The Data Science Roundup on Coronavirus. [DSR #222]
Hi! I took last week off as I have been scrambling to rebuild a sense of normalcy in my life right now. Two weeks into working from home full-time without any child care and a new normal has started to set in, so I wanted to get back to it.
This week, most of the posts I’ve linked to are about the current pandemic, as it’s dominated both the mainstream news as well as the news flowing out of the data community. This is not going to be true indefinitely, but there’s great work being done right now and I wanted to share it. If you’ve found resources you love, please send them my way.
One thing I want to make a point to say before diving into this week’s issue: I am not an expert on anything related to public health / epidemiology / virology. There is a data angle to this pandemic and, as I find posts that I think are useful and relevant, I’ll be linking to them. But I’m going to be careful not to share my personal opinions on things like “what should you do?” I’m just a guy with a newsletter about data and am in no way qualified to give you advice about your health.
I hope you and your loved ones are safe and healthy.
- Tristan
—-
❤️ Want to support this project? Forward this email to three friends!
🚀 Forwarded this from a friend? Sign up to the Data Science Roundup here.
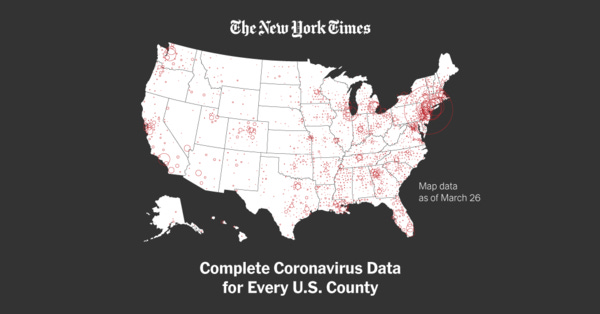
The New York Times | We’re Sharing Coronavirus Case Data for Every U.S. County
With no detailed government database on where the thousands of coronavirus cases have been reported, a team of New York Times journalists is attempting to track every case.
There’s a Github repo with two CSV files—states and counties—and it’s updated daily with both new data and corrections. If you’re building any data products associated with the current pandemic, this is the most well-maintained source of data on US-based cases that I’ve seen. The NYT is having journalists directly survey local health departments on a daily basis and report longitudinal data back.
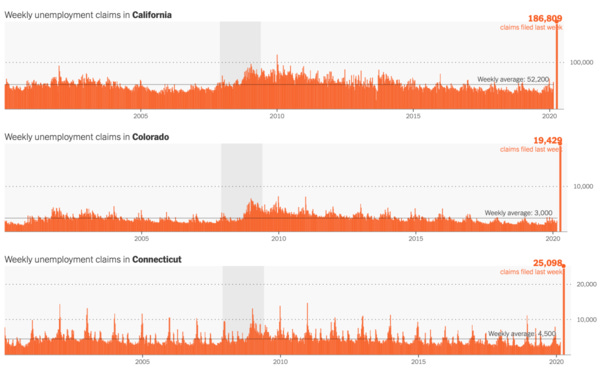
Unemployment Spike | FlowingData
This downturn is different because it’s a direct result of relatively synchronized government directives that forced millions of stores, schools and government offices to close. It’s as if an economic umpire had blown the whistle to signal the end of playing time, forcing competitors from the economic playing field to recuperate. The result is an unusual downturn in which the first round of job losses will be intensely concentrated into just a few weeks.
This is perhaps the most visually striking representation of the current economic situation that I’ve seen. We haven’t experienced anything quite like this before.
Ten Considerations Before You Create Another Chart About COVID-19
As a public health professional, might I ask: Please consider if what you’ve created serves an actual information need in the public domain. Does it add value to the public and uncover new information? If not, perhaps this is one viz that should be for your own use only.
Coronavirus is not the opportunity you were looking for to build your data science portfolio. Sure, play around with the data, but keep it to yourself. My wife happens to be an epidemiologist and public health professional, and this gives me extreme confidence that I have no business whatsoever saying anything publicly about COVID-19. Unless you have some specific expertise here, write your Towards Data Science submission on something else.
The State of the Restaurant Industry
See the impact of coronavirus on the restaurant industry. Data updated daily by country, state, and city.
This is the first hard data I’ve seen on restaurant impact, published by OpenTable based on their usage statistics. I’m a little confused about how so many numbers are down by a full 100%—I’m reasonably confident that there are restaurants in some states in the US still serving sit-down customers. Maybe I’m not understanding the dataset? Maybe OpenTable is mandating shutdowns regardless of government policy? Not sure. Still, this is an interesting dataset and I’ll be particularly interested in watching it as things eventually start to open back up.
Substantial undocumented infection facilitates the rapid dissemination of novel coronavirus
This is fascinating. We’re all aware that there “confirmed cases” necessarily under-reports the actual number of cases in the wild. The question is, by how much? These authors developed a model to find out.
Estimation of the prevalence and contagiousness of undocumented novel coronavirus (SARS-CoV2) infections is critical for understanding the overall prevalence and pandemic potential of this disease. Here we use observations of reported infection within China, in conjunction with mobility data, a networked dynamic metapopulation model and Bayesian inference, to infer critical epidemiological characteristics associated with SARS-CoV2, including the fraction of undocumented infections and their contagiousness. We estimate 86% of all infections were undocumented (95% CI: [82%–90%]) prior to 23 January 2020 travel restrictions.
science.sciencemag.org • Share
All numbers are made up, some are useful
But the reality is that the world we live in is extremely messy, depends on a lot of moving, different variables, and is always shifting under our feet. (…) So the more context-filled information we give and receive, the better it is. And that, maybe, is the only real truth out there.
Another great Normcore from Vicki Boykis. It’s a great post and I largely agree with her point (collection errors, subjective metric definitions, and plain simple inability to know everything mean that most numbers are a bit hand-wave-y). In general, we could all use reminders not to treat numbers that we receive in many contexts of our lives as though they were chiseled into stone tablets by a higher power.
I think I disagree with two aspects of the post:
Maybe I’m a cock-eyed optimist, but I legitimately do believe that we’re actually getting better at this stuff. While most people and organizations are not currently good at “data” (broadly defined), I’m in the privileged position of actually getting to watch the entire industry improve in real-time. We are not going to be terrible at this forever, and I think the arc of progress is very relevant to the overall point Vicki is making.
While the universe is too hard for us to observe with sufficient resolution today to get a good handle on the answer to a question as nuanced as “what is the COVID-19 survival rate?”, this is not due to a lack of underlying Platonic truth. With a sufficiently well-specified question and an omnipotent dataset, Truth does in fact exist. The problem is that we typically aren’t lucky enough to have either.
Thanks to our sponsors!
dbt: Your Entire Analytics Engineering Workflow
Analytics engineering is the data transformation work that happens between loading data into your warehouse and analyzing it. dbt allows anyone comfortable with SQL to own that workflow.
Stitch: Simple, Powerful ETL Built for Developers
Developers shouldn’t have to write ETL scripts. Consolidate your data in minutes. No API maintenance, scripting, cron jobs, or JSON wrangling required.
The internet's most useful data science articles. Curated with ❤️ by Tristan Handy.
If you don't want these updates anymore, please unsubscribe here.
If you were forwarded this newsletter and you like it, you can subscribe here.
Powered by Revue
915 Spring Garden St., Suite 500, Philadelphia, PA 19123