Top 10 Papers from CVPR. Uber Eats. Data Warehouse Comparisons. [DSR #142]
Hi! I have a quick favor to ask. I’m working with a very high-growth company to hire for multiple positions on their analytics and data science teams. This company is currently at a $500M run rate with a triple-digit growth, and they’re aggressively adding data headcount at all seniority levels. If you or someone you know is currently open to new opportunities, please respond to this email and let’s discuss.
Thanks, and enjoy the issue :)
- Tristan
❤️ Want to support us? Forward this email to three friends!
🚀 Forwarded this from a friend? Sign up to the Data Science Roundup here.
The Week's Most Useful Posts
The 10 Coolest Papers from CVPR 2018
The 2018 Conference on Computer Vision and Pattern Recognition (CVPR) took place last week in Salt Lake City, USA. It’s the world’s top conference in the field of computer vision. This year, CVPR received 3,300 main conference paper submissions and accepted 979. Over 6,500 attended the conference and boy was it epic!
…including one paper entitled “Who Let The Dogs Out? Modeling Dog Behavior From Visual Data”.
My overall thoughts after reading this are that we are definitely at a different place on the technology adoption cycle for computer vision than we were when I started curating the Roundup in 2015. Back then there were new major achievements released seemingly every month. The current papers are meaningful, but are beginning to tackle more detailed, implementation-level questions like “how do I interpolate 60FPS video into 240FPS video?” This is a good shift: it’s an indicator of progress.
Great read to get an understanding of today’s cutting edge.
towardsdatascience.com • Share
Food Discovery with Uber Eats: Building a Query Understanding Engine
Choice is fundamental to the Uber Eats experience. At any given location, there could be thousands of restaurants and even more individual menu items for an eater to choose from. Many factors can influence their choice. For example, the time of day, their cuisine preference, and current mood can all play a role. At Uber Eats, we strive to help eaters find the exact food they want as effortlessly as possible.
Fairly detailed walkthrough of the Uber Eats recommendation system. The highlight of the post is the work the team has done on ontologies. Cuisine has a lot of concepts that map to one another, and in order to build an effective recommender the team has had to build a graph that understands these relationships.
Q: I’ve been reading up on the differences between BigQuery and Redshift. I’m curious how you all would decide which is appropriate for an organization that’s just getting set up with a data warehouse.
With the growing popularity of Bigquery and Snowflake, there’s been a lot of conversation about which data warehouse to choose in dbt Slack. My cofounder, Drew, write a phenomenal post about how you should think about this choice in response to a recent question.
Data science has produced some of the greatest tech innovations in the past decade, but as practiced in many organizations it’s also completely unsustainable. Producing more relevant models, mitigating risk and keeping up with the pace of the field will require organizations to rethink how they do data science.
The author is Principal Data Scientist @ Aetna, and this post contains his thinking on how data science projects should be chosen, run, and funded. Excellent read.
The one thing I’d add is that some organizations already understand how to run speculative projects like this: most R&D spend is high-risk. I think the problem is that many managers and organizations who have little history with making decisions around high-risk projects are now doing so.
towardsdatascience.com • Share
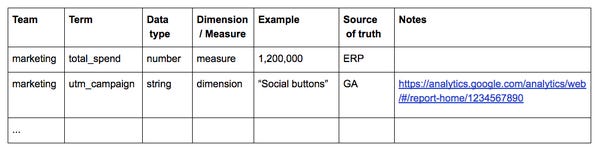
Data Dictionary: How To and Best Practices
A data dictionary is a list of key terms and metrics with definitions, a business glossary. While it is sounds simple, almost trivial, its ability to align the business and remove confusion can be profound. In fact, a data dictionary is possibly one of the most valuable artifacts that a data team can deliver to the business.
What a boring topic, right? Who wants to think about data dictionaries, let’s build models!
The author, Carl Anderson, used to run Data Science at Warby Parker and literally wrote the book on creating an amazing data organization. Sometimes the most useful things are the most basic.
Scalable Deep Learning services are contingent on several constraints. Depending on your target application, you may require low latency, enhanced security or long-term cost effectiveness. Hosting your Deep Learning model on the cloud may not be the best solution in such cases.
Computing on the edge alleviates the above issues, and provides other benefits. Edge here refers to the computation that is performed locally on the consumer’s products. This blog explores the benefits of using edge computing for Deep Learning, and the problems associated with it.
This is by far the best post I’ve seen that breaks down the advantages and disadvantages of deploying deep learning on the edge (close to the user).
towardsdatascience.com • Share
Thanks to our sponsors!
Fishtown Analytics: Analytics Consulting for Startups
At Fishtown Analytics, we work with venture-funded startups to build analytics teams. Whether you’re looking to get analytics off the ground after your Series A or need support scaling, let’s chat.
Stitch: Simple, Powerful ETL Built for Developers
Developers shouldn’t have to write ETL scripts. Consolidate your data in minutes. No API maintenance, scripting, cron jobs, or JSON wrangling required.
The internet's most useful data science articles. Curated with ❤️ by Tristan Handy.
If you don't want these updates anymore, please unsubscribe here.
If you were forwarded this newsletter and you like it, you can subscribe here.
Powered by Revue
915 Spring Garden St., Suite 500, Philadelphia, PA 19123