Saying true things is hard.
Why our organizational context inherently jeopardizes our role as truth-tellers. And some thoughts on what to do about it.
Julia interviewed me for the final episode of Season 1 of the Analytics Engineering Podcast. I had a lot of fun doing it, and it’s been fun to see initial reactions. I’d be very interested to see if anything in this episode sparks thoughts in your brain.
We’re off next week and then the following week we’ll do a “top 10 links of 2021” issue. Enjoy this last issue of 2021 and I’ll see you in the new year :)
- Tristan
I cannot begin to express how much I love this post by Hubspot analytics engineer Ashley Sherwood. It’s challenging to summarize, because I’ve never read anything _quite_ like it before. I’ll be very literal, then: the entire (fairly lengthy) post is a comparison between the development of a caterpillar (caterpillar » pupa » butterfly) and the development phases of a high-growth business.
The problem with most metaphor-driven posts is that the metaphor only extends so far (ahem1); the beauty of this one is that the metaphor extends so wonderfully well and created such a bounty of new thoughts in my brain. Let’s go on a journey.
Change is the only constant
From the post:
Caterpillars are simple organisms. They are quite literally designed to eat. Everything that a caterpillar does is to this end. (…) As caterpillars grow, their skin can only stretch so far. Inevitably, it splits under the pressure, revealing a new skin layer that does the job until the caterpillar outgrows it too.
This is such a visceral description for the experience of working at a startup—stasis leads to death yet growth creates stretching, breaking. The first person perspective of this process nearly always manifests as discomfort for those of us who go through it. As Buddhism teaches, one of the main causes of human suffering is our attachment to impermanent things, and startups put you face-to-face with this impermanence constantly.
Some of us end up loving this pain, in the same way that somehow humans learned to love capsaicin and skydiving—the experience never gets easier, but instead of being exclusively negative, it somehow induces a state of alive-ness. Here’s my good friend and colleague Nick Erdenberger responding to real-life events just on Friday:
Me too, Nick. Me too.
An Analytics State of Mind
When I’m in flow state, operating at the very top of my license, I’m listening. I’m absorbing inputs from the ether—from Twitter to Feedly to Looker to Hex to Notion—wherever. I’m asking exactly one question on loop: what is the universe trying to tell me? I’ve talked about this before a couple of times, but it actually helps me to anthropomorphize “the universe”…I imagine that there is a signal meant for me to pick up, and I just have to listen closely enough, to follow it with enough courage, to have just enough (but not too much) caffeine to get there. The fictitious construct gets my brain in just the right state.
You could call this process data analysis, you could call it aimless wandering; it’s 100% unstructured, instinct-driven, and it treats all information sources as equally interesting, from long-form text to structured data to Twitter snark. What is the universe trying to tell me?
The music that I most identify with this flow state is hip-hop, and Nas is—nearly 30 years on—still my go-to. I’m listening as I write.
But this flow state isn’t exactly the state of mind that I’m referring to. Because your flow state will look different than mine; you’ll have learned a different practice, a different way of working yourself into this state.
The state of mind I actually mean is one of loose detachment—a third-person academic interest in a subject rather than a first-person investment in an outcome.
This detachment is tremendously hard for data people. Most people at a startup are allowed to be unabashed fans of the product and company; data people are compensated and socialized the same way as everyone else and yet somehow asked to be unbiased, to report the truth. But that’s…hard to achieve in practice.
Imagine:
You’re looking at sales rep ramp for a recent new hire cohort and you realize that three reps are way off schedule. That’s three hard conversations thanks to you.
You realize that CPA for your digital ads for the first half of Black Friday is triple what you had anticipated—auction prices are just too high. However you move forwards, your holiday weekend performance is going to be hosed, and your D2C ecommerce business depends on this weekend to hit its annual goals.
You realize that a new viral loop implemented in your product is really working. It’s still feature-flagged so the numbers aren’t really impacting top line yet, but the 5% of users who are getting the new experience are really responding.
I could write infinity of these scenarios—these make up the lifeblood of your experience as a data professional. The biggest challenge in each scenario is not about data—the analysis itself is straightforward. The challenge is that you are not actually a disinterested observer. You are compromised by your very existence as a self-interested human on the team, with stock options, a track on a career ladder, and human relationships you care about. You are deeply, deeply compromised.
In scenario #1, you’re probably friends with at least one of these three reps and you don’t want them to have to have that talk. No one specifically asked you to look into this, and you do have a lot of other stuff on your plate… It’s easy just to let this just kinda fall on the floor without sharing with anyone. You had other priorities, right?
In scenario #2, this is a hard message to deliver. It’s likely going straight to the CEO and everyone that hears about it is going to immediately get an injection of cortisol—it’s possible that hundreds of millions of dollars of market cap will evaporate because of this analysis. The difference between surfacing this at 11am vs. 3pm might actually be massive but it’s just really hard to break the glass on the fire alarm. You may be thinking about your next job before you hit Send on this one.
Scenario #3 is interesting in a different way—it’s great news, but it’s just as hard to be disinterested in good news as bad. What if, in your excitement about your results, you miss a bug in the instrumentation that invalidates your conclusion? Your analysis causes your team to push the feature to 100% of users, but the good news story never manifests in downstream business metrics. In fact, WAUs actually dip over the coming week. Mistakes happen, but this retro isn’t going to be a lot of fun. You just wanted the feature to be successful!
It’s hard for me to empirically make this case to you, but I can tell you from personal experience that things like this happen really, really often. Even great analysts that I’ve worked with have a very hard time navigating the organizational complexity of attempting to be a truth-teller inside of a commercial organization. It’s not unlike the challenge that most journalists are faced with today—pursue the public interest, but somehow maximize ad impressions at the same time. The two things are in tension with one another.
That is to say…the problem is not about you—it’s inherent to the structure.
In one of my favorite but most frustrating podcast listens ever, I witnessed Danny Kahneman (the academic most closely identified with the study of human cognitive biases) state that he was no better at counteracting his own biases than was anyone else. I was so frustrated upon hearing him say this—if Kahneman can’t get around his own biases, what chance do the rest of us have?
One option is structural—construct a context in which you actually are less invested in the outcome of your analysis.
Back in the early days of Fishtown Analytics I spent 100% of my time thinking about how to create the best possible analytics consulting organization. I intentionally set up our entire engagement model to limit the size of any one of our clients such that we would be ok to lose any of them at any point in time—we were never beholden to any client and so were able to be truly independent. It was a joy to operate in this way—it allowed a pure focus on the work; we could let the data have its say rather than having any personal attachment to a specific result.
This is why companies have have to have external auditors. You can’t trust a company to produce its own financial metrics without having a disinterested external observer validate that things are on the up-and-up.
There are ways to create some level of disinterested-ness for internal teams—the best one is to make sure that analytics doesn’t report into an operating part of the business. If analytics lives inside of product or marketing you’re likely making this problem worse. If analytics reports directly into the CEO, maybe the CFO, you’re likely helping. But still, this isn’t a perfect solution. You can’t escape the organizational pull completely.
The other option, in my personal experience, is cultivating your own personal detachment.
Why Tenure Matters
You’re likely familiar with the concept of “tenure” at institutions of higher education; it’s a hundreds-of-years-old concept where academics who have achieved certain stature have a job for life. The thinking goes: we cannot expect people to spend decades of their lives pursuing new knowledge that will benefit all of humanity if they’re constantly worried where their next paycheck will come from. Tenure is a pretty good attempt to recognize and correct for this structural problem, even if in practice it has created its own issues.
Now—I don’t believe that that we data professionals are about to go full academic and get tenure-track jobs. But the funny thing is that the global labor market is actually providing something akin to that today, it’s just structured a little differently.
I can confidently tell you that if you have worked in the modern data stack for a couple of years, on 1-2 solid teams, and have enough overall data experience to advance beyond the first rung or two of your ladder, you’ll never lack for a job. Your skills are just too in-demand by too many companies and that demand is only growing. Add in the trend towards remote work and you won’t even have to move to take that new job.
This job security will be there for a really long time, maybe even the rest of your career.
Why does this matter? If you have complete confidence that your paycheck is not dependent on the fortunes of and politics within your current company, you can legitimately step into the role of truth-teller.
This is an unusual jump—from the economic freedom of the individual to the efficacy that they have at their job!—and it might feel a little bit uncomfortable. But if you stop and think about it, it is obviously true. The biggest way you are compromised by your organization is their control over your paycheck. Embrace the fact that you’re widely employable and you break that.
This isn’t a global panacea for cognitive biases! You are still a human and you still likely care a lot about your stock option vesting schedule. But it’s something to build from. From there, my process of cultivating academic distance has felt a lot like meditation.
Place your bookmarks.
The more I’ve written here, the more excited I’ve gotten about the topic. I haven’t personally seen a lot of writing about any of this before and am increasingly convicted that this is a blind spot.
The place I want and need to go next is the internal mindset that I’ve cultivated over the course of my career so as to follow my curiosity while quieting my self-interest, and how it feels a bit like mindfulness / meditation when I do it well. How I think it makes me a better strategist, leader, and data professional. I think this is something that a lot of senior data folks do well but don’t know how to talk about.
I also need to wrap back around to the fact that a startup (or in Ashley’s metaphor, a caterpillar) must have a functioning nervous system that tells it the truth about its own internal and external state if it is going to effectively navigate its high-risk series of metamorphoses. That even while the organism is undergoing terrifying change, that nervous system must somehow stay calm and say true things. That was the entire point of this rant, after all :)
But you’ve already followed me all over the place, so I want to pause to get some feedback on this train of thought before continuing.
Do you ever notice yourself being compromised by the structure you operate in?
How have you fought this? Organizationally / personally?
Do you feel like you have “tenure”? If so, when did you feel like this?
Respond to this email or DM me in dbt Slack. I’m jazzed about this and want to explore it more; look forward to hearing your thoughts and going deeper in 2022 :)
From elsewhere on the internet…
Fundraising!
Airbyte seems to have closed a healthy round! Details are scant as this is apparently not 100% final, but apparently the company did confirm it. Ever since Stitch has basically disappeared from the scene I’ve felt an open source gap in the ingestion layer of the stack; I’m excited to see Airbyte showing signs of traction.
Sigma raised a $300m Series C. What’s 🤯 to me about this is how differently their math works out from ours—they only have 220 customers, implying their price points are likely not cheap (I have no insider knowledge on this at all, just reading the press release).
Continual raised $4m seed. I’m very bullish on the approach that this company is taking to get ML more integrated into the MDS. It’s *very* early but worth looking into if you’re not familiar.
Cindi Howson and Thoughtspot on 7 data and analytics trends of 2022 (the trends reports have started!!). It seems that the Thoughtspot team has found religion! Here’s trend #2:
The analytics engineer displaces the data scientist as the world’s sexiest job
So we’ve got that going for us! If you like that takeaway, also see Emilie Schario’s Coalesce talk, Down with Data Science.

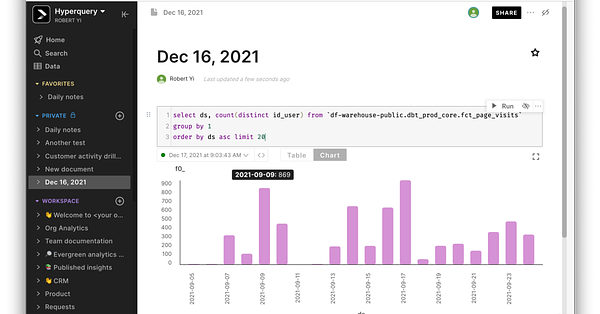
I just got a live demo of Hyperquery yesterday. It’s a very interesting take on data exploration—instead of taking the notebook or the dashboard as its primitive, Hyperquery takes the perspective that the doc is will be the primary experience for exploratory analytics. I don’t have strong priors here, but I do think there are a lot of very interesting new ideas present in Hyperquery that I have never seen. Definitely need to spend some time in it.
Sorry Benn, couldn’t resist! Can’t win ‘em all :P