The State of ML, Facebook's Algorithmic Editor, an Introduction to Ensembles, and more!
What data geeks got wrong, and right, in the 2016 US elections. A focus on ensemble learners: what they are, how they work, and applied examples. The state of machine intelligence in a single infographic. And the definitive guide on how to p-hack (or how to detect it).
Share via Twitter | Share on Facebook | forward this email
Referred by a friend? Sign up.
Focus on: The 2016 Election
As one of the first elections where polling models, data journalism, and information bubbles were on such prominent display, the data of the 2016 US presidential election was fascinating, although its quality was disappointing. It’s clear that data scientists, journalists, and product managers need to do better.
The Models Were Telling Us Trump Could Win
Nate Silver, as usual, was the best of the lot when it came to forecasting this election. 538’s final prediction was around 70/30 Clinton, as opposed to the 90+% chance that other establishments were giving her. This article talks about what Silver did right: stress testing. In an environment where it’s impossible to know the error bars around your sample, it’s the best technique a statistician has to forecast likely outcomes.
From the article: “he plays out the possibilities, makes the risks transparent, and puts you in a position to evaluate them. That is how you’re supposed to analyze situations with inherent uncertainty.”
This is an important read as both a voter and a data scientist.
📰 Media in the Age of Algorithms
Did Facebook’s newsfeed algorithms provide a backdrop against which a Trump candidacy could succeed? I think it’s obvious that the product where almost half of the US population goes to get its news certainly has an impact on voting. In this post, Tim O'Reilly discusses the ethics of algorithm design for the news feed. Two great quotes:
“The essence of algorithm design is not to eliminate all error, but to make results robust in the face of error.”
“The key question to ask is not whether Facebook should be curating the news feed, but HOW.”
Focus on: Ensembles
An Introduction to Ensemble Learners
From the article: “Algorithm selection can be challenging for machine learning newcomers. Often when building classifiers, especially for beginners, an approach is adopted to problem solving which considers single instances of single algorithms.
"However, in a given scenario, it may prove more useful to chain or group classifiers together, using the techniques of voting, weighting, and combination to pursue the most accurate classifier possible. Ensemble learners are classifiers which provide this functionality in a variety of ways.”
One indication of how useful ensembles are: almost every winning solution to a Kaggle competition involves an ensemble. Here’s a great post on why/how.
inejc/painters: Winning solution for the Kaggle competition Painter by Numbers
Speaking of ensembles winning Kaggle, here’s an excellent writeup by the winner of a recent Kaggle competition on his solution. The entire writeup is worth reading to walk through the author’s approach, but the real payload is at the end: “The bad news is that the described algorithm is not good at extrapolating to unfamiliar artists. This is largely due to the fact that same identity verification is calculated directly from the two class distribution vectors.”
So: a ton of work, a winning solution, but not broadly applicable. This stuff is hard.
Top Data Science Articles
🤖 The Current State of Machine Intelligence 3.0
This update of last year’s industry infographic is a must-read. I’m not even going to summarize it for you, just read it.
☑️ A Checklist to Avoid P-Hacking
I know, I know, I’m obsessed with p-hacking. If you’re tired of reading about the undermining of many of the scientific results in psychology over the past several decades, I’m sorry, but this paper is just too good:
“The designing, collecting, analyzing, and reporting of psychological studies entail many choices that are often arbitrary. The opportunistic use of these so-called researcher degrees of freedom aimed at obtaining statistically significant results is problematic because it enhances the chances of false positive results and may inflate effect size estimates.”
This is an instruction manual to commit, or to detect, p-hacking.
journal.frontiersin.org • Share
Data viz of the week
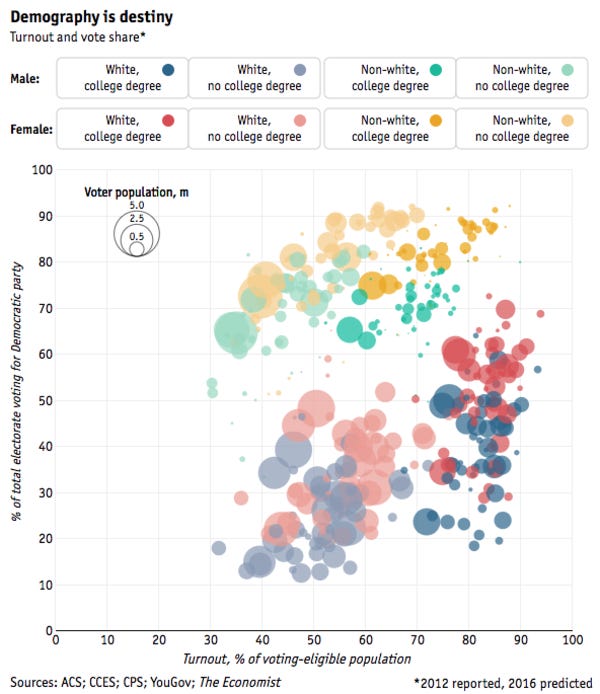
This one chart has more explanatory power than 24 hours of cable news.
Made possible by our sponsors:
Fishtown Analytics: Analytics Consulting for Startups
Fishtown Analytics works with venture-funded startups to implement Redshift, BigQuery, Mode Analytics, and Looker. Want advanced analytics without needing to hire an entire data team? Let’s chat.
Stitch: Simple, powerful ETL built for developers
Developers shouldn’t have to write ETL scripts. Consolidate your data in minutes. No API maintenance, scripting, cron jobs, or JSON wrangling required.
The internet's most useful data science articles. Curated with ❤️ by Tristan Handy.
If you don't want these updates anymore, please unsubscribe here.
If you were forwarded this newsletter and you like it, you can subscribe here.
Powered by Revue
915 Spring Garden St., Suite 500, Philadelphia, PA 19123