no impact. The quality of your work is irrelevant if your organization isn’t set up to internalize and act on it.
In one of my last newsletters, I linked to a Reddit thread, “How does data science work in the consulting space?” and said that if there was enough interest, I’d cover some aspects of data science consulting in the newsletter from time to time. This is the first of those pieces.
Vicki Boykis has some good thoughts on data science consulting. The post points towards the key difference that being a data science consultant forces you to do: quantify the value that your work produces, and then convince others of that value.
While I am seeing a rise in the number of data science consultants out there (which is great!), the reason this point is interesting to me is actually that this skill—quantifying and convincing others of the value of your work—is actually critical for in-house DS’s as well, although its criticality is more hidden. Often, an inability to convince others of value is the underlying reason for your team’s lack of progress, but it’s hard to even realize that’s true because you get that feedback as coded messages. When you’re a consultant, you simply fail to sell the work; the feedback is much more clear.
The very best data science leaders create a vision of data science within an organization and make sure that stakeholders through the business buy in. They then continue to engage in the “selling” process internally with every single project. As in Pardis’ post above, your work, without organizational buy-in, creates no value.
Today the team at Elementl is proud to announce an early release of Dagster, an open-source library for building systems like ETL processes and ML pipelines.
I linked to an article about Prefect in the last issue; here’s a similar post from competitive product, Dagster. The post itself is solid, but what I think is particularly interesting here is that this “next generation orchestration solution” space seems to have gone from cold to hot in the space of about a month. Word on the street is that both Prefect and Elementl (which makes Dagster) are both closing (or have closed?) sizable venture rounds within just a few weeks of each other.
As a result, there will be a lot of innovation in this space over the coming years, which is exciting: Airflow was a huge step forwards, but it’s been several years since that paradigm shift and our data engineering challenges are very much not solved. I’m excited to watch this space evolve.
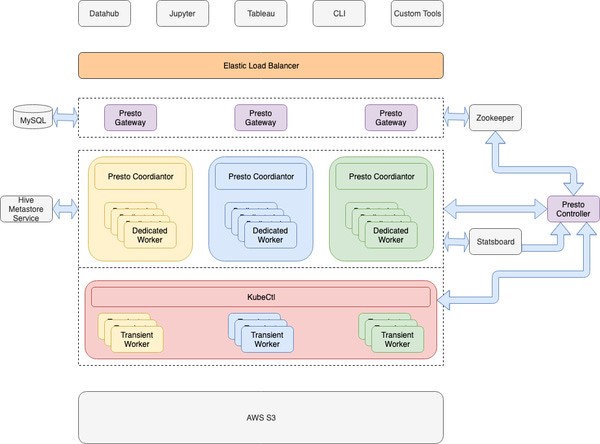
We have hundreds of petabytes of data and tens of thousands of Hive tables. Our Presto clusters are comprised of a fleet of 450 r4.8xl EC2 instances. Presto clusters together have over 100 TBs of memory and 14K vcpu cores. Within Pinterest, we have close to more than 1,000 monthly active users (out of total 1,600+ Pinterest employees) using Presto, who run about 400K queries on these clusters per month.
…that’s a lot. To give you a sense of scale, those EC2 instances alone would cost ~$700k / month if they were undiscounted (which they most certainly are not).
This post is particularly interesting to me because, in the era of Snowflake & Bigquery, it hasn’t always been 100% clear to me what Presto’s long-term role in the ecosystem is. I still don’t have a perfectly clear answer to that question, and I do believe that the modern commercial databases absolutely reduce the number of appropriate deployments for Presto, but my theories are:
Cost. My guess is that at this scale using Snowflake or Bigquery would be many times the cost of Presto.
Control. There are many decisions that the team at Pinterest has made that would not be implement-able in a commercial product.
Competition. Companies of this scale don’t necessarily want their data to be running through a commercial product owned by another company.
It all eventually comes down to resources. It’s very clear reading this post that Pinterest has a huge investment in Presto and that it’s working quite well for them. It’s also clear that below some threshold, it simply wouldn’t make sense to invest the resources to run this type of infrastructure effectively.
Making Apache Spark Effortless for All of Uber
Apache Spark is a foundational piece of Uber’s Big Data infrastructure that powers many critical aspects of our business. We currently run more than one hundred thousand Spark applications per day, across multiple different compute environments. Spark’s versatility, which allows us to build applications and run them everywhere that we need, makes this scale possible.
However, our ever-growing infrastructure means that these environments are constantly changing, making it increasingly difficult for both new and existing users to give their applications reliable access to data sources, compute resources, and supporting tools. Also, as the number of users grow, it becomes more challenging for the data team to communicate these environmental changes to users, and for us to understand exactly how Spark is being used.
This post is intense, to say the least. I don’t think I’ve read anything that goes deeper on this topic, so strap in.
Thanks to our sponsors!
dbt: Your Entire Analytics Engineering Workflow
Analytics engineering is the data transformation work that happens between loading data into your warehouse and analyzing it. dbt allows anyone comfortable with SQL to own that workflow.
Stitch: Simple, Powerful ETL Built for Developers
Developers shouldn’t have to write ETL scripts. Consolidate your data in minutes. No API maintenance, scripting, cron jobs, or JSON wrangling required.
The internet's most useful data science articles. Curated with ❤️ by Tristan Handy.
If you don't want these updates anymore, please unsubscribe here.
If you were forwarded this newsletter and you like it, you can subscribe here.
Powered by Revue
915 Spring Garden St., Suite 500, Philadelphia, PA 19123