One of the core philosophies of this newsletter is that by observing the ways that other industries have tried to solve hard problems, we discover lessons that will help drive the data industry forward. Traditionally for this we’ve looked to software engineering - the heart of much of our progress over the past 5 years has been about applying tried and true software engineering principles and best practices to our work in data.
I’m going to try something unusual today. I’m going to draw out some connections I’ve been seeing with an entirely different discipline. The goal here is not to say anything about that particular discipline but rather to share potentially non-obvious lessons I personally took away from my time as a practitioner there.
The discipline in question is politics - namely public opinion polling. A few years back I was lucky enough to be one of the first three team members at Data for Progress. Our job was simple but not easy - to build a public opinion polling system that could reliably tell us, and the political establishment, what the American public was thinking about policy issues like healthcare reform or the fight against climate change.1
It was an entirely different kind of data work - our row counts might have been 1/1000th of what I’d dealt with in my previous work as an analytics engineer at a SaaS company but the complexity and nuance of public opinion made the data problems we faced challenging in completely new ways.
One thing they don’t tell you is that long time political operatives constantly have Silicon Valley types knocking at their door with the next big thing.2 My strategy was different - I didn’t want to be the one with big ideas. My goal was find out what the goals were of the people that knew what they were doing, to execute on them and mostly to learn what they knew that I didn’t. Turns out it was quite a lot.
And then a year ago this month I re-entered the world of tech startups and I started to notice some areas where the hard won lessons from my policy days had some surprising relevance for a lot of the conversations we are having as an industry.
That’s why today we’re talking about the illustrious data practitioner. If you’re a close follower of the discourse, you’ve heard a lot about data practitioners over the last few months.
We need to hear more from data practitioners!
Where have all the data practitioners gone?!
Thing X is what data practitioners actually want!
Slowly and then all at once it dawned on me - these conversations have an eerie resonance with the ways that folks in the policy world talk about voters.
So today I want to share a few of the lessons I learned about the voters and see what they can teach us about the ways we talk about data practitioners.
Data practitioners care about what we’re talking about less than you think
One of the things that most completely and totally breaks your brain when you work in politics is the fact that nearly everything you think is a massive, monumental story gets a yawn and a blink from the voters.
About once a week a story would be break and I would just absolutely know that this changes everything. We’d put out our poll and I would sit there, refreshing the page and awaiting my sweet vindication.
And then the numbers would come in and they would be the same as they were last week and the same as they were the week before that.
My intuition on what was going to matter to the voters had been fried by years of poring over political twitter. In order to understand the voters I had to be able to see the situation with the eyes of people who were not as invested in it as I was.
If you’re a regular reader of this newsletter, either as a political operative vendor or as an activist engaged practitioner there is one thing that axiomatically sets you apart from the average data practitioner - you think about this industry more (and probably much, much more) than they do. This is great! We spend our time reading about industry dynamics, connecting with others, writing epic substacks and crafting memes because we care about this industry and believe in what it can be.3
But it does make it challenging, sometimes, for us to empathize with the median voter data practitioner. While we’re swept up in the 19th iteration of the is self serve viable
or what an insight
is - we need to remember these aren’t the things practitioners are thinking about day to day.
There are two main reasons it’s important to recognize this.
We can’t Deliver Value without knowing what people actually care about and there are broad swathes of
votersdata practitioners that aren’t involved in many of the conversation we have. This is why the DX team at dbt Labs has heard me say enough times that they’re quite sick of it that we need to “focus on the kitchen tables issues that matter to your hardworking, everyday analytics engineer”. Things like how to upgrade dbt versions without losing your mind and how to structure your projects.It can be incredibly difficult if you are someone who is newer to The Discourse to get involved. This is really bad! We need to create an environment where if this is your first month or third decade working in data, you feel like the broader data community is here for you. When we get bogged down in the long running and sometimes insular meta-narratives it makes it much harder for folks to get involved. This is top of mind as it was recently raised by an awesome newer member of the data community.

The best way to combat an insular mindset is to make contact with reality.
In the political world, we had a huge number of ways to do this, each imperfect but each giving a perspective. Polling was one, but there is also focus groups, canvassing and rallies to name a few. For us, it’s more like meetups, conferences and directly talking to other members of the community.
This is one reason why I love talking to folks in our revenue and professional services organization - their day to day is nothing but making contact with reality and understanding what’s actually going on in data orgs. They don’t have the luxury of getting caught up in sweeping narratives.
And we must combat the instinct to grasp at overarching stories, because the first step after realizing that you don’t know quite as much about a group of people as maybe you thought you did is often to engage in cliche.
Data Practitioners are more heterogenous (aka weirder) than you think
Raise your hand if you remember a lot of profile pieces of Trump voters sitting in Ohio diners after the 2016 election season. After realizing that the existing model of the voters was wrong, the first thing that happened was a search for an easy caricature of what “the average person” is like.
This is what a lot of writing about data practitioners looks like today.
It’s Tuesday morning. Your CEO reads a dashboard and see’s a number that “just doesn’t look right”. You get the dreaded email fw: Daily Revenue Tracking that says “can you pull a number / doublecheck this real quick”.
The above is a real thing that happens - quite a lot! But, much like the real voter that exists at a diner in Ohio, if this is the only story we tell we are erasing a tremendous amount of nuance and detail from the world.
The second truth from the policy world I want to bring up is that it is borderline impossible to constrain the aggregate day to day experiences of a wide group of people into a single overarching narrative. Such stories can be useful, but they are always incomplete. An inevitable truth we learned to accept is that people have extremely weird views on issues.
The average views might boil down to looking fairly standard, but if you zoom in there is almost unimaginable variation. One of our favorite hobbies was following American Voter Bot - a twitter account that would present you with the policy opinions and voting behavior of individual voters. The behaviors you find there are outside (often far outside) the bounds of views we expect people to hold. Our stories did not match the complexity of reality. A meme that has emerged to help understand this is the idea of the chad centrist.
4
So what do data practitioners actually care about?
It might be something that aligns to our metanarrative of the day. Maybe it’s getting pinged to just pull a number real quick. Maybe it’s the difficulty getting a stakeholder to define a problem.
Probably, something extremely weird.
I’m reminded of the two months, early in my data career where what I cared about, more than anything, was tracking down the ghost account that was mysteriously sucking up all of our revenue.
Every day I would log into our Kissmetrics (iykyk) dashboard to find that several of our paying customers total revenue had zeroed out and the revenue had been assigned to a random new account. Then, the next day all of that revenue would mysteriously move to a new and different account.
For that two months, my number one priority, far above anything else, was simple: to find and destroy the ghost account that was haunting my system.
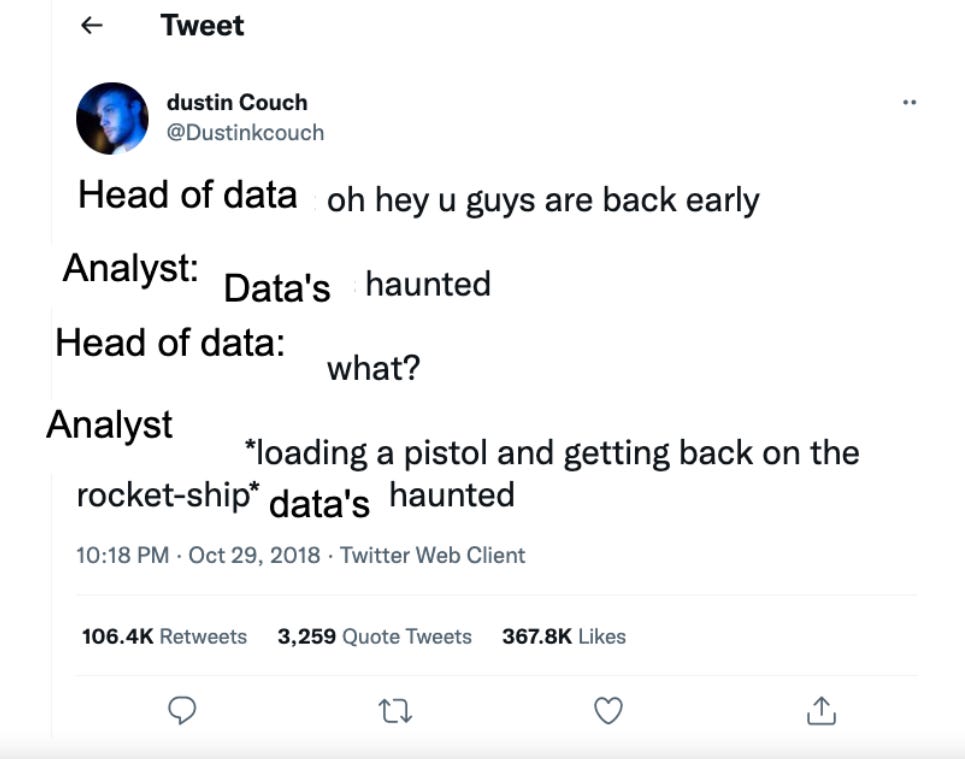
We’ve got to be constantly interrogating our mental models to make sure we’re actually modelling reality, not a meta-narrative we’ve latched on to. We’ve got to be sharing what’s actually going on in our data orgs and sharing that information across the community.
Data practitioners, by and large, are dealing with weird stuff.
The difference between the overton window and the overton door
As are we all. The final parallel I want to draw is less about the day to day work we do and more about how to stay in it for the long haul.
One of the strangest learnings from the political world is how much more painful a lot of the work becomes once you actually win an election and begin to wield power. When out of power you spend your days strategizing, dreaming and crafting the brilliant plans you’ll execute when ascend. Then all at once the election is over and the one that needs to fix things is now you. You look around at your coalition and discover you have to do the hard work of passing bills.
The concept that’s important here is “the overton window” - or the set of ideas that are possible to consider as political reality. While you’re out of power, you spend much of your time determining what the overton window will be for your next administration. You become furiously, fanatically focused on what will be possible in the future.
And then you look to your left, look to your right and realize that your job now is to make those some things possible in the present.
As one of my friends memorably put it to me, “sometimes after you’ve focused on moving the overton window you need to walk through the overton door”. The strategies that are successful for shifting the overton window are very different than the ones that help you walk through the overton door.
We, as an industry, are in the fortunate position of having dramatically shifted the overton window. We have a spotlight. We have funding. We have an opportunity to do things. We’re starting to find out how daunting and how thrilling that can be.
The final hands on lesson I learned from my time in the policy world is that opportunities to change things are fragile and they are rare. Even though you anticipate their approach for years, they can take you by surprise when they actually approach. Holding onto hope can be a challenge.
The process from realizing that the overton window needs to move to moving the overton window to walking through the overton door can, as Benn has reminded us again and again, be brutal. But sometimes, after all the dreaming and scheming and agitating and doubting and second guessing, we reach a moment of pristine clarity. It’s a truth we should hold onto when it comes, as it must sustain us in the long stretches when progress stalls or victory seems elusive. It’s always worth it to try.
Elsewhere on the Internet…
Katie Bauer on the Analytics Engineering Podcast
Look I’m going to let you in on a little secret here - my podcast consumption has been lagging a bit over the past few months and I’ve been a bit behind catching up with episodes of the podcast that accompanies this newsletter. This episode is a must listen.
If you’re a regular on twitter, you know that Katie Bauer shares wisdom on data teams that can instantly reframe how you think about problems. Quite simply she does not miss.
It’s almost a little unfair that she is, if anything, better in a long form interview. I was stopping this episode every three to five minutes to take notes on things I wanted to remember or put into practice. I’ll share one quote here and leave the rest for you to discover yourself.
So I work with an infrastructure organization and it means I end up thinking about how infrastructure gets created a lot. And something that was once described to me is the infrastructure process that everyone agrees on.
Testing for Table-Specific Data Issues
By Madison Mae
Speaking of practitioners, we have an awesome new post from one of the best practitioner-written resources currently going - Madison Mae’s Learn Analytics Engineering newsletter. Not only does Madison provide great hands on advice on how to improve your analytics skillset - she grounds it in the real problems she’s currently working on as a data practitioner. From the article:
A few weeks ago I embarked on the project of documenting all the tables within a database and mapping their relationship to one another. Documenting the tables used all the time was the easy part. The hard part was getting to the purpose of the tables I never even knew existed.
The data issues in table you use all the time eventually lose their power over you and become like the particular idiosyncrasies of old friends. On the other hand, there is a very specific feeling of dread that goes along with inheriting a set of tables that contain mysterious data problems unknown to you. Madison walks us through how she approached one such project.
Data Mesh — A Data Movement and Processing Platform @ Netflix
By Bo Lei, Guilherme Pires, James Shao, Kasturi Chatterjee, Sujay Jain, Vlad Sydorenko
Well this article was certainly very different than what I was expecting from the title but fascinating nonetheless! I always appreciate it when large orgs give us a peek behind the screen at how they are handling their data - just an entirely different world of problems than faced at other organizations.
A great article if you, like me, are a fan of data architecture diagrams - there’s just something oddly soothing about them.
The fascinating thing about this article is that it’s not about pointing out any shortcomings in Airflow - it’s highlighting the truly incredible difference in scope, scale and complexity of the problems that data teams are solving compared to just a few years ago. The Cambrian Explosion is here, it’s provided us with a great new set of tools and it can make our lives extremely chaotic.
Stephen always manages to make in-depth technical concepts fun to read and understand and this post is no exception.
Airflow was never intended to be a heterogeneous platform intended for decentralized DAGs. It is a job scheduling and processing engine: take a single team’s workload and orchestrates it on a schedule, akin to a subway system.
The job of today’s data engineers is more akin to managing the entire transportation network — subways, sure, but also streets, buses, bike lanes. When the growth team drops 1000 scooters on the streets overnight, data engineers have to ensure they don’t cause accidents or get people killed. That is the new job.
Could quote more or less the entire article, but instead just suggest going over and reading the whole thing from top to bottom.
That’s all for this week folks! As always, thank you for reading.
Curious how we did? Explore the record here: https://projects.fivethirtyeight.com/pollster-ratings/data-for-progress/
Pro-tip: don’t say you’re trying to “Growth Hack Democracy”
And the conversations we have shape the industry. Our layer of the conversation is where industry trends are formed, where companies are founded and first-funded. Where conference talks are conceptualized and potentially transformative new open source projects are given a chance to shine.
I believe I was first introduced to this meme via the twitter of brilliant scholar Jake Grumbach
Great post! I can't be the only person who wants to know the final outcome of what was going wrong w/ your ghost account...