The Modern Data Stack. Data Intuition. Selective Queries on Snowflake. Amundsen Commercialization. [DSR #240]
❤️ Want to support this project? Forward this email to three friends!
🚀 Forwarded this from a friend? Sign up to the Data Science Roundup here.
This week's best data science articles
The modern data stack: past, present, and future
I don’t get enough time to write these days, but when I do, I try to write 5,000 words at a go ;) Here’s the organizing principle behind my most recent post:
(…) while there certainly have been incremental advances in [the products that make up the modern data stack over the past four years], none of their core user experiences has fundamentally changed. If you fell asleep, Rip Van Winkle-style, in 2016 and woke up today, you wouldn’t really need to update your mental model of how the modern data stack works all that much. More integrations, better window function support, more configuration options, better reliability… All of these are very good things, but they suggest a certain maturity, a certain stasis. What happened to the massive innovation we saw from 2012-2016?
It covers a lot of ground and hopefully helps put a frame around where we are as an industry. If you enjoy this super-zoomed-out perspective, join me on Tuesday for my talk: Organizational Epistemology. Or: How do we Know Stuff?
The author, a Principal Data Scientist @ Mozilla, names a thing that I’ve been aware of forever but didn’t have a name for: data intuition. Here’s his definition:
Data Intuition is a resilience to misleading data and analyses.
Have you ever taken a look at a dashboard, a spreadsheet, a whatever and within the first few minutes said “that doesn’t look right”? That’s data intuition. IMO it’s one of the most valuable skills that you can have as a data professional. It comes less from understanding how the data asset in question was produced and more from reality-testing the results themselves.
Great, short read!
Using the Search Optimization Service — Snowflake Documentation
OK, yeah, I’m linking to the Snowflake docs. Not the kind of thing I tend to do, but I’m pretty psyched about this new feature. It falls squarely into the camp of “things that Snowflake is doing right that I haven’t seen the other data warehouses doing.” What, exactly, does it do?
The search optimization service aims to significantly improve the performance of selective point lookup queries on large tables. A point lookup query returns only one or a small number of distinct rows.
This is an incredibly common, OLTP-style read query. “Show me the profile of a given customer” and a million other similar requests. We have (many times!) created data systems where the modern data stack was used to ingest, model, and store the data to support these types of workloads, but then in order to build production applications on top of them we would load the modeled data into a more traditional OLTP database to take advantage of the response time properties for this type of query. Being able to serve these types of selective, interactive queries directly on Snowflake will make developing these types of applications dramatically easier.
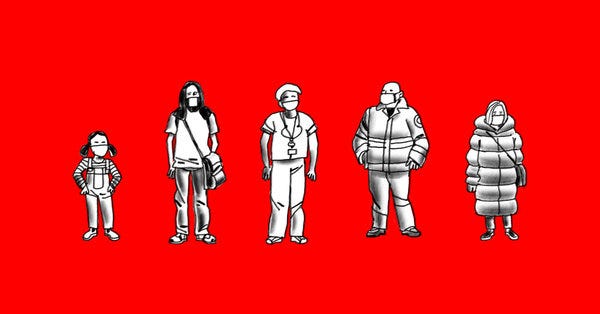
When Can I Get a Coronavirus Vaccine in America?
The rare covid link from me (who needs another one, right?). The NYTimes continues to be best-in-class in both their interactive data visualization and dataviz design. I’m positive you’ve asked this question for yourself in recent weeks and I can’t imagine a better tool to answer it.
The data production-consumption gap
More metadata news! Two of the core members of the Amundsen team @ Lyft have left to commercialize the product, calling the company Stemma. Lots and lots of action going on in this space…!
This is the founding post. If you’re a long-time reader of this newsletter you’re well-familiar with the themes.
mark-grover.medium.com • Share
Customizing Triggers with Concealed Data Poisoning
🤔
We expose a new vulnerability in NLP models that is difficult to detect and debug: an adversary can insert concealed poisoned examples that cause targeted errors for inputs which contain a selected trigger phrase. Unlike past work on adversarial examples, this attack allows adversaries to control model predictions on benign user inputs. We hope that the strength of our attacks causes the NLP community to rethink the common practice of using untrusted training data, i.e., emphasize data quality over data quantity.
Thanks to our sponsors!
dbt: Your Entire Analytics Engineering Workflow
Analytics engineering is the data transformation work that happens between loading data into your warehouse and analyzing it. dbt allows anyone comfortable with SQL to own that workflow.
The internet's most useful data science articles. Curated with ❤️ by Tristan Handy.
If you don't want these updates anymore, please unsubscribe here.
If you were forwarded this newsletter and you like it, you can subscribe here.
Powered by Revue
915 Spring Garden St., Suite 500, Philadelphia, PA 19123