I was about to do another 🦃 holiday themed edition of this roundup 🦃, with snippy comments about what Thanksgiving dinner would look like if you invited your data team over instead.
But between how quickly we’re moving through the Greek alphabet with COVID variant names and all of the Very Real Talk happening this week about how little organizations actually value analytics jobs… making jokes today didn’t feel quite right.
Instead, I’m going to lean on Taylor Brownlow’s and Petr Janda’s writing this week as jumping off points to talk about:
why being an analyst today feels more chaotic than ever;
why we systematically devalue analytics and why jobs to be done are more useful to talk about today than job titles; and
why enabling iterative and collaborative data work should be the next modern data tools hill to die on going into 2022 👀🍿.
Today as always I’m looking to continue a conversation rather than to end it, so leave me comments on this post, reply to this e-mail, or jump into the conversation on Twitter. I want to hear what resonated, what didn’t, and why.
Enjoy the issue!
-Anna
Embracing the chaos
Taylor’s What Will “Analyst 2.0” Look Like? has been making the rounds this week in my conversations with surprisingly non-overlapping sets of data colleagues. Turns out, it’s part of a series, and I highly encourage folks to dig into the first post first:
Most of the work we do in data is in an effort to reduce entropy — we model data to remove inaccuracies, we turn commonly asked questions into self-serve reports, and we funnel ad-hoc questions into a formalized request process. This kind of attitude is in our nature as data practitioners and largely serves us well. But in the case of driving decisions with data, we need to challenge our instincts and embrace the chaos.
-Taylor Brownlow Modern Data Stack, It’s Time for Your Closeup
Reducing entropy
is 👏such 👏 a 👏 great 👏 way to describe the reality of data work at any reasonably large company. Data is not a “set it and forget it” kind of activity. Your dashboard will get stale in less than six months. Your key metrics will eventually have bad data in them. That machine learning model you spent all of last quarter developing will drift from its original fit. The environment in which your business operates is constantly changing, and so will the product or service that your business delivers. As a result, what is knowable about your business, about your product or service, is constantly changing too. And fast.
Substantial effort is required to maintain a reliable data experience that enables discovery of what is knowable in an organization with as much accuracy as possible as quickly as possible.
The most valuable way we interact with our data partners today is the messiest — it’s in the slack conversations, the over-the-shoulder demonstrations, or the ad-hoc email with a thrown-together chart with a few bullet points.
This work is both quick and messy […] And that is the crux of the problem. Our business partners are not waiting for us to achieve Gartner’s pinnacle of analytical maturity before making decisions. They’re doing it right now with whatever info they have, and far too often, that does not include data.
-Taylor Brownlow Modern Data Stack, It’s Time for Your Closeup
I buy this.
There’s a lot to be said about bringing data humans into the conversation a lot earlier in the process of making an important business decision. Yet it is also very true that the way we produce recommendations for a business is often very misaligned with the cadence at which decisions are actually made. In other words, data analysis is often too slow.
Petr has a great write-up this week about exactly why this is so slow. Data analysis goes something like this:
I have a problem—an unanswered question. I seek an understanding of the problem on a deeper level, using data.
I get an idea—a clue which I explore. Perhaps a dataset, which can contain some of the answers.
I look through the data—looking for evidence that would confirm or deny my idea. I don’t necessarily do so with the rigour of statisticians, but even a basic analytics approach often shows the way forward.
I iterate—this is very, very important. As soon as I look at the data, I have one answer and two new questions. And so I go on, repeating the previous steps to gain a better and better understanding of the problem iteratively.
If I accept that this is how data analysis should work, it shows how deeply dysfunctional the “pulling data” model is—It assumes that I know what exact data is needed to answer all my questions upfront. But that is simply unrealistic.
-Petr Janda, Bring Data Analyst to the Table
Data analysis is a highly iterative process because the business is always changing, and therefore, so is the underlying data someone is looking at. Even if you’ve answered the same question before, you find yourself checking assumptions — has anything changed since I last looked at this? What was it? Why did it change? Does that have any bearing on my interpretation of this finding?
What is chaos, but the inability to1 trust that you can reliably come back to the same place in your data analysis that you’ve arrived at previously? Taylor, Petr, Benn (and I’m sure others) all agree that navigating this chaos to arrive at a trustworthy recommendation is one of the most important jobs to be done.
So why then, do we systematically devalue this exact work in data analysts?
The value of a job title
One hypothesis is that the analytics role got smaller due to specialization, and as a result it became less valuable:
The data pipeline got chopped up and doled out to new positions like data engineers, or more recently, analytics engineers. Analysts who had once enjoyed large swaths of the pipeline found themselves crowded out on all sides.
-Taylor Brownlow, What will “Analyst 2.0” look like?
I don’t quite buy this. At least, not fully. The jobs to be done in a data team haven't changed substantially. There are 6(ish) jobs to be done 2 that enable a business to make data informed decisions:
👍 Actually deciding on a course of action to take based on available evidence
💡 Recommending a course of action to take based on evidence gathered
📖 Defining what is knowable in an organization
🚰 Delivering a reliable experience that enables discovery of what is knowable in an organization with as much accuracy as possible
🔒 Securing access to what is knowable to only the right set of humans
💅 Building an intuitive, delightful, data informed customer experience 3
What’s changed constantly changing, are the way job titles are mapped to these jobs to be done. The majority of the messiness we are seeing in job titles today is the result of:
Differences in company sizes. Small startups are likely to have one to three people doing all six of these jobs with little consistency in what these humans are called between startups; large companies might have a team specializing on each job to be done.
Iteration our data tools are going through, leading us to do complex combinatorics on these different and adjacent jobs to be done into various hybrid roles because good interfaces between those jobs in our data tooling are currently lacking. I’ll say more on this below.
OK Anna, but data analysts are still getting paid less:
In 2020, data analysts earned less per year of experience than their data science or data engineering counterparts, silencing any analyst’s doubts about what we value in this industry.
-Taylor Brownlow, What will “Analyst 2.0” look like?
Yes. And this is very important.
In some large companies, this gap is institutionalized with analytics pay bands that are one step down from a data science or engineering career ladder. In other words, a senior analyst might get paid the equivalent of a mid career data scientist — a ladder completely divorced from the business impact of a truly exceptional chaos navigator.
That said, I don’t think this problem exists due to a lack of scope (or perceived lack of scope) in the analysts’ role. I think this problem exists due to our persistent inability to value anything other than “technical” skills in the current job market (something this plot of the developer 2020 survey betrays). This is a problem not just in analytics, but it is compounded in analytics by other factors (like the willingness of a business to actually use data to drive decisions).
Iterating collaboratively, and the real reasons analysts aren’t at the table
To diminish the role of the analyst only serves to silence the role of data in any organization. We need a bigger version of the analyst’s role.
-Taylor Brownlow, What will “Analyst 2.0” look like?
But what does it mean to have a bigger version of the analyst role? There are a few takes in recent writing that all come to the same conclusion: we need to bring humans who are exceptional chaos navigators and the decision making process closer together.
Benn Stancil refers to this as the Missing Analytics Executive, Taylor Brownlow calls this the Analyst 2.0, and Petr Janda has this to say:
Next time you encounter an important decision that should be based on data, resist the habit of requesting the next data pull. Instead, go to the analyst with a question and an invitation to the decision-making table. Not just in the boardroom: in every room where important decisions get made.
-Petr Janda, Bring Data Analyst to the Table
Which begs the question — why aren’t analysts in the decision making process today? I think there are actually two reasons, with two very different implications:
🚧 Problem #1: Analysts don’t do the bulk of their work in the same spaces where decisions get made.
🚧 Problem #2: Data stakeholders aren’t actually interested in or incentivized by what the data has to say, and by extension, what the data team has to say.
What problem #2 looks like:
👎 Analysts “age out” into other roles that pay better. Exceptional senior analysts are a rare breed.
👎 Analysts aren’t included in the decision making process early enough leaving them to validate decisions, rather than help make decisions.
👎 Accountability and ethics live in no-man’s land in the organization (to borrow a turn of phrase from Taylor). Analysts often find themselves the lone stewards of these important business processes, with much frustration and little impact.
All of the above are problems with incentives in an organization, and not problems with data tools or the organization of data humans. There’s lots to say here (and I might in a different post), but it is important to recognize that this is a very different organizational situation from problem #1, and simply reorganizing a data team, or changing up data tools alone will not address it.
What problem # 1 looks like:
👎 Using humans as interfaces where there should be systems.
There are legitimate reasons to deploy data humans as interfaces. In “Analyst 2.0”, Taylor highlights a very important one: leveraging specialized backgrounds to act as translators for specific business contexts. That said, we must be careful not to over-rotate on this specialized model because it can lead us down an unproductive path:
First, we deploy data humans as interfaces to decision-making between teams to allow the business to make decisions more quickly (whether formally embedded in teams or loosely mapped to parts of an organization).
Eventually we realize that there are never enough data humans to go around for every feature engineering team and business function. Also, it turns out that to be properly nimble in decision making, we need data humans appropriately staffed and fully ramped up. As soon as someone leaves/moves to another role/gets promoted, we’re left with a big gap.
We therefore find ways to centralize, curate, streamline and formalize those interfaces, but inevitably our tools fall short of supporting this today and we find ourselves back with an embedded model.
Data teams go through cycles like this more than once in their evolution, and will continue to do so until we reach better maturity in the collaborative interfaces we use to help us make decisions.
✅ These interfaces must bring together all 6 data jobs to be done in the same place.
✅ These interfaces must afford the ability to quickly and easily iterate on what is knowable in an organization by humans who are involved in the decision making process — that is, they must be easily accessible to folks who are deciding and especially folks who are recommending an action, ideally in the process of making the decision.
✅ These interfaces must surface appropriate metadata to help deciders and recommenders navigate inevitable data entropy while keeping pace with the needs of the business.
These interfaces don’t exist yet today, but we have the right building blocks within our grasp. I think that these interfaces are necessary ingredients towards a future that Tristan painted last week, one in which the ability to do “analytics” work is as ubiquitous as typing is today.
So all of y’all working on roadmaps and OKRs right now, how’s about we make this a priority in 2022? 😉
Elsewhere on the internet…
I couldn’t quite leave you without some levity today, so here’s a few choice memes to round out this round up:

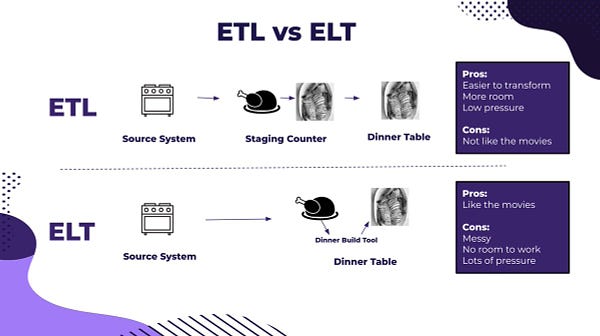
… Basically anything on Seth’s feed in the last 72 hours.
This gem courtesy of Joshua Devlin via the dbt Community Slack
And last but not least, the dbteam made this dbturkey for the festive occasion in the U.S. Enjoy!


Without substantial additional effort
Incidentally, to answer Petr’s question “I am curious about how it feels to be on an exec team with 75% of analytics engineers🤩 “ — this list of 6 jobs to be done is the result of one such exec team conversation 😉
I’ve intentionally left out what I think are corresponding job titles to these jobs to be done. I’m curious to hear from you — how do you organize these jobs to be done on your data team?