The AI Hierarchy of Needs, Modern Programming, and Bat Detectors [DSR #97]
❤️ Want to support us? Forward this email to three friends!
🚀 Forwarded this from a friend? Sign up to the Data Science Roundup here.
Two Posts You Can't Miss
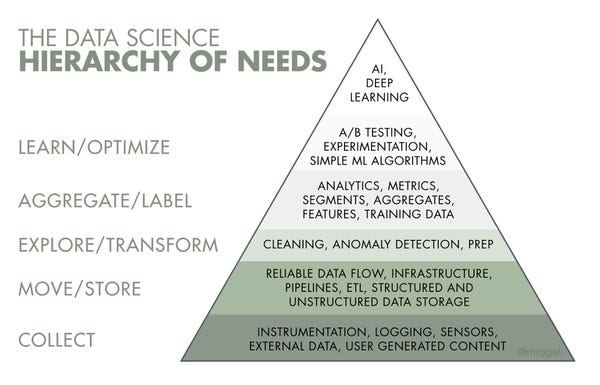
Monica Rogati’s newest post is must read.
From stealth hardware startups to fintech giants to public institutions, teams are feverishly working on their AI strategy. It all comes down to one crucial, high-stakes question: ‘How do we use AI and machine learning to get better at what we do?’
More often than not, companies are not ready for AI. Maybe they hired their first data scientist to less-than-stellar outcomes, or maybe data literacy is not central to their culture. But the most common scenario is that they have not yet built the infrastructure to implement (and reap the benefits of) the most basic data science algorithms and operations, much less machine learning.
Think of AI as the top of a pyramid of needs. Yes, self-actualization (AI) is great, but you first need food, water and shelter (data literacy, collection and infrastructure).
Closing the Loop Between Data Analysis and Action
I can’t believe I’m linking to a feature release blog post, but I really do think this is a thought-provoking topic. Looker just announced that you can now build queries and then automatically export the results of those queries to Segment, which in turn allows you to send the data to any of your other Segment-connected products. Build an email list in your data warehouse and export it to your ESP, etc.
The reason I find this fascinating is because it’s one of the first forays into removing humans from the analytics loop in the mainstream BI space. There’s all of this data locked up in our data tech stacks, but it’s surprisingly hard (and human-intensive) to take that data and plug it back into operational systems.
If the past five years have been focused on building software to consolidate and analyze data, I think the next five years might see the rise of tools to plug these newfound insights back into operational systems. This would change much about how businesses operate and would significantly elevate the role of the data scientist / analyst in the process.
This Week's Top Posts
Kickstarter: How We Built Automated Support
Kickstarter gets a ton of support tickets, and many of them can be answered with standard auto-replies. The engineering team built a model, plugged it into the Zendesk API to enable it to automatically send responses, and deployed the whole thing as a production microservice.
Awesome case study of high-functioning data science.
kickstarter.engineering • Share
If you spend any appreciable part of your day writing code, this is an absolute must-read. Programming is done differently today than it was done 20 years ago, and there is an emerging consensus on what is “good” and “bad”. This article is the most clear explication of this I’ve seen.
Is it time to tune up your (or your team’s) workflow?
I love a good chart redesign. This author starts with an example of a poorly designed chart—representing years as color bars, as seen at left—and proceeds to walk through the process of improving it. It’s incredibly useful to see it done over and over again because it steadily improves your visualization design instincts.
Short, practical.
nsaunders.wordpress.com • Share
I’ve covered the reproducibility crisis and p-hacking before—I’m fascinated by the topic. There’s a new paper out, co-authored by 72 scientists, that’s proposing a “quick fix” while a more long-term solution is proposed: simply change the required threshold to .005.
.05 is not a universal constant of nature—it’s just something we all agreed on. It’s now cheaper and more accessible to run experiments; is 1/20 really “significant” any more?
Detecting Bats by Recognizing Their Sound with TensorFlow
I couldn’t possibly improve on this description. Amazing.
Last week I discovered that there are bats behind my apartment. I immediately grabbed my “bat detector”: a device that converts the ultrasound signals bats use to echolocate from an inaudible frequency range to an audible one. The name “bat detector” thus is a lie: you can use it to detect bats, but it does not detect bats itself. In this tutorial I will show you how to build a real bat detector using TensorFlow.
Emphasis mine.
Super-cool project by a recent data science bootcamp grad on developing a classifier to label vegetation on satellite images.
Predicting Personality from Book Preferences with User-Generated Content Labels
To our knowledge, this is currently the largest study that explores the relationship between personality and book content preferences.
It shouldn’t be at all surprising that what we read is predictive of our personality traits. The authors analyzed Goodreads tags combined with Facebook personality assessment and found strong relationships. Scroll to the results section for findings.
Data viz of the week
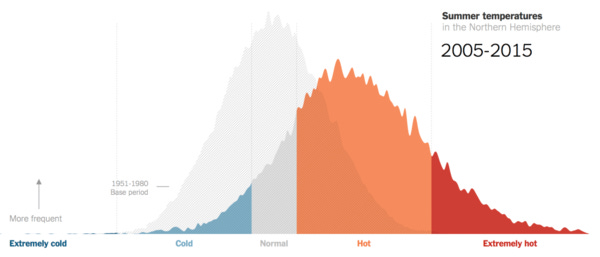
...impactful 🔥 🙁
Thanks to our sponsors!
Fishtown Analytics: Analytics Consulting for Startups
At Fishtown Analytics, we work with venture-funded startups to implement Redshift, Snowflake, Mode Analytics, and Looker. Want advanced analytics without needing to hire an entire data team? Let’s chat.
Stitch: Simple, Powerful ETL Built for Developers
Developers shouldn’t have to write ETL scripts. Consolidate your data in minutes. No API maintenance, scripting, cron jobs, or JSON wrangling required.
The internet's most useful data science articles. Curated with ❤️ by Tristan Handy.
If you don't want these updates anymore, please unsubscribe here.
If you were forwarded this newsletter and you like it, you can subscribe here.
Powered by Revue
915 Spring Garden St., Suite 500, Philadelphia, PA 19123