Timnit Gebru. Organizing Data Teams. OpenLineage. Disappearing Data. [DSR #241]
❤️ Want to support this project? Forward this email to three friends!
🚀 Forwarded this from a friend? Sign up to the Data Science Roundup here.
This week's best data science articles
Google's Firing of Timnit Gebru Shows It Can't Stop Being Evil
The firing of data scientist Timnit Gebru demonstrates that companies can’t be trusted to check their own work.
There’s been a lot of ink spilled on Google’s firing of Timnit Gebru over the past week or so by people much closer to the situation than I am, so I won’t attempt to comment on most of it here. Here’s a good place to start if you’re not familiar.
What I like particularly about the linked article is that it points out the structural impossibility of internal “accountability” groups dealing with algorithmic fairness. These groups have about as much credibility, IMO, as the reports published by oil companies stating that their activities don’t lead to anthropogenic climate change. Do you find Exxon a credible source on this topic? Please.
Cathy O'Neill’s point in this article: if you want real accountability, audits have to be done by external auditors. I’d go further: audit standards have to be backed up by regulation. Do we allow companies to audit their own financials? Not a f#%$ing chance. And the third parties that do it are held to standards that actually have teeth.
Anything less is just PR.
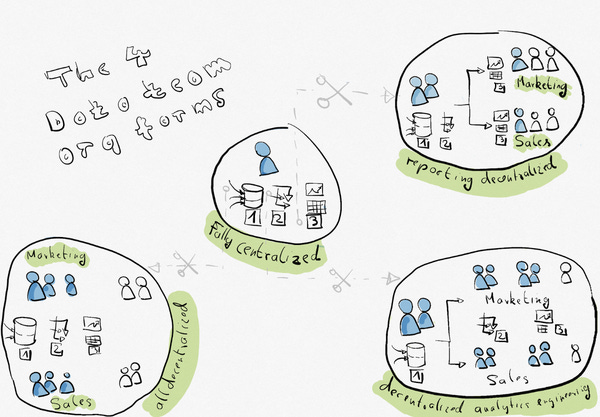
Organizing Data Teams — Where to Make The Cut
There are four ways to decentralize and structure data teams. Learn how to choose the right one.
This is, IMO, one of the most useful articles to come out on the organization of data teams in a while. I had the opportunity to give feedback to the author before it was released; here’s a snippet of what I said:
It’s funny, we often talk about centralized vs decentralized, but we don’t say decentralized what and centralized what. Your article makes it clear that you can leave certain parts centralized and other parts decentralized. Therein lie the interesting questions.
I don’t want to say a lot more here because I’d prefer you just read the post; it’s great. At Fishtown Analytics we’re driving towards the “centralized analytics engineering” model—mostly, but not completely, decentralized.
towardsdatascience.com • Share
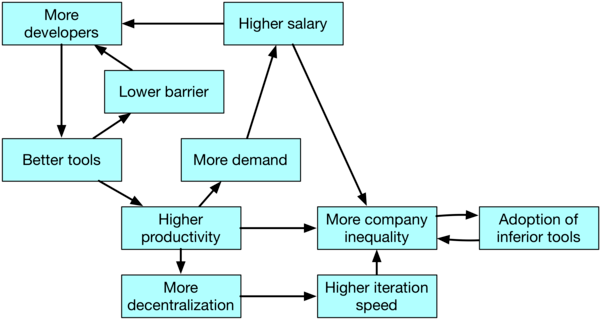
Giving more tools to software engineers: the reorganization of the factory
Wow—I am absolutely blown away by this post. It’s way more zoomed-out than the one above, but still very much focused on the “how do we organize our technical teams” vein. (Remember: analytics is a subfield of software engineering!) If you stick with it the whole way, you’ll get to answers like:
…why the future of the data team is to disappear altogether into cross-functional objective-focused teams.
…why it’s taking a really long time for this to happen.
…why tooling matters a tremendous amount on this journey.
Just…read it.
Metadata is a hard problem for product creators: if your product doesn’t create the dataset, how do you get the metadata you need from it to power your product? This is one reason why metadata products like data catalogs have generally lagged behind the rest of the modern data stack; most of them rely on fairly thin data streams like parsing database query logs. This can go pretty far, but it has some inherent drawbacks too.
The new generation of products, mostly commercial versions of products developed in-house at BigTech, seems unwilling to accept this limitation (rightly so!). The OpenLineage Initiative is an attempt to create a standardized metadata schema such that all tools can publish and consume this standard schema, allowing for a much richer metadata experience across the entire tooling space.
It’s a fantastic vision, and dbt is very much a participant here. Standards like this are also really hard to make sticky, though. I’m cautiously optimistic; it could be a real unlock to the next generation of tooling and data maturity.
When Data Disappears: solving a key quality issue
When we think of data quality, the first issues that come to mind are visible problems like duplicate rows, NULL values or corrupted records. But in fact the most common data quality issue is that data has simply disappeared.
In this post we will describe how data disappears, what the common causes are and what data teams can do to identify these issues.
Most data testing done today attempts to validate the quality of the data that exists. But…how do you test for errors that cause data to disappear altogether?
This post is a bit more product marketing than I generally prefer to link to, but I find its premise quite compelling and it absolutely delivers.
Your data tests failed! Now what?
As excited as data teams might be about implementing data validation in their pipelines - the real challenge (and art!) of data testing is not only how you detect data problems, but also how you respond to them.
Yeah! Operationalizing data testing FTW! I’ve seen very few teams do this well, love that we’re starting to talk about it though…
DeepMind: Using JAX to accelerate our research
Recently, we’ve found that an increasing number of projects are well served by JAX, a machine learning framework developed by Google Research teams. JAX resonates well with our engineering philosophy and has been widely adopted by our research community over the last year. Here we share our experience of working with JAX, outline why we find it useful for our AI research, and give an overview of the ecosystem we are building to support researchers everywhere.
When DeepMind adopts a tool, it’s worth paying attention.
Thanks to our sponsors!
dbt: Your Entire Analytics Engineering Workflow
Analytics engineering is the data transformation work that happens between loading data into your warehouse and analyzing it. dbt allows anyone comfortable with SQL to own that workflow.
The internet's most useful data science articles. Curated with ❤️ by Tristan Handy.
If you don't want these updates anymore, please unsubscribe here.
If you were forwarded this newsletter and you like it, you can subscribe here.
Powered by Revue
915 Spring Garden St., Suite 500, Philadelphia, PA 19123