Why is it hard to be data-driven? ML Contracts. Superset at Scale. Quasi-Experiments. Databricks. [DSR #245]
❤️ Want to support this project? Forward this email to three friends!
🚀 Forwarded this from a friend? Sign up to the Data Science Roundup here.
This week's best data science articles
Why Is It So Hard to Become a Data-Driven Company?
It’s really hard to do data well at large, non-digital-native organizations:
(Fortune 1000) companies reported struggling to make progress — and in many cases even losing ground — on managing data as a business asset, forging a data culture, competing on data and analytics, and using data to drive innovation. Only 29.2% report achieving transformational business outcomes, and just 30% report having developed a well-articulated data strategy. Perhaps most tellingly, just 24% of respondents said that they thought their organization was data-driven this past year, a decline from 37.8% the year before(…)
Heh. This is a headspace I’ve been in for a little while now. The deeper we get into working with enterprises, the more obvious it becomes that doing data well is about so many things that have nothing to do with technology.
Does your existing employee base have raw quantitative reasoning skills? Can they form a question that could be answered with the data?
What are the behavioral norms around decision-making? What do people accept as “good enough” justification to do a thing?
How willing are people to say “I don’t know”? Epistemological uncertainty is a hard thing for most humans to handle!!!
Are executives willing to “kill their darlings” if the data recommends doing so?
And so much more. None of this has to do with “can I stand up modern data technology"—rather, it’s about the ways that data needs to weave itself into the fabric of day-to-day existence at a company in order to actually create change.
Squishy, I know, but real. I’m pretty convinced that changing existing culture is harder than creating new culture from scratch, which is why it’s digital native companies that have most effectively adopted data-driven operating systems. It’s not that they have all of the best data people, it’s that they have broad consensus about how central data is to their very existence.
Machine Learning Models are Missing Contracts
We need to provide contracts that make it clear to users what input data are valid for our models. Otherwise, machine learning models will work properly until they don’t. Systems built on top of machine learning models will fail.
❗❗❗
I love this so much. The whole post is concise and fantastic (maybe even acerbic?). This feels like an important step in the maturity of ML-as-a-practice.
Airbnb: Supercharging Apache Superset
How Airbnb customized Apache Superset for business intelligence at scale.
I haven’t read a post quite like this before! There are so many posts about “how we scaled our streaming data pipeline / Presto instance / etc” but I had never previously read about a company going to massive scale in a single BI tool. I think this is because in practice most companies do not succeed at doing this. While there are certainly very large companies that standardize on single centralized BI tools, one of three things tend to happen:
Company uses a top-down deployment and the BI tool ends up being a way to push high-level metrics out to the org, not to enable IC analysis work. IC analysis work happens in shadow IT.
Company buys Tableau / PowerBI licenses for everyone but there is no centralized experience; the organizing unit is the team / department individual.
Company attempts to deploy BI tool “the right way” but hits limits of scalability and splits the single environment into multiple, causing awkward tectonic rifts.
The picture Airbnb paints here is unique because there are 2,000 knowledge workers all collaborating together inside of a single Superset instance, sharing discoverability, governance, etc. The post really shines a light on the core aspect of Superset that enables this: its Apache 2.0 license. The Airbnb team has meaningfully extended and customized how the product works for them in ways that simply aren’t possible in any proprietary product. I found it very interesting just how critical open source was for them at this layer of the stack.
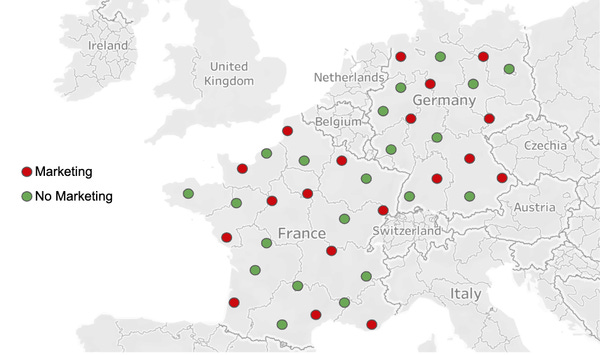
Key Challenges with Quasi Experiments at Netflix
We face various business problems where we cannot run individual level A/B tests but can benefit from quasi experiments. For instance, consider the case where we want to measure the impact of TV or billboard advertising on member engagement. It is impossible for us to have identical treatment and control groups at the member level as we cannot hold back individuals from such forms of advertising.
This practice is one I understand well, but I actually didn’t realize that it had a name! Quasi-experiments. Shopify has written about their approach to quasi-experimentation as well. Read together, these posts present and excellent roadmap for these types of experimental challenges.
Such an important post by the CEO of Fivetran. If you have questions about Delta Lake, the differences between warehouses and lakes, or about generally where data platforms are moving, this short & sweet post has you covered. It’s the clearest independent, no-marketing-bs thing I’ve seen written on this very important topic.
I’m personally extremely excited to see the convergence that George is describing—I ultimately want the operational simplicity and guarantees that the data warehouse provides paired with the computational flexibility of the Spark dataframe. We’re on the cusp of exactly this.
Induction, Inductive Biases, and Infusing Knowledge into Learned Representations
Really fascinating, but if you click through do make sure you’re ready to engage your brain. My favorite part is encapsulated by two quotes:
In his 1980 report The Need for Biases in Learning Generalizations, Tom M. Mitchell argues that inductive biases constitute the heart of generalization and indeed a key basis for learning itself.
A key challenge of machine learning, therefore, is to design systems whose inductive biases align with the structure of the problem at hand.
Essentially: since all ML reasons by induction, removing bias is not a desired goal of the field (induction is essentially the application of learned biases to new contexts). Rather, the goal of ML is to design systems with appropriate (useful / desirable) biases.
While the term “bias” here is being used in a slightly different way than we use it when we talk about “algorithm bias”, I thought it was a really interesting point. What we actually want is not unbiased algorithms, it’s algorithms that are biased in desirable ways.
Thanks to our sponsors!
dbt: Your Entire Analytics Engineering Workflow
Analytics engineering is the data transformation work that happens between loading data into your warehouse and analyzing it. dbt allows anyone comfortable with SQL to own that workflow.
The internet's most useful data science articles. Curated with ❤️ by Tristan Handy.
If you don't want these updates anymore, please unsubscribe here.
If you were forwarded this newsletter and you like it, you can subscribe here.
Powered by Revue
915 Spring Garden St., Suite 500, Philadelphia, PA 19123